Fixed Budget Kernel LMS based Estimator using Random Fourier Features
2020 IEEE Radar Conference (RadarConf20)(2020)
摘要
Accurate estimation of delay and Doppler shift are essential for target detection and tracking in a radar system. In this regard, online reproducing kernel Hilbert space (RKHS) based estimation techniques have emerged as viable for radar systems, due to guarantees of universal representation, and convergence to low estimator variance. However, existing RKHS based estimation techniques for radar rely on growing dictionary of observations, which makes it difficult to predict the memory requirement beforehand. Furthermore, online dictionary based learning techniques are prone to erroneous observations in the high-noise regime. In this work, a finite implementation-budget estimator is proposed, which utilizes an explicit mapping to RKHS using random Fourier features (RFF). The proposed RFF based estimator achieves equivalent/better performance as compared to its dictionary-based counterpart and has a finite memory requirement that makes the estimator attractive for practical implementation. Simulations are performed over realistic radar scenarios, that suggest the viability of the proposed RFF based estimator.
更多查看译文
关键词
KAF,KLMS,RKHS,RFF-KLMS,Euclidean space
AI 理解论文
溯源树
样例
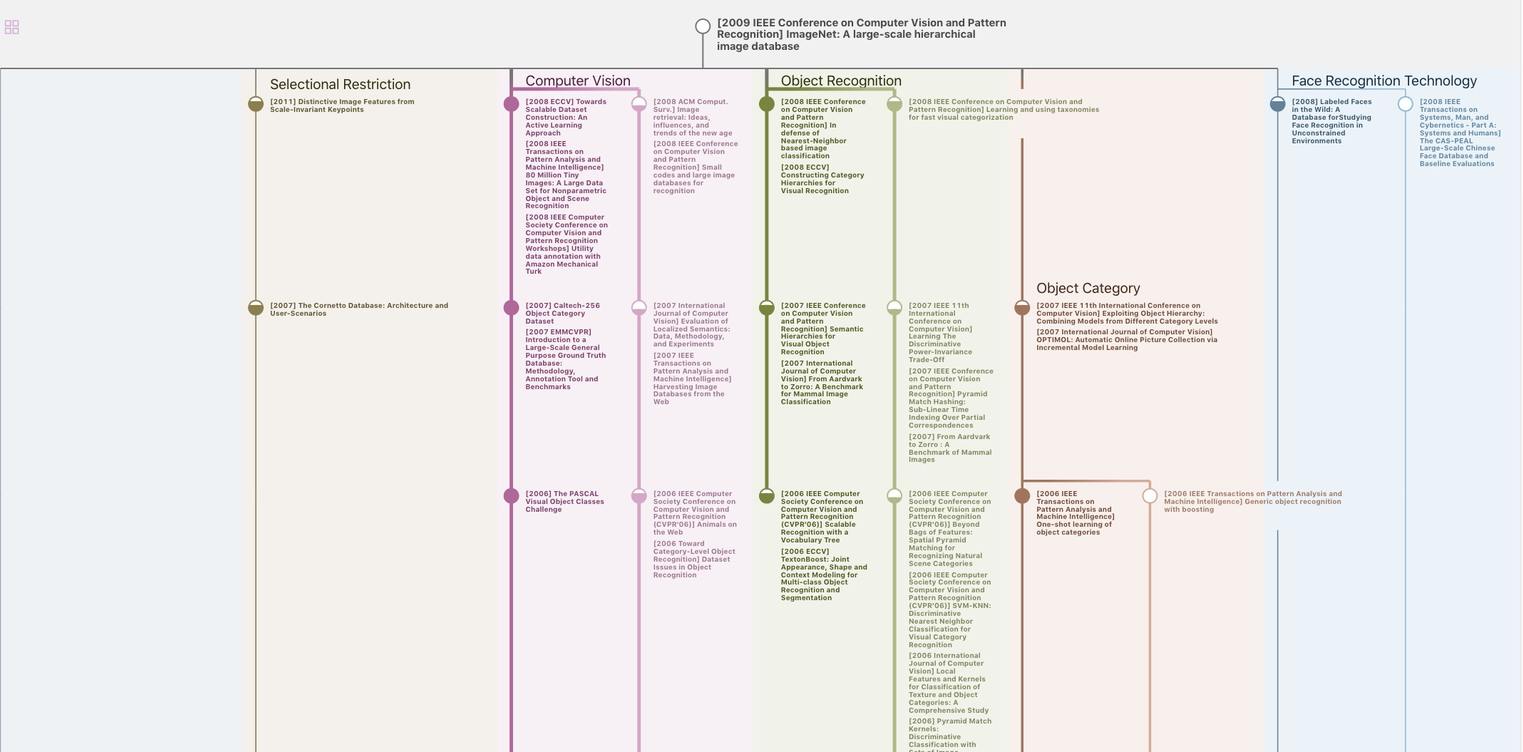
生成溯源树,研究论文发展脉络
Chat Paper
正在生成论文摘要