Cnn-Lstm Based Approach For Parameter Estimation Of K-Clutter Plus Noise
2020 IEEE RADAR CONFERENCE (RADARCONF20)(2020)
摘要
This paper concerns the problem of estimating the parameters of the K plus noise distribution. In a previous work, it has been shown that, in the multilook scenario, the modified fractional order moment estimator (MFOME) has about the same estimation accuracy as the [zlog(z)] method, but lower computational complexity. However, significant estimation errors have been observed in the single look scenario, low sample size, and large values of the K-distribution shape parameter. Moreover, the computational complexity of the [zlog(z)] estimator discourages its implementation in practical applications. The aim of this work is to estimate the shape parameter of the K-distribution with reduced computational complexity. The problem can be formulated as a supervised many-to-one sequence prediction. We propose here a hybrid model including convolutional and long-short-term-memory (LSTM) neural networks (NN). Estimation performance is investigated by processing both simulated and real clutter data.
更多查看译文
关键词
K-Clutter, Thermal Noise, CNN, LSTM, Parameter Estimation, Deep Learning
AI 理解论文
溯源树
样例
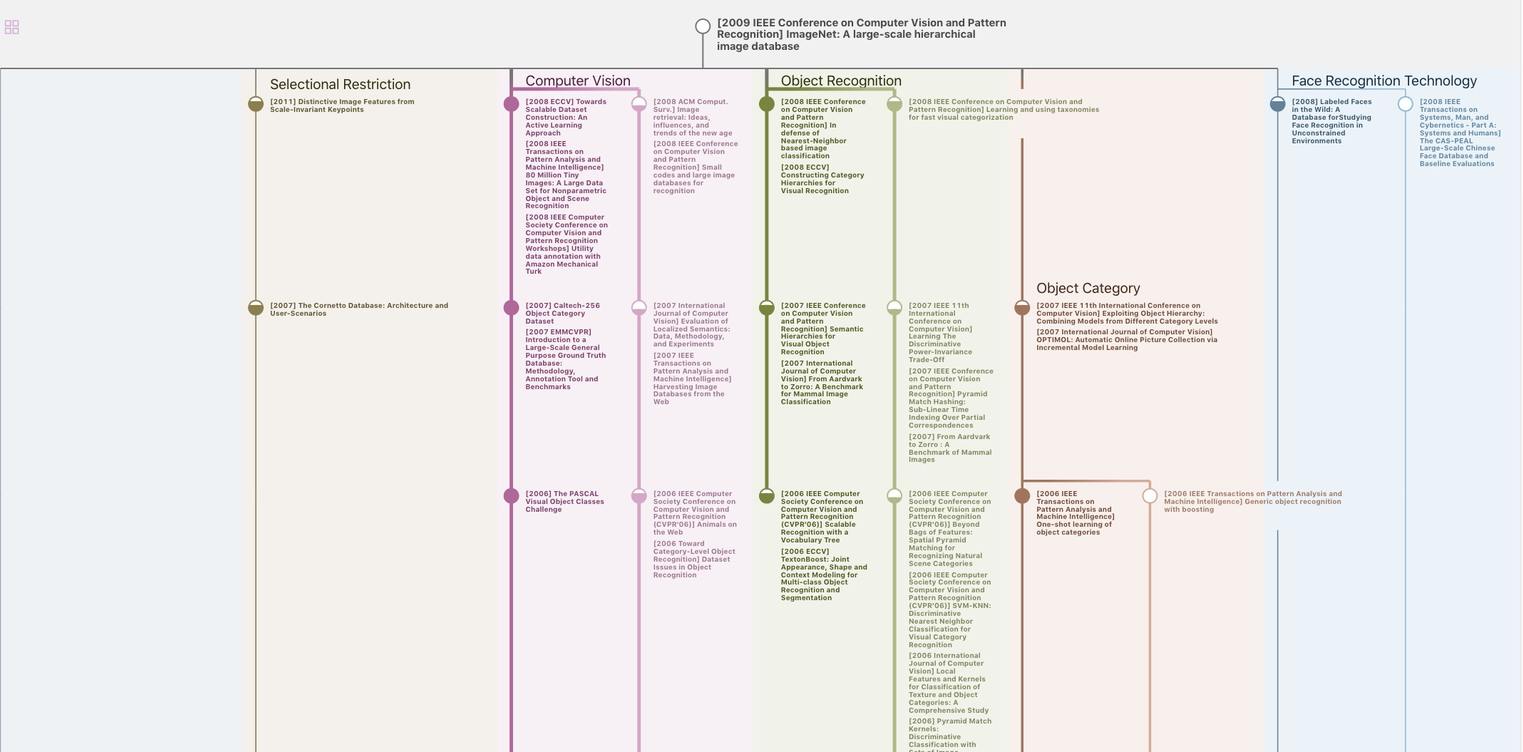
生成溯源树,研究论文发展脉络
Chat Paper
正在生成论文摘要