NIMG-09. PREDICTING OVERALL SURVIVAL OF GLIOBLASTOMA PATIENTS ON MULTI-INSTITUTIONAL HISTOPATHOLOGY STAINED SLIDES USING DEEP LEARNING AND POPULATION-BASED NORMALIZATION
Neuro-oncology(2020)
摘要
Abstract MOTIVATION Glioblastoma is the most common and aggressive adult brain tumor. Clinical histopathologic evaluation is essential for tumor classification, which according to the World Health Organization is associated with prognostic information. Accurate prediction of patient overall survival (OS) from clinical routine baseline histopathology whole slide images (WSI) using advanced computational methods, while considering variations in the staining process, could contribute to clinical decision-making and patient management optimization. METHODS We utilize The Cancer Genome Atlas glioblastoma (TCGA-GBM) collection, comprising multi-institutional hematoxylin and eosin (H&E) stained frozen top-section WSI, genomic, and clinical data from 121 subjects. Data are randomly split into training (80%), validation (10%), and testing (10%) sets, while proportionally keeping the ratio of censored patients. We propose a novel deep learning algorithm to identify survival-discriminative histopathological patterns in a WSI, through feature maps, and quantitatively integrate them with gene expression and clinical data to predict patient OS. The concordance index (C-index) is used to quantify the predictive OS performance. Variations in slide staining are assessed through a novel population-based stain normalization approach, informed of glioblastoma distinct histologic sub-regions and their appearance from 509 H&E stained slides with corresponding anatomical annotations from the Ivy Glioblastoma Atlas Project (IvyGAP). RESULTS C-index was equal to 0.797, 0.713, and 0.703 for the training, validation, and testing data, respectively, prior to stain normalization. Following normalization, staining variations in H&E and ‘E’ gained significant improvements in IvyGAP (pWilcoxon< 0.01) and TCGA-GBM (pWilcoxon< 0.0001) data, respectively. These improvements contributed to further optimizing the C-index to 0.871, 0.777, and 0.780 for the training, validation, and testing data, respectively. CONCLUSIONS Appropriate normalization and integrative deep learning yield accurate OS prediction of glioblastoma patients through H&E slides, generalizable in multi-institutional data, potentially contributing to patient stratification in clinical trials. Our computationally-identified survival-discriminative histopathological patterns can contribute in further understanding glioblastoma.
更多查看译文
AI 理解论文
溯源树
样例
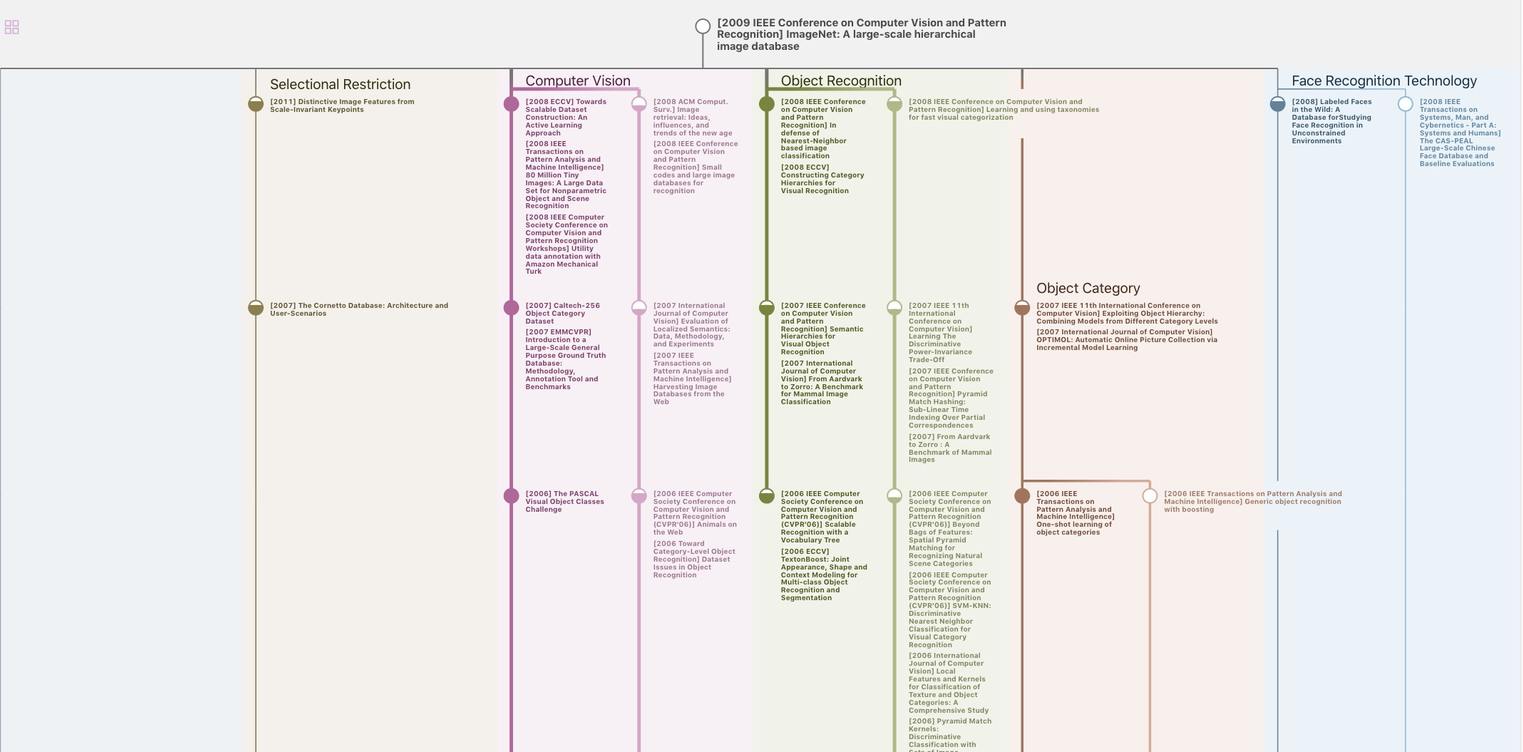
生成溯源树,研究论文发展脉络
Chat Paper
正在生成论文摘要