Dynamic Depth Fusion and Transformation for Monocular 3D Object Detection.
ACCV (1)(2020)
摘要
Visual-based 3D detection is drawing a lot of attention recently. Despite the best efforts from the computer vision researchers visual-based 3D detection remains a largely unsolved problem. This is primarily due to the lack of accurate depth perception provided by LiDAR sensors. Previous works struggle to fuse 3D spatial information and the RGB image effectively. In this paper, we propose a novel monocular 3D detection framework to address this problem. Specifically, we propose to primary contributions: (i) We design an Adaptive Depth-guided Instance Normalization layer to leverage depth features to guide RGB features for high quality estimation of 3D properties. (ii) We introduce a Dynamic Depth Transformation module to better recover accurate depth according to semantic context learning and thus facilitate the removal of depth ambiguities that exist in the RGB image. Experiments show that our approach achieves state-of-the-art on KITTI 3D detection benchmark among current monocular 3D detection works.
更多查看译文
关键词
dynamic depth fusion,3d object detection,object detection
AI 理解论文
溯源树
样例
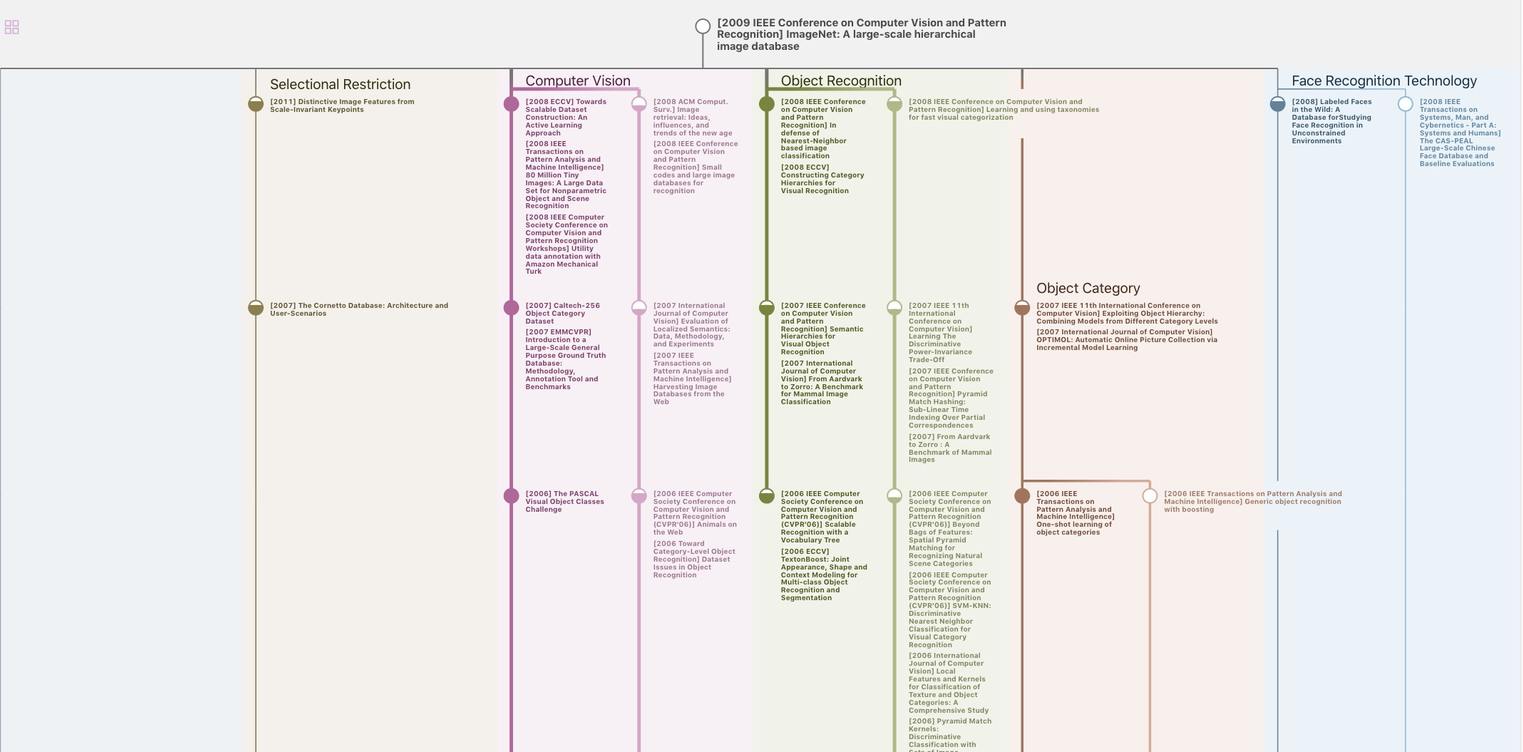
生成溯源树,研究论文发展脉络
Chat Paper
正在生成论文摘要