End-To-End Quantum Machine Learning Implemented With Controlled Quantum Dynamics
PHYSICAL REVIEW APPLIED(2020)
摘要
To achieve quantum machine learning on imperfect noisy intermediate-scale quantum (NISQ) processors, the entire physical implementation should include as few as possible hand-designed modules with only a few ad hoc parameters to be determined. This work presents such a hardware-friendly end-to-end quantum-machine-learning scheme that can be implemented with imperfect NISQ processors. The proposal transforms the machine-learning task to the optimization of controlled quantum dynamics, in which the learning model is parameterized by experimentally tunable control variables. Our design also enables automated feature selection by encoding the raw input to quantum states through agent control variables. Comparing with the gate-based parameterized quantum circuits, the proposed end-to-end quantum-learning model is easy to implement as there are only a few ad hoc parameters to be determined. Numerical simulations on the benchmarking MNIST (Mixed National Institute of Standards and Technology) dataset of handwritten digits demonstrate that the model can achieve high performance using only 3-5 qubits without downsizing the dataset, which shows great potential for accomplishing large-scale real-world learning tasks on NISQ processors.
更多查看译文
AI 理解论文
溯源树
样例
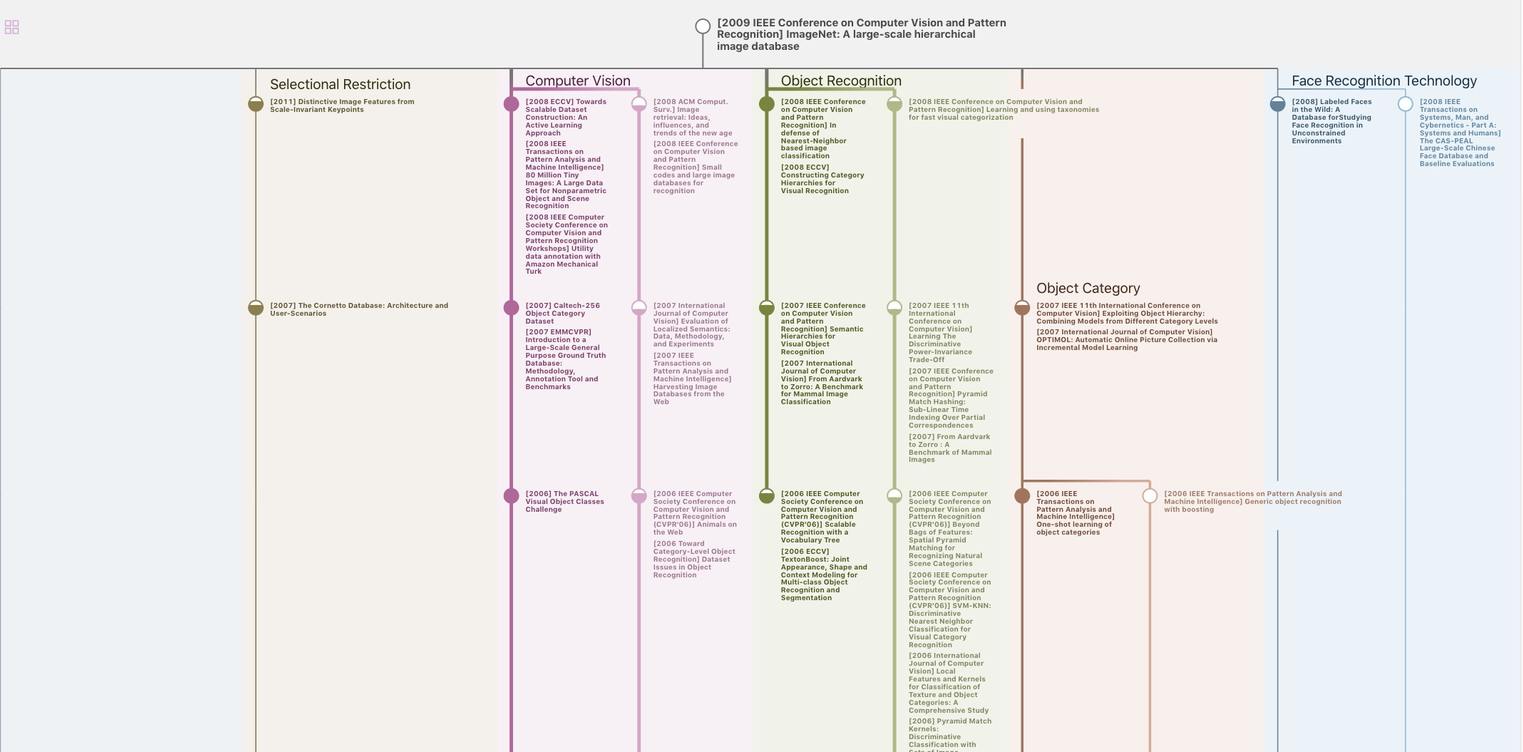
生成溯源树,研究论文发展脉络
Chat Paper
正在生成论文摘要