Two Graph-Regularized Fuzzy Subspace Clustering Methods
APPLIED SOFT COMPUTING(2021)
摘要
This paper presents a new fuzzy subspace clustering (FSC) method which finds some subspaces as clusters such that each point belongs to the nearest subspace with a certain weight or probability. Then we propose two graph-regularized versions for it, in which two points are more likely to be assigned to the same cluster if they are close spatially or with the same labels. In the proposed two graph regularizations, one encodes the weight (or probability) of a point assigned to a cluster and the other encodes the projection coefficients of a point on a subspace. We develop iterative solutions for these methods through constructing a surrogate, which monotonically decrease the cost function with a simple structure. The experimental results, using both synthetic and real-world databases, demonstrate the effectiveness and flexibility of the proposed methods.(c) 2020 Elsevier B.V. All rights reserved.
更多查看译文
关键词
Fuzzy c-means (FCM), Fuzzy subspace clustering (FSC), Gaussian mixture model (GMM), Graph regularization, Surrogate
AI 理解论文
溯源树
样例
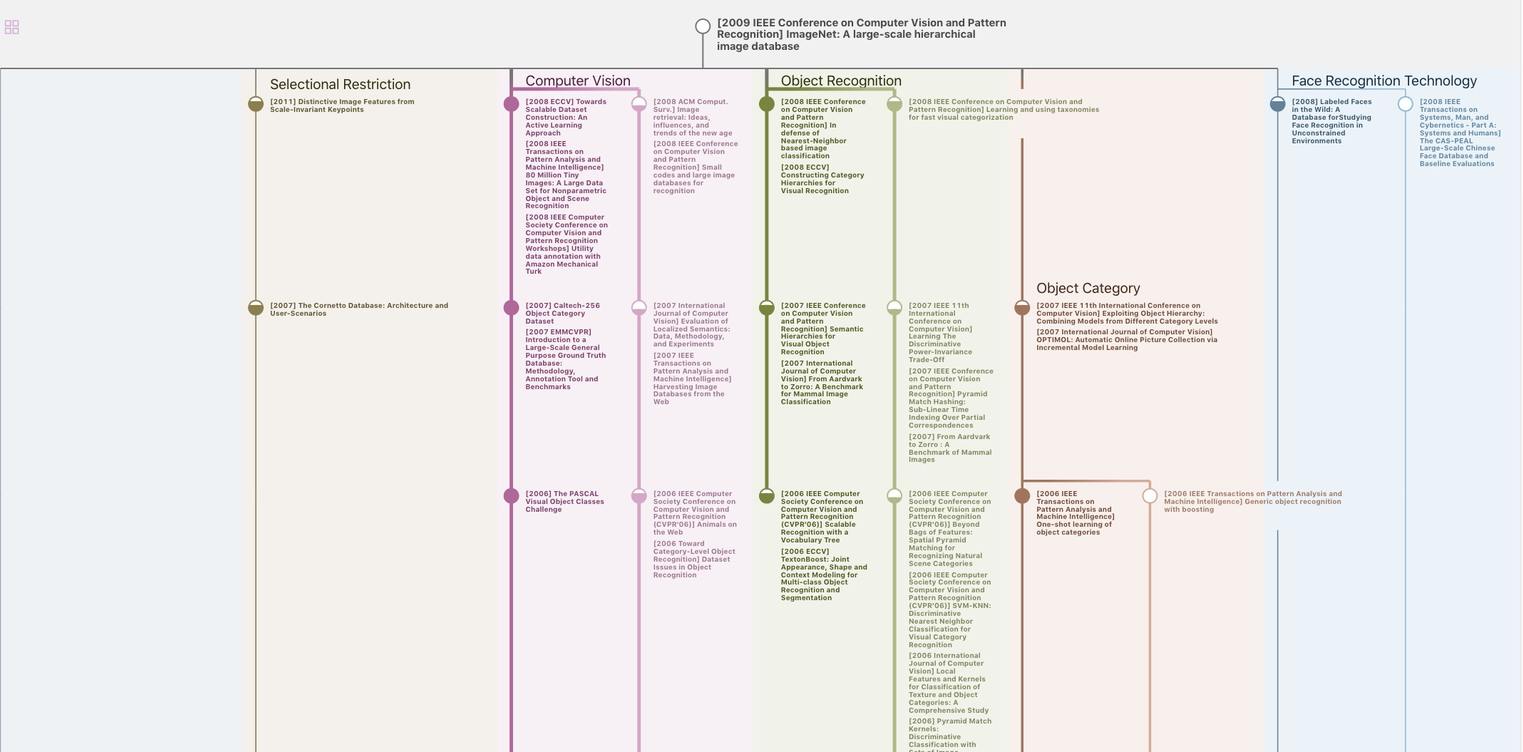
生成溯源树,研究论文发展脉络
Chat Paper
正在生成论文摘要