Machine Learning-Based Regional Scale Intelligent Modeling Of Building Information For Natural Hazard Risk Management
AUTOMATION IN CONSTRUCTION(2021)
摘要
The intensity of many natural hazards, such as hurricanes, floods, tornadoes, etc., are increasing as a consequence of climate change. This increase in intensity coupled with the increase in population density, particularly along the coasts, is only magnifying the impact of such events. In order to quantify and mitigate the risk due to the hazards and to prepare for the potential impacts in a region, it is necessary to collect the information of existing buildings that are pertinent to natural hazard analysis and risk management. Gathering the building information in a regionor city-scale is a laborious and expensive undertaking. This paper presents a framework for regional scale building information generation/gathering to support regional hazard analysis. In this framework, different types of data are acquired from multiple sources (satellite and street view images, property tax assessment data, etc.) and are fused to semantically profile each building in a city. Specifically, deep learning technique is employed to extract building information from street or satellite images. A novel data mining tool is developed to overcome the data scarcity issue, quantify the uncertainty and enrich the data repository. With this framework, building inventories of cities are created to provide the data needed for disaster and risk management planning and simulations.
更多查看译文
AI 理解论文
溯源树
样例
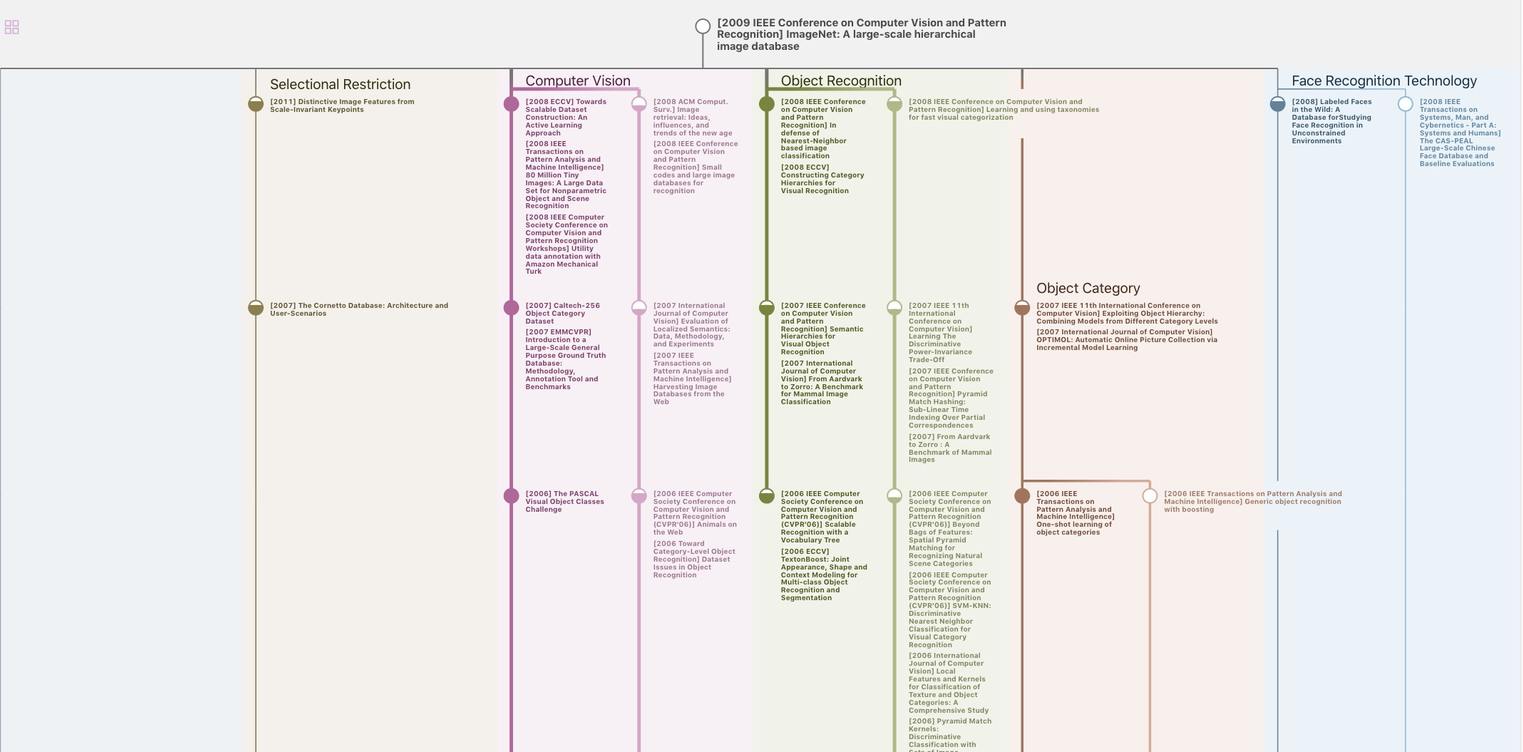
生成溯源树,研究论文发展脉络
Chat Paper
正在生成论文摘要