Quantum Adiabatic Machine Learning By Zooming Into A Region Of The Energy Surface
PHYSICAL REVIEW A(2020)
摘要
Recent work has shown that quantum annealing for machine learning, referred to as QAML, can perform comparably to state-of-the-art machine learning methods with a specific application to Higgs boson classification. We propose QAML-Z, an algorithm that iteratively zooms in on a region of the energy surface by mapping the problem to a continuous space and sequentially applying quantum annealing to an augmented set of weak classifiers. Results on a programmable quantum annealer show that QAML-Z matches classical deep neural network performance at small training set sizes and reduces the performance margin between QAML and classical deep neural networks by almost 50% at large training set sizes, as measured by area under the receiver operating characteristic curve. The significant improvement of quantum annealing algorithms for machine learning and the use of a discrete quantum algorithm on a continuous optimization problem both opens a class of problems that can be solved by quantum annealers and suggests the approach in performance of near-term quantum machine learning towards classical benchmarks.
更多查看译文
关键词
adiabatic machine,quantum,energy surface,machine learning
AI 理解论文
溯源树
样例
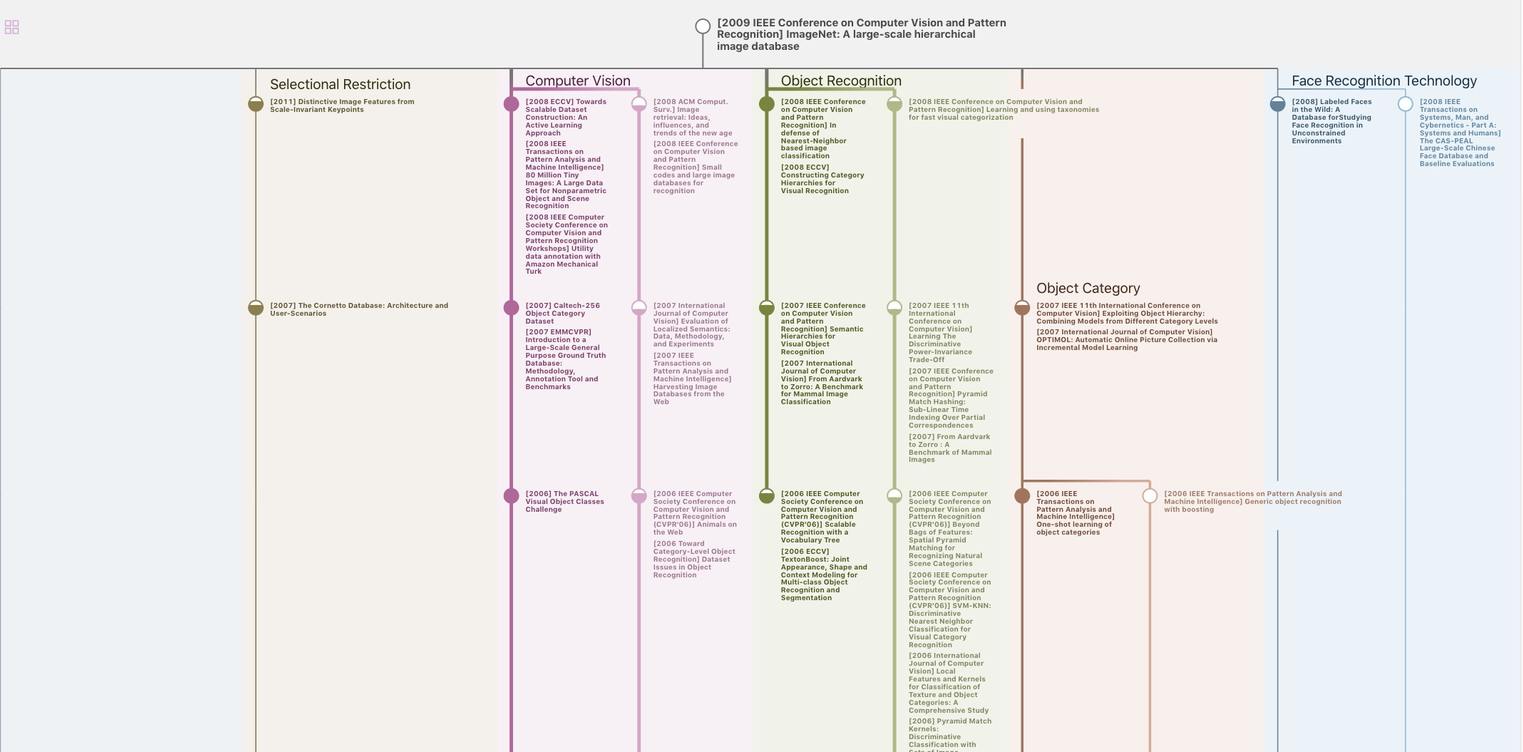
生成溯源树,研究论文发展脉络
Chat Paper
正在生成论文摘要