On Learning Based Compressed Sensing For High Resolution Image Reconstruction
IET IMAGE PROCESSING(2021)
摘要
Compressed sensing (CS) or compressive sampling has shown an enormous potential to reconstruct a signal from its highly under-sampled observations. A high dimensional image processing system can adopt the CS paradigm to reduce the storage and the transmission burden. However, a large sensing system (matrix) is required to capture high dimensional images. The CS reconstruction algorithms demand heavy computational requirement due to the use of large sensing matrix. It sometimes becomes impractical to implement a sensing system of the desired size due to the limited access to the CPU memory. To address this issue, the present work proposes a deep learning based CS framework that uses a convolutional neural network to enable capturing of a high dimensional image by utilizing a multi-layer subsampling and filtering operations. The proposed approach uses another convolutional neural network that reconstructs the original image without depending on the sensing network at a significantly reduced computational cost. Extensive simulation results show that the proposed method reconstructs a high dimensional image with an improved PSNR value by 1.5 +/- 0.63 dB, SSIM value by 0.04 +/- 0.02 and FSIM value by 0.02 +/- 0.02 compared to the other state-of-the-art methods in less than 1.4 seconds.
更多查看译文
关键词
Image and video coding,Computer vision and image processing techniques,Neural nets
AI 理解论文
溯源树
样例
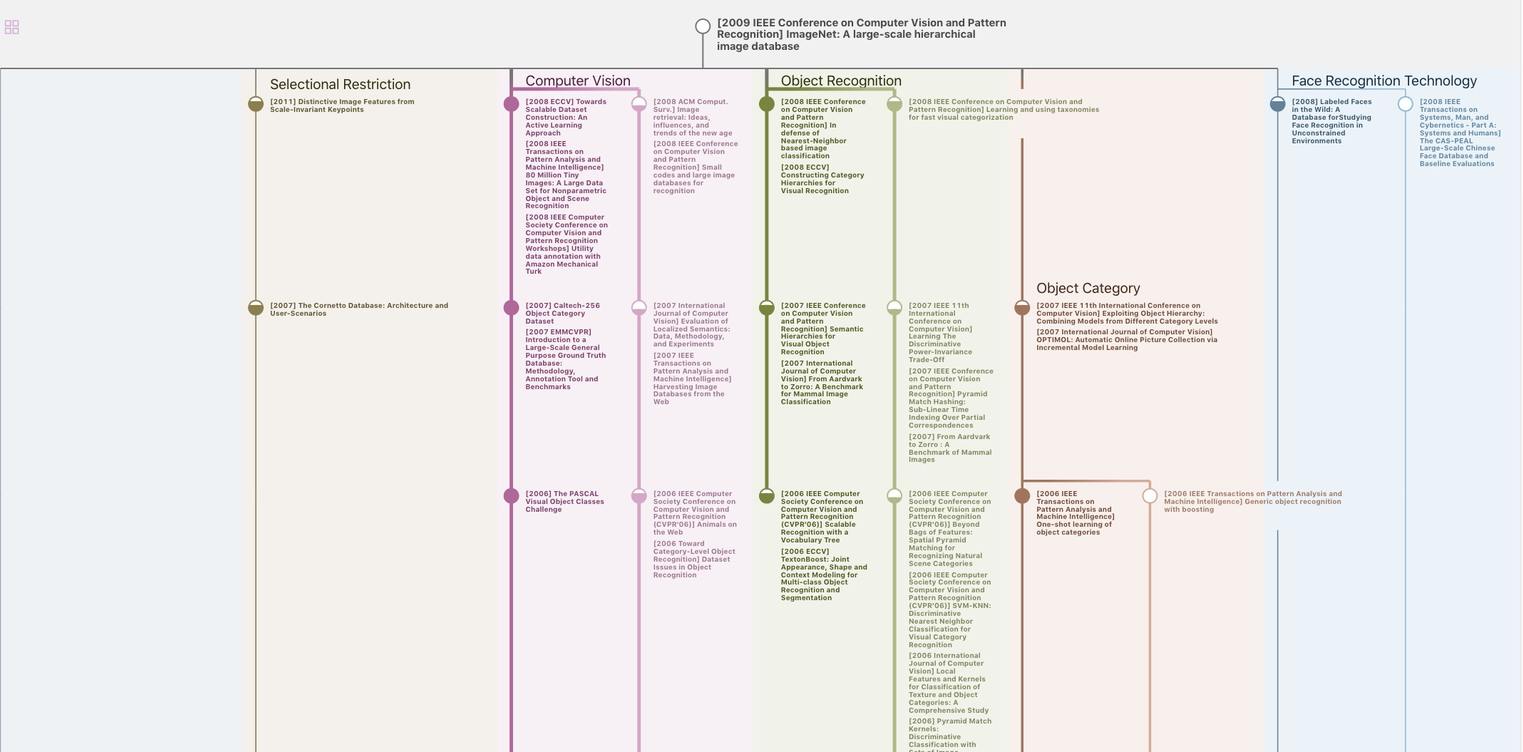
生成溯源树,研究论文发展脉络
Chat Paper
正在生成论文摘要