Class Factorized Variational Auto-encoder for Radar HRRP Target Recognition
2020 IEEE Radar Conference (RadarConf20)(2020)
摘要
In this paper, a class factorized variational auto-encoder (CFVAE) is developed for radar high-resolution range profile (HRRP) target recognition. The proposed model integrates the discriminative information into the deep statistical modeling of HRRP. In detail, the CFVAE utilizes an encoder to project all observations to the deep latent space and then separately defines the generative process from the latent features to observed data in each class, via the collection of specific class-decoders. Due to the stronger ability of each class-decoder to the description of observations in the corresponding class, the CFVAE model can directly assign a test sample into the class that corresponds to the decoder with the minimum reconstruction error, which avoids the mismatch of the extracted features and the back-end classifier. Meanwhile, compared to the traditional variational auto-encoder (VAE) describing the whole dataset with a single decoder, the proposed method has the capacity to give a more accurate description for all observations, thus beneficial to the improvement of features in characterization ability. Moreover, instead of imposing a fixed prior on the latent representations, our CFVAE model learns the conditional prior distribution based on the samples' labels, which further enhances the discrimination of the latent space. Finally, experiments on the measured HRRP dataset demonstrate the promising recognition performance of the proposed method.
更多查看译文
关键词
High-resolution range profile (HRRP),radar automatic target recognition (RATR),variational auto-encoder (VAE),class factorizing,minimum reconstruction error
AI 理解论文
溯源树
样例
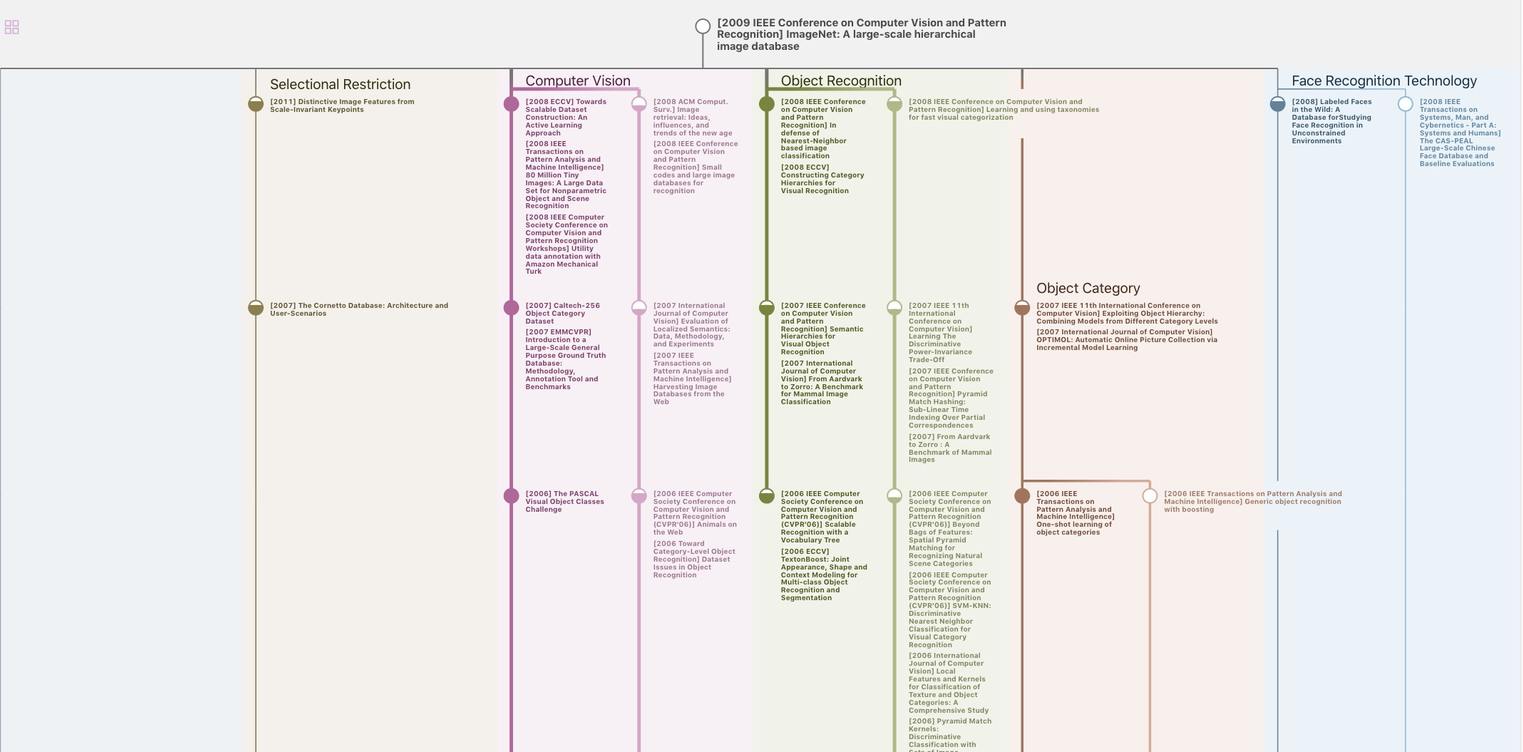
生成溯源树,研究论文发展脉络
Chat Paper
正在生成论文摘要