Support Vector Subset Scan For Spatial Pattern Detection
COMPUTATIONAL STATISTICS & DATA ANALYSIS(2021)
摘要
Discovery of localized and irregularly shaped anomalous patterns in spatial data provides useful context for operational decisions across many policy domains. The support vector subset scan (SVSS) integrates the penalized fast subset scan with a kernel support vector machine classifier to accurately detect spatial clusters without imposing hard constraints on the shape or size of the pattern. The method iterates between (1) efficiently maximizing a penalized log-likelihood ratio over subsets of locations to obtain an anomalous pattern, and (2) learning a high-dimensional decision boundary between locations included in and excluded from the anomalous subset. On each iteration, location-specific penalties to the log-likelihood ratio are assigned according to distance to the decision boundary, encouraging patterns which are spatially compact but potentially highly irregular in shape. SVSS outperforms competing methods for spatial cluster detection at the task of detecting randomly generated patterns in simulated experiments. SVSS enables discovery of practically-useful anomalous patterns for disease surveillance in Chicago, IL, crime hotspot detection in Portland, OR, and pothole cluster detection in Pittsburgh, PA, as demonstrated by experiments using publicly available data sets from these domains. (C) 2020 The Author(s). Published by Elsevier B.V.
更多查看译文
关键词
Anomalous pattern detection, Machine learning, Spatial analysis, Subset scanning
AI 理解论文
溯源树
样例
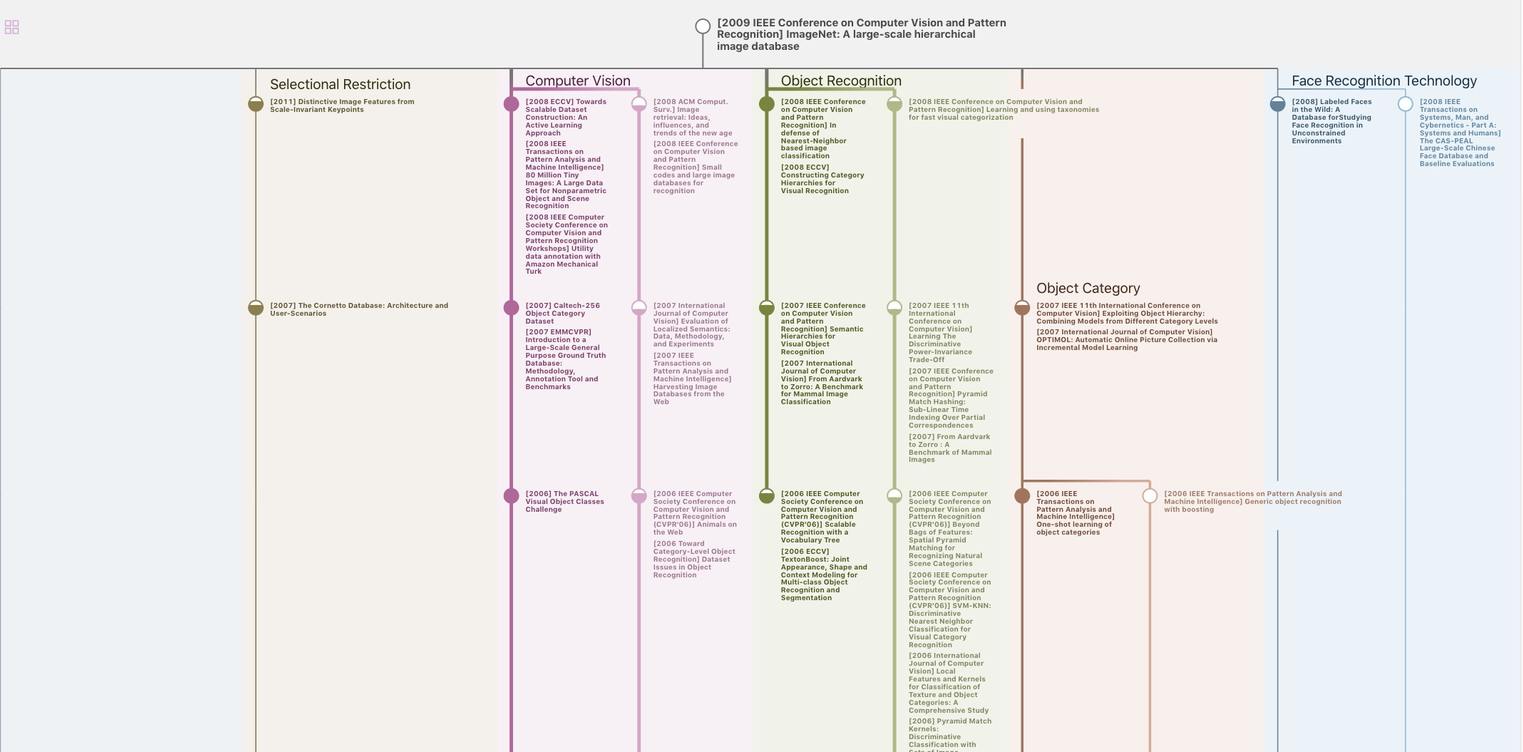
生成溯源树,研究论文发展脉络
Chat Paper
正在生成论文摘要