Obtaining Underlying Parameters From Magnetic Domain Patterns With Machine Learning
JOURNAL OF THE PHYSICAL SOCIETY OF JAPAN(2021)
摘要
The question of how to understand the underlying mechanisms of complicated spatiotemporal patterns formed in various physical, chemical, and biological systems is still and open issue. Here, we give a successful example using the machine learning methods of a convolutional neural network (CNN) and a kernel ridge regression (KRR). By using these methods, we could quantitively identify an underlying parameter, the anisotropy coefficient a, from images of magnetic domain patterns formed in ferromagnetic thin films. We demonstrated that models based on both machine learning methods successfully identify a, with a mean absolute error of less than 0.077, from data synthesized with the time-dependent Ginzburg-Landau (TDGL) equation. Further, we showed that the CNN model outperformed the KRR model, but the KRR model provided significant pattern features for representing a more interpretably than the CNN could. Our results provide new insights into pattern analysis of magnetic materials from a machine learning viewpoint.
更多查看译文
关键词
Backpropagation Learning
AI 理解论文
溯源树
样例
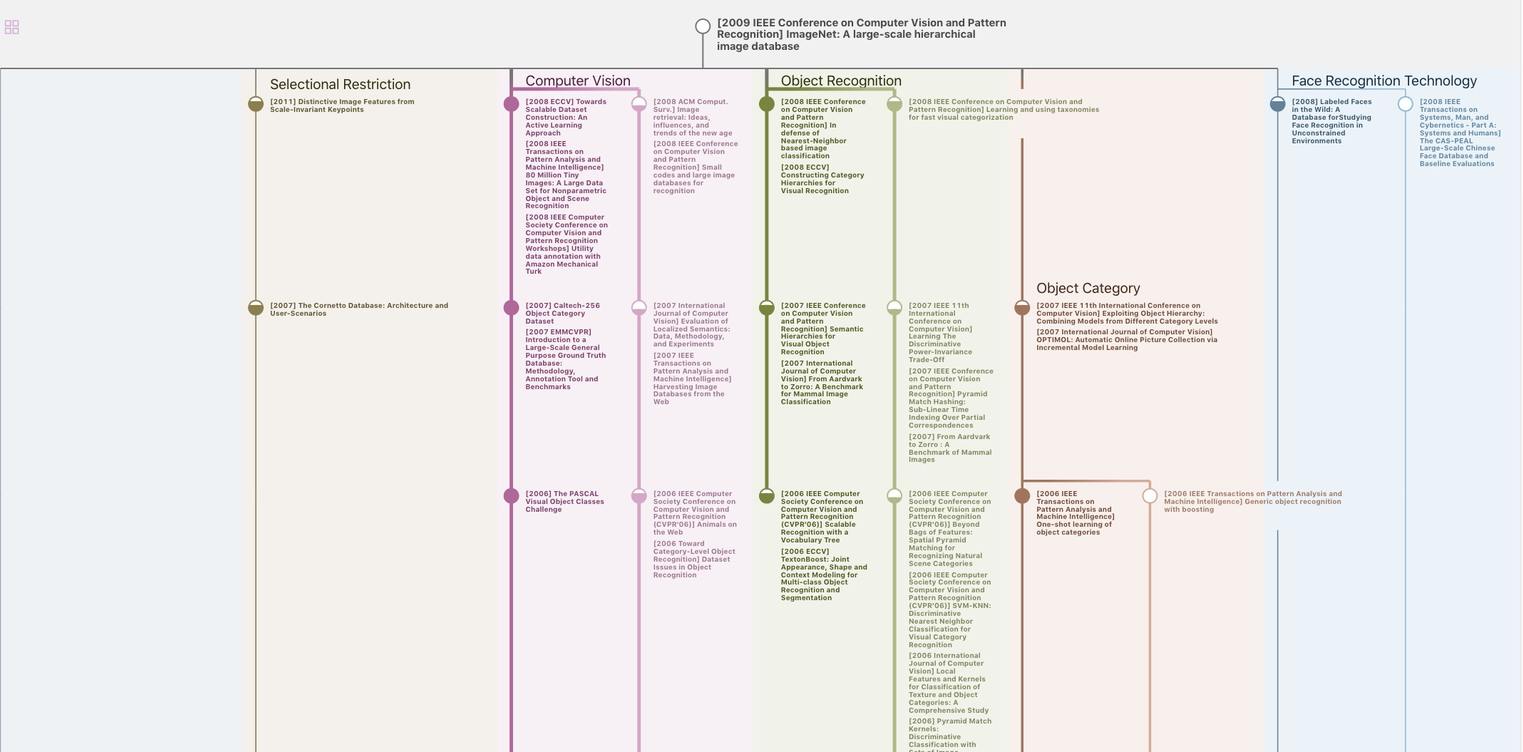
生成溯源树,研究论文发展脉络
Chat Paper
正在生成论文摘要