On oversampling imbalanced data with deep conditional generative models
Expert Systems with Applications(2021)
摘要
Class imbalanced datasets are common in real-world applications ranging from credit card fraud detection to rare disease diagnosis. Recently, deep generative models have proved successful for an array of machine learning problems such as semi-supervised learning, transfer learning, and recommender systems. However their application to class imbalance situations is limited. In this paper, we consider class conditional variants of generative adversarial networks and variational autoencoders and apply them to the imbalance problem. The main question we seek to answer is whether or not deep conditional generative models can effectively learn the distributions of minority classes so as to produce synthetic observations that ultimately lead to improvements in the performance of a downstream classifier. The numerical results show that this is indeed true and that deep generative models outperform traditional oversampling methods in many circumstances, especially in cases of severe imbalance.
更多查看译文
关键词
Deep generative models,Conditional variational autoencoders,Class imbalance,Oversampling
AI 理解论文
溯源树
样例
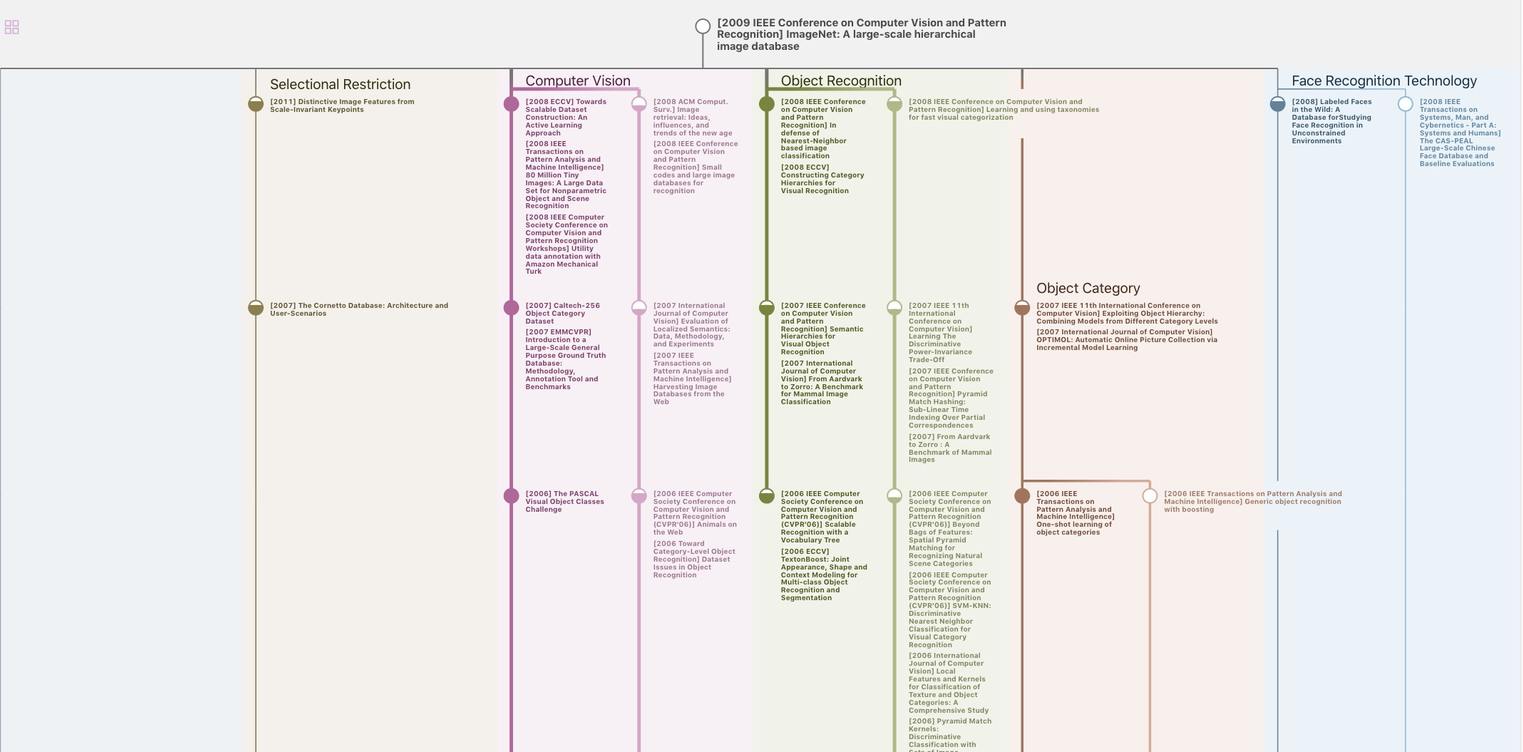
生成溯源树,研究论文发展脉络
Chat Paper
正在生成论文摘要