Low-Power And Reliable Gas Sensing System Based On Recurrent Neural Networks
SENSORS AND ACTUATORS B-CHEMICAL(2021)
摘要
A new gas sensing system using recurrent neural networks (RNNs) is proposed for reliable gas identification. A field-effect-transistor (FET)-type gas sensor with an In2O3 film as a sensing material was fabricated for detecting NO2 and H2S gases. Then, the transient characteristics of the sensor were investigated depending on the operating temperature and gas concentration. Training was conducted in the Pytorch framework (high-level simulation) using RNNs and fully connected neural networks (FCNNs) with the measured sensing data, and the performance of these networks was compared. In a high-level simulation, the RNNs showed better performance (1.94 % error) than that of the FCNNs (3.02 % error) even with fewer neurons and smaller synapse arrays. Using the RNNs, a hardware-based and low-power (0.412 mW) neural system was designed for gas detection with the sensors, synaptic devices, and peripheral circuits. The weights were trained in the high-level simulation and transferred to the conductance of the synaptic devices. Input encoding in the system is performed by pulse-width modulation, and the weighted sum was calculated according to Kirchhoff laws. The system operation in the time domain was verified in the SPICE simulation, and the predicted output of the system matched that of the highlevel simulation within a 0.3 % error.
更多查看译文
关键词
Gas sensing, Field-effect-transistor (FET)-type gas sensor, Neuromorphic system, Recurrent neural network (RNN), Synaptic device, Gas sensing system
AI 理解论文
溯源树
样例
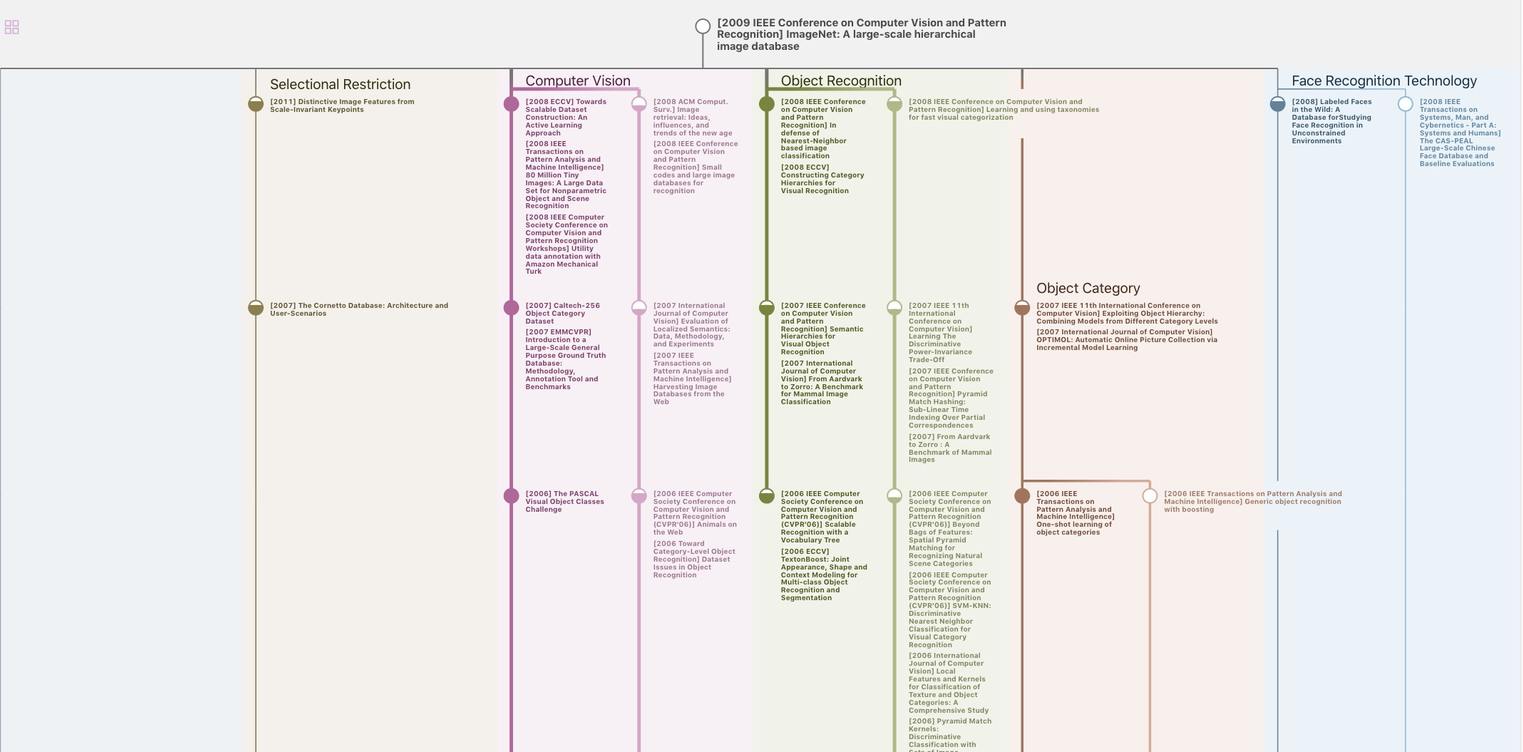
生成溯源树,研究论文发展脉络
Chat Paper
正在生成论文摘要