A Hybrid Model Based On Multi-Stage Principal Component Extraction, Gru Network And Kelm For Multi-Step Short-Term Wind Speed Forecasting
IEEE ACCESS(2020)
摘要
Accurate wind speed forecasting exerts a critical role in energy conversion and management of wind power. In term of this purpose, a hybrid model based on multi-stage principal component extraction, kernel extreme learning machine (KELM) and gated recurrent unit (GRU) network is developed in this paper, where the multi-stage principal component extraction combines complete ensemble empirical mode decomposition with adaptive noise (CEEMDAN), singular spectrum analysis (SSA) and phase space reconstruction (PSR). Firstly, CEEMDAN is employed to decompose the raw wind speed data into a sequence of intrinsic mode functions (IMFs) and a residual component. Then the principal components and residual components of all IMFs are captured by SSA. Meanwhile, all residual components obtained by CEEMDAN decomposition and SSA processing are added to form a new component. Subsequently, PSR is utilized to construct each forecasting component obtained by CEEMDAN-SSA into the input and output of training set and testing set for the prediction model. Later, KELM and GRU neural network are conducted to predict the high-frequency and low-frequency components, respectively. Eventually, the prediction values of each component are accumulated to acquire the final prediction result. To evaluate the performance of the proposed model, four datasets from Sotavento Galicia wind farm are adopted to conduct experimental research. The experimental results manifest that the proposed model achieves higher accuracy of multi-step prediction than other comparative models.
更多查看译文
关键词
Multi-step short-term wind speed prediction,multi-stage principal component extraction,complete ensemble empirical mode decomposition with adaptive noise,singular spectrum analysis,phase space reconstruction,kernel extreme learning machine,gated recurrent unit network
AI 理解论文
溯源树
样例
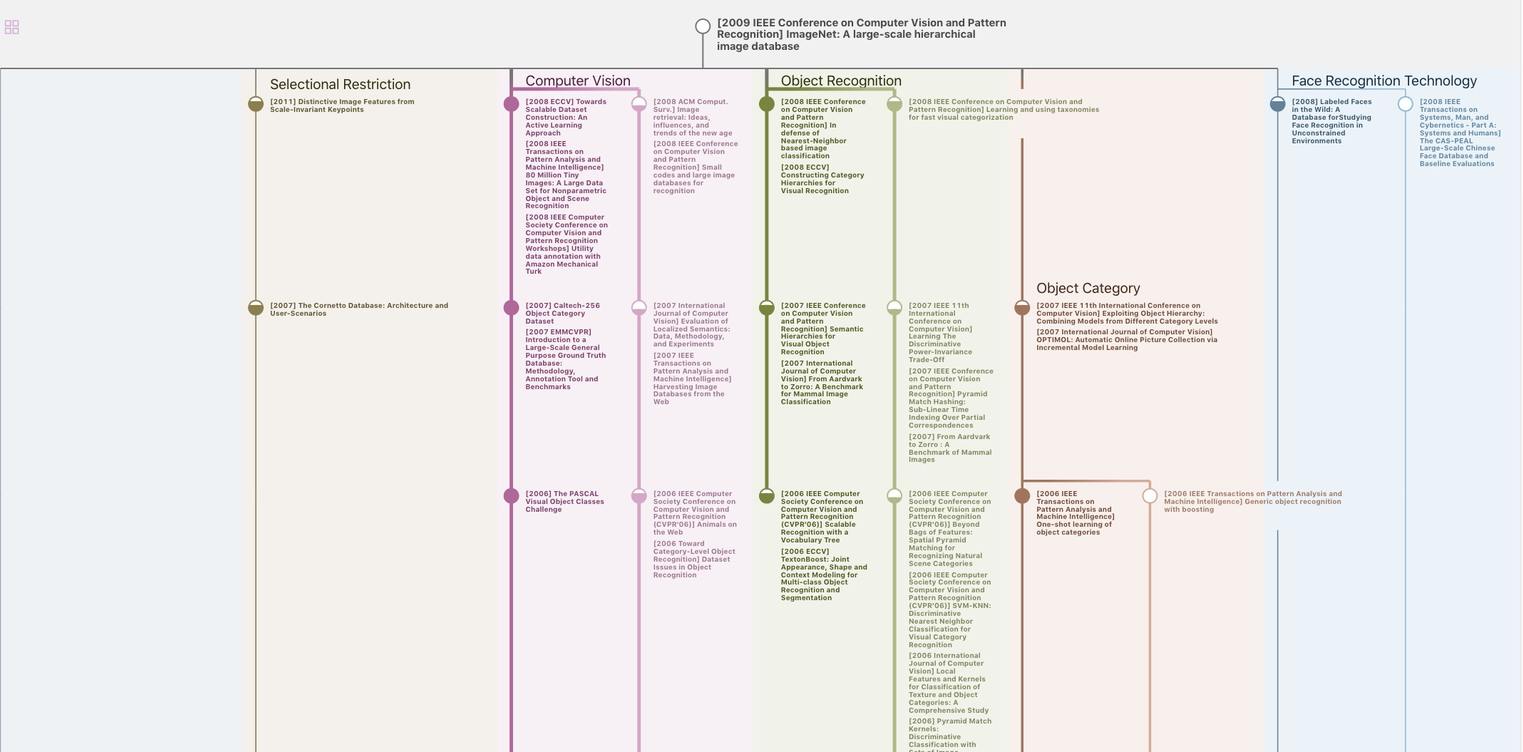
生成溯源树,研究论文发展脉络
Chat Paper
正在生成论文摘要