Metaphor identification: A contextual inconsistency based neural sequence labeling approach
Neurocomputing(2021)
摘要
Metaphor identification helps improve the performance of various natural language understanding tasks such as word sense disambiguation and sentiment analysis. Though many efforts have been made to deal with metaphor identification, most existing studies largely overlook a fact of contextual inconsistency of the metaphors in natural language. We observe that the greater the semantic inconsistency between current word and contextual words is, the more likely the word belongs to the metaphorical category. In this paper, we formulate the metaphor identification as a sequential tagging problem, and then develop a novel contextual inconsistency based neural sequence labeling approach, which can leverage the semantic contextual inconsistency among words of a sentence to address the problem. We propose to rely on distance metric to measure the contextual inconsistency, and evaluate four widely used distance functions in experiments. Experimental results on publicly available datasets validate the benefit of the proposed model over state-of-the-art baselines for metaphor identification.
更多查看译文
关键词
Sequence labeling model,Neural networks,Metaphor identification,Contextual inconsistency
AI 理解论文
溯源树
样例
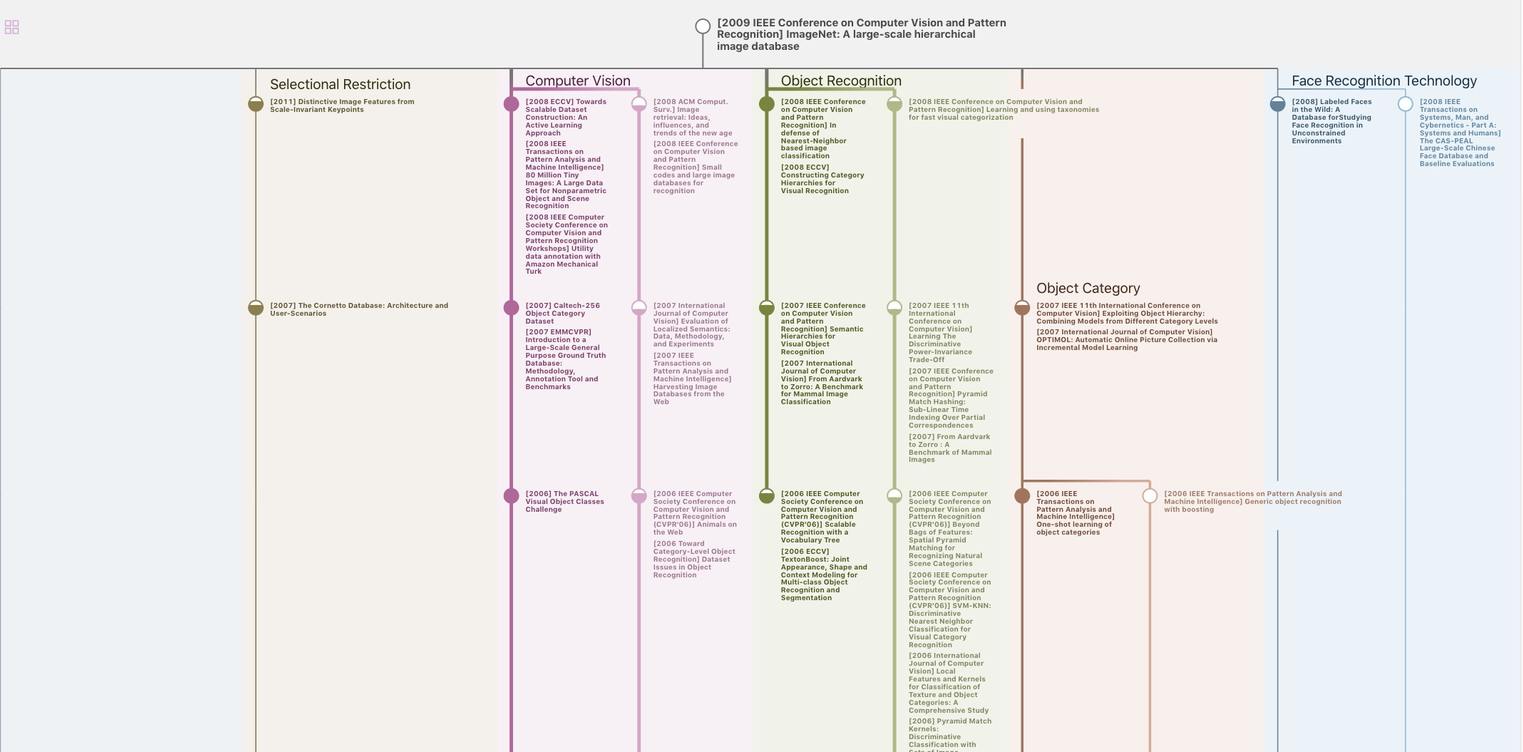
生成溯源树,研究论文发展脉络
Chat Paper
正在生成论文摘要