Fetal Brain Segmentation Using Convolutional Neural Networks with Fusion Strategies.
international symposium on visual computing(2020)
摘要
Most of the Convolutional Neural Network (CNN) architectures are based on a single prediction map when optimising the loss function. This may lead to the following consequences; firstly, the model may not be optimised, and secondly the model may be prone to noise hence more sensitive to false positives/negatives, both resulting in poorer results. In this paper, we propose four fusion strategies to promote ensemble learning within a network architecture by combining its main prediction map with its side outputs. The architectures combine multi-source, multi-scale and multi-level local and global information collectively together with spatial information. To evaluate the performance of the proposed fusion strategies, we integrated each of them into three baseline architectures namely the classical U-Net, attention U-Net and recurrent residual U-net. Subsequently, we evaluate each model by conducting two experiments; firstly, we train all models on 200 normal fetal brain cases and test them on 74 abnormal cases, and secondly we train and test all models on 200 normal cases using a 4-fold cross validation strategy. Experimental results show that all fusion strategies consistently improve the performance of the baseline models and outperformed existing methods in the literature.
更多查看译文
关键词
convolutional neural networks,segmentation,fusion,brain
AI 理解论文
溯源树
样例
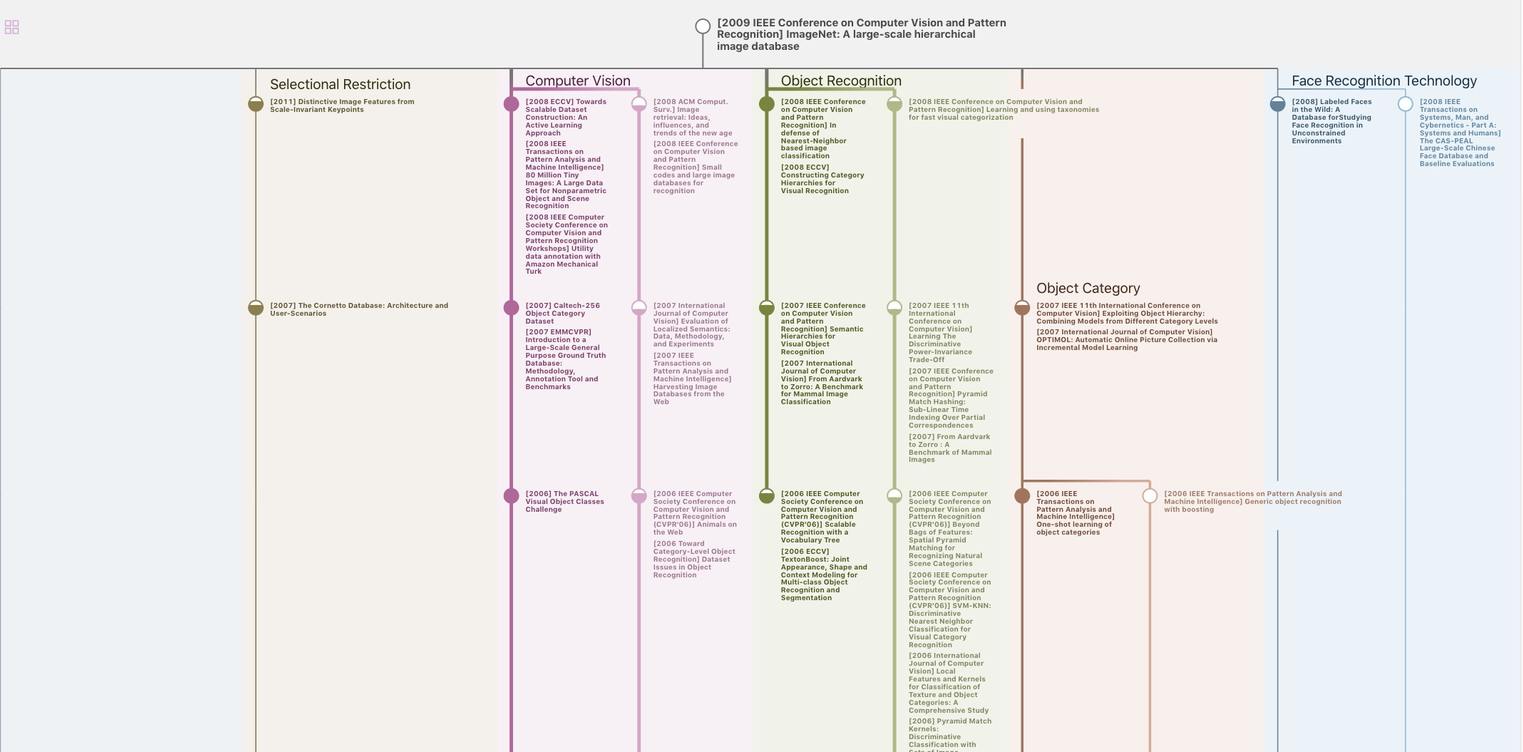
生成溯源树,研究论文发展脉络
Chat Paper
正在生成论文摘要