Novel Architectures for Contractive Autoencoders with Embedded Learning
2020 17th Biennial Baltic Electronics Conference (BEC)(2020)
摘要
A Contractive Autoencoder (CAE) is an unsupervised Artificial Neural Network (ANN) with a regularization term controlling the internal representations. During its operation, the autoencoder extracts dominant features of the input, which can be either used for communication bandwidth reduction or as an input to another neural network. While hardware implementations for the forward pass of various ANNs can be found in literature, this paper proposes three novel architectures for Field Programmable Gate Array (FPGA) based implementations of CAE, for the first time with embedded learning: (i) topography-affine inter-layer communication via crossbar; (ii) efficient carousel-type communication scheme; (iii) optimized carousel-type architecture. All architectures use node-level parallel calculation scheme and have been implemented on a Xilinx Zynq 7020 System On Chip (SoC).
更多查看译文
关键词
communication bandwidth reduction,field programmable gate array,CAE,embedded learning,topography-affine inter-layer communication,unsupervised artificial neural network,unsupervised ANN,dominant feature extraction,carousel-type communication scheme,carousel-type architecture optimization,contractive autoencoders,FPGA,Xilinx Zynq 7020 system on chip,node-level parallel calculation
AI 理解论文
溯源树
样例
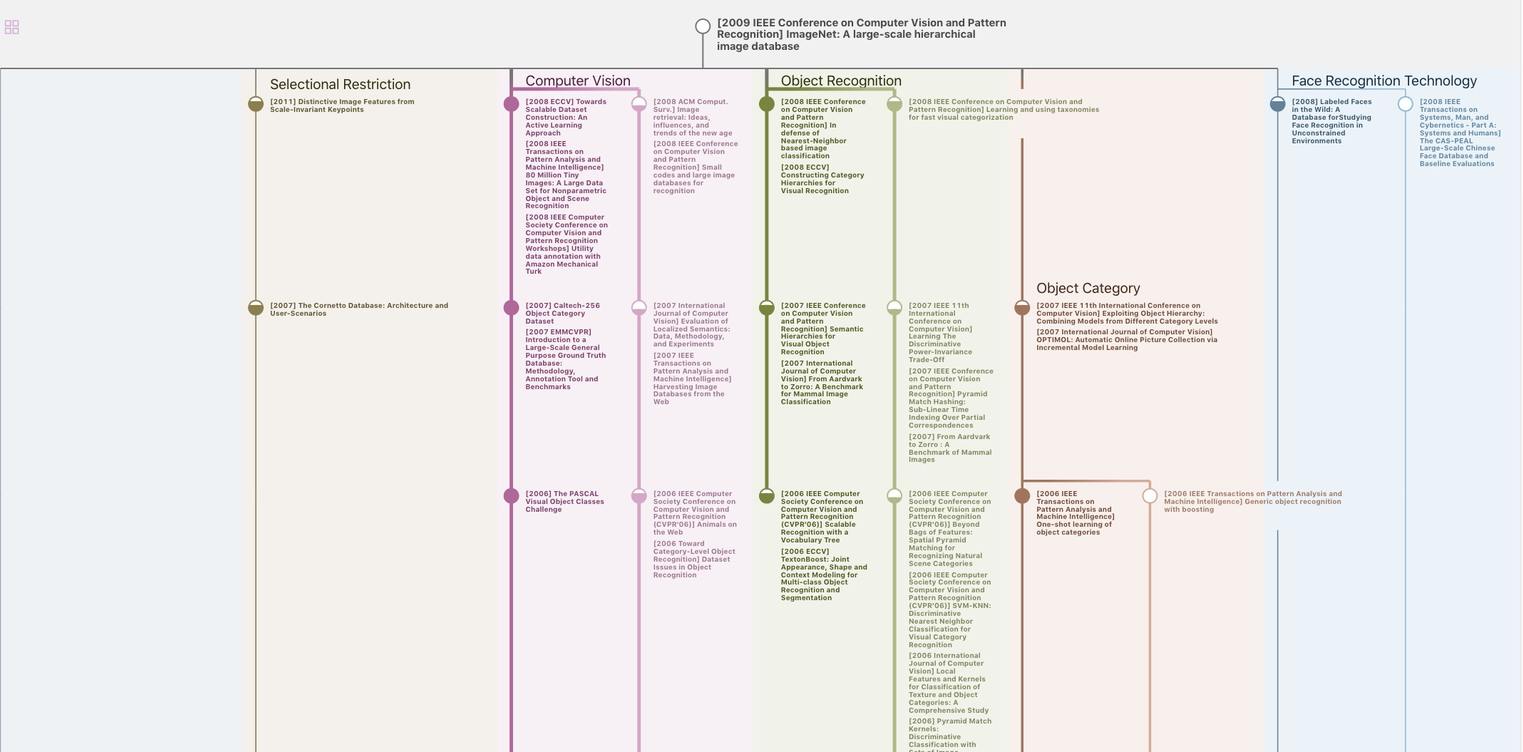
生成溯源树,研究论文发展脉络
Chat Paper
正在生成论文摘要