Energy-Efficient All-Spin BNN Using Voltage-Controlled Spin-Orbit Torque Device for Digit Recognition
IEEE Transactions on Electron Devices(2021)
摘要
Artificial intelligence has been demonstrated for numerous applications including image recognition and processing, internet-of-things (IoT), and speech recognition. Recent neural network (NN) architectures escalating to perform these functionalities require deep-learning and deep NN (DNN) implementation. However, the present algorithm and circuit of DNN suffer from area and energy-efficiency. The usage of an energy-efficient and cost-effective binary NN (BNN) can mitigate the performance bottleneck. The basic component of BNN (the XNOR and accumulate module) is capable of replacing the conventional multiply-and-accumulate (MAC) operation. Among various emerging nonvolatile memories (NVMs), spintronics-based devices are attracting attention for the implementation of the aforementioned architectures. Multilevel voltage-controlled spin-orbit torque -based magnetic memory (MV-SOTM) spin device as synapse and voltage-controlled SOTM (V-SOTM) device as spin-neuron are capable of producing an all-spin NN. This article presents advanced XNOR operation using a computing-in-memory (CiM) mechanism wherein both V-SOTM and MV-SOTM (using series and parallel configuration) based synapses are compared. The MV-SOTM design dissipates 35.51% lesser energy as compared to the 1-bit V-SOTM. Further, the 8-bit synaptic crossbar array is implemented using both the devices with two output spin-neurons. These series and parallel MV-SOTM-based synaptic arrays consume 42.22% and 45.12% lesser power, respectively, as compared to 1-bit V-SOTM based synaptic array. Furthermore, the all-spin BNN design demonstrates a handwritten digit recognition application.
更多查看译文
关键词
Binary neural network (BNN),computing-in-memory (CiM),magnetic memory,neuromorphic computing,voltage-controlled spin-orbit torque (SOT)
AI 理解论文
溯源树
样例
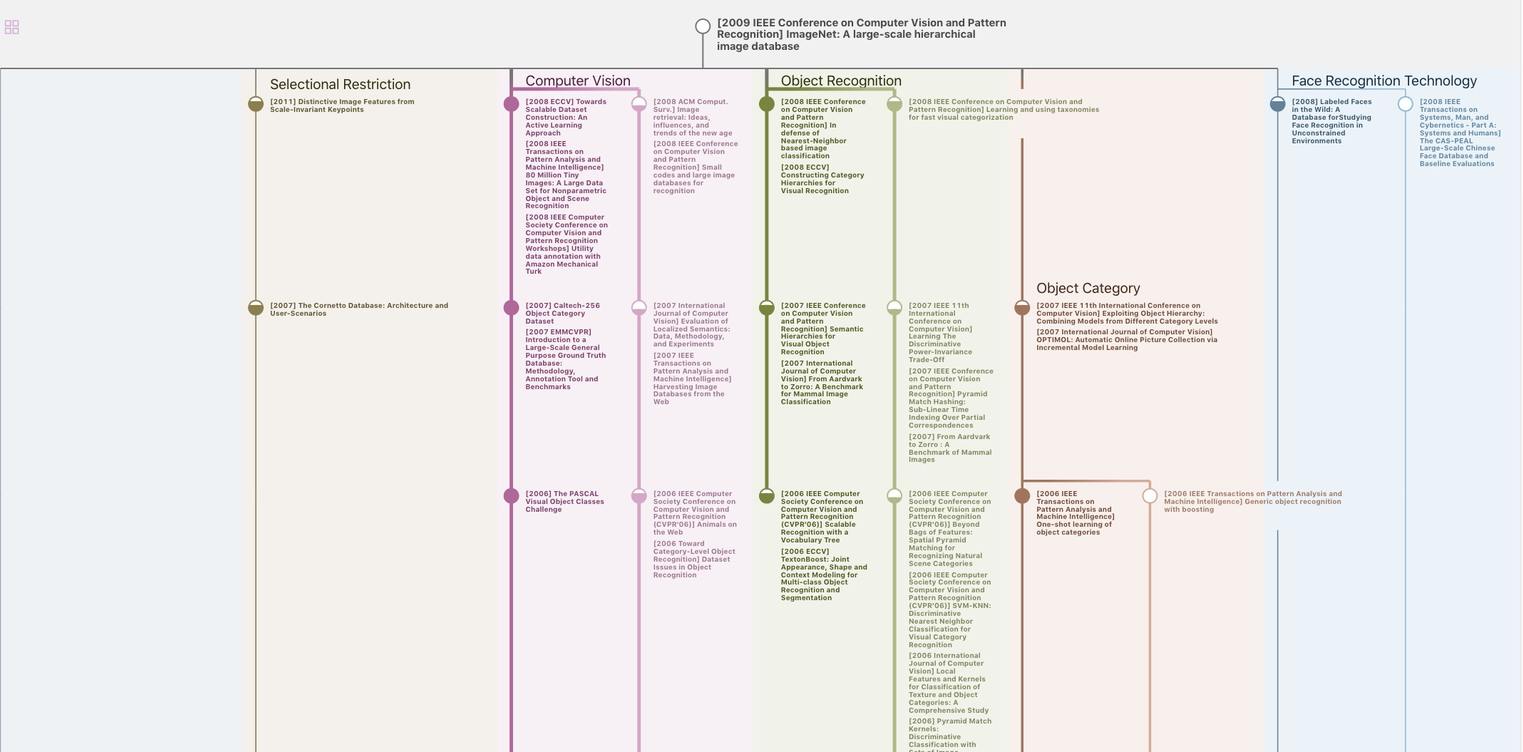
生成溯源树,研究论文发展脉络
Chat Paper
正在生成论文摘要