Nonignorable Missing Data, Single Index Propensity Score and Profile Synthetic Distribution Function
JOURNAL OF BUSINESS & ECONOMIC STATISTICS(2022)
摘要
In missing data problems, missing not at random is difficult to handle since the response probability or propensity score is confounded with the outcome data model in the likelihood. Existing works often assume the propensity score is known up to a finite dimensional parameter. We relax this assumption and consider an unspecified single index model for the propensity score. A pseudo-likelihood based on the complete data is constructed by profiling out a synthetic distribution function that involves the unknown propensity score. The pseudo-likelihood gives asymptotically normal estimates. Simulations show the method compares favorably with existing methods.
更多查看译文
关键词
Missing not at random, Nonignorable missing, Pseudo-conditional likelihood, Single index model, Synthetic distribution
AI 理解论文
溯源树
样例
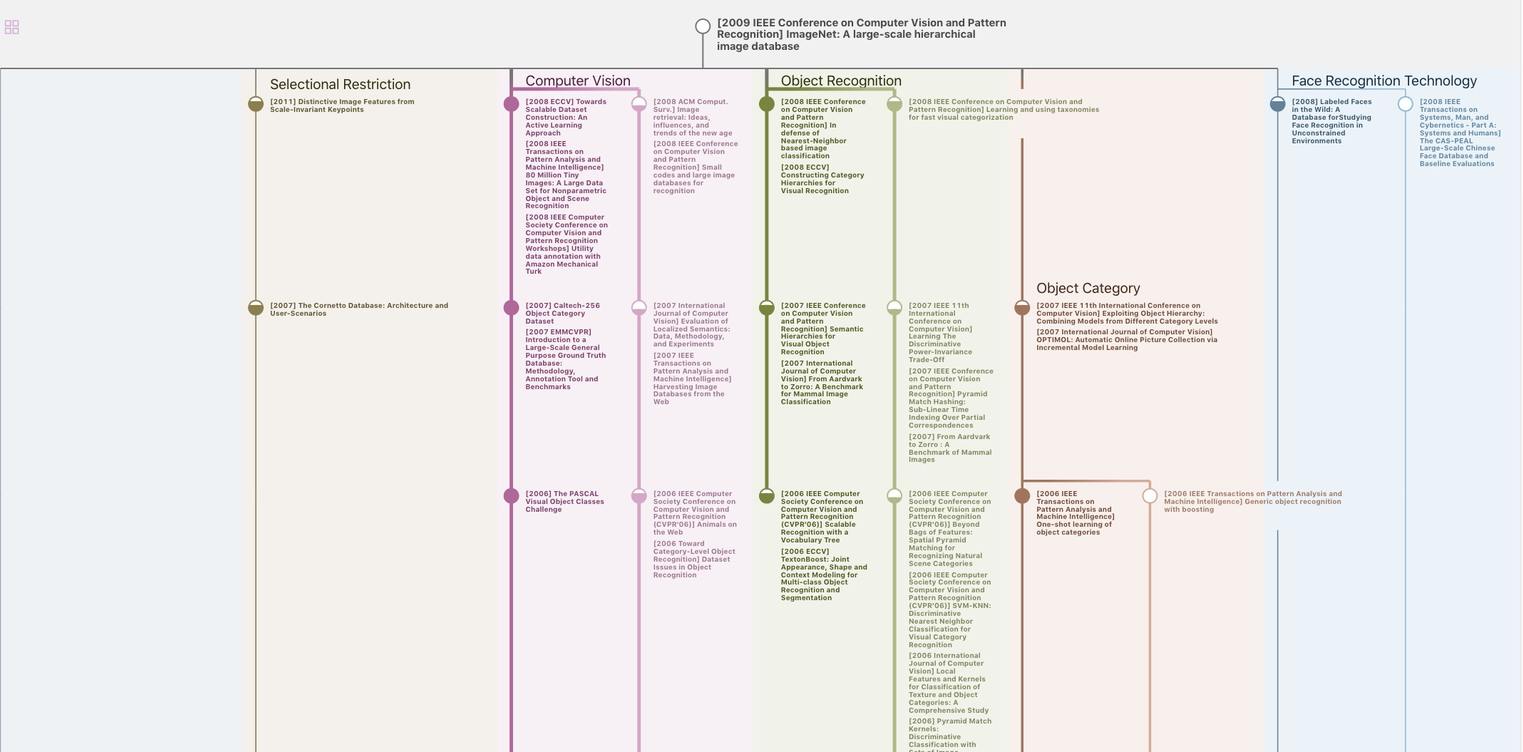
生成溯源树,研究论文发展脉络
Chat Paper
正在生成论文摘要