Automotive Radar Interference Reduction Based On Sparse Bayesian Learning
2020 IEEE RADAR CONFERENCE (RADARCONF20)(2020)
摘要
Automotive radar plays an important role in advanced driver assistant systems to support level-2 automated driving functions. However, the mutual interference between automotive radars increases due to the rising density of radars on the road. Therefore, the radar signal will be distorted to some extent and the performance of radars will degrade if no countermeasures are taken. In this paper, an interference mitigation approach using compressive sensing (CS) and Bayesian learning is introduced. By utilizing the sparsity of the beat signal in the frequency domain, the range-Doppler (RD) spectrum can be reconstructed with the help of undistorted samples in the beat signal. The sparse Bayesian learning method (SBL) is used to estimate the posterior of the signal's sparse representation and to infer the maximally sparse representation by using the Expectation-Maximization (EM) algorithm. It is shown that the SBL-based method has its advantages in signal-to-interference-plus-noise ratio (SINR) and target peak detection in comparison to conventional CS or classical signal reconstruction algorithms like linear predictive coding (LPC).
更多查看译文
关键词
automotive radar, interference mitigation, compressive sensing, sparse Bayesian learning
AI 理解论文
溯源树
样例
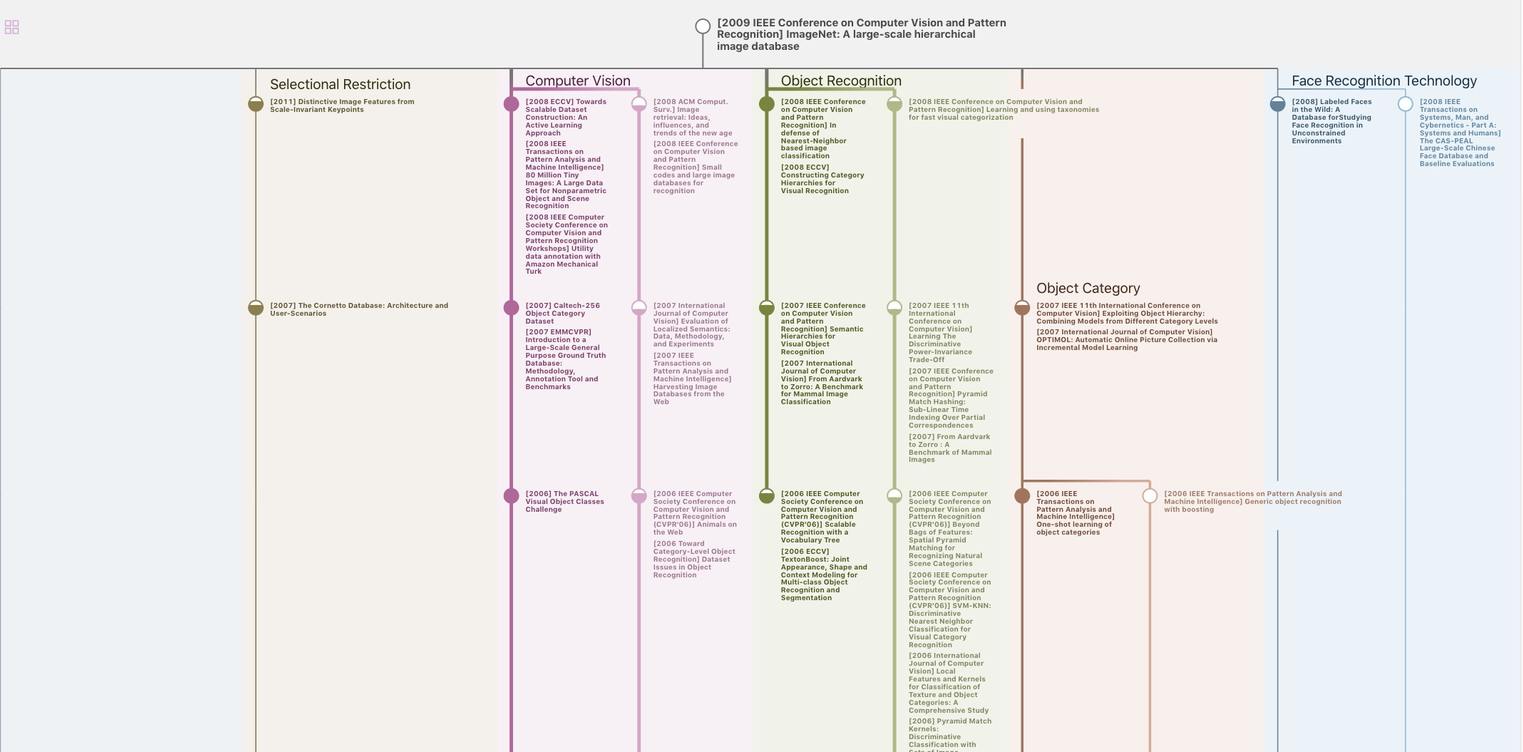
生成溯源树,研究论文发展脉络
Chat Paper
正在生成论文摘要