Residential Electricity Conservation In Response To Auto-Generated, Multi-Featured, Personalized Eco-Feedback Designed For Large Scale Applications With Utilities
ENERGY AND BUILDINGS(2021)
摘要
While past research has shown that providing residents with feedback about their electricity usage can reduce demand and its associated environmental burdens, some questions remain regarding what makes such feedback most effective. We followed the electricity usage of 36 residents who each received 14 feedback messages over 2 months. Using approaches borrowed from Natural-Language-Processing, feed-backs were generated automatically, using 10 features in random combinations. Unlike in previous studies, each resident received varying types of messages over time. In 504 observations, the average prompted reduction in electricity usage was 11 +/- 3%, compared to a control group of 89 residents who received no messages. Feedback types prompting the largest reductions were self-comparisons with one's own earlier usage (average reduction 14%) and messages of high variety from one feedback-cycle to the next (average reduction 16%). Comparisons with neighbors did not prompt higher reductions on average. Instead, they prompted reductions only when a resident's recent usage happened to be higher than the average usage of neighbors, and increases when the reverse was true. This behavior was exhibited by all residents and is likely explained by a norm-conforming mean reversion of residents to their neighbors' average usage, rather than an anti-conform "boomerang" behavior previously suggested in similar contexts. (C) 2020 Elsevier B.V. All rights reserved.
更多查看译文
关键词
Energy conservation, Electricity usage, Eco-feedback, Social comparisons, Natural language processing, Boomerang effect, Sentiment, Mean reversion
AI 理解论文
溯源树
样例
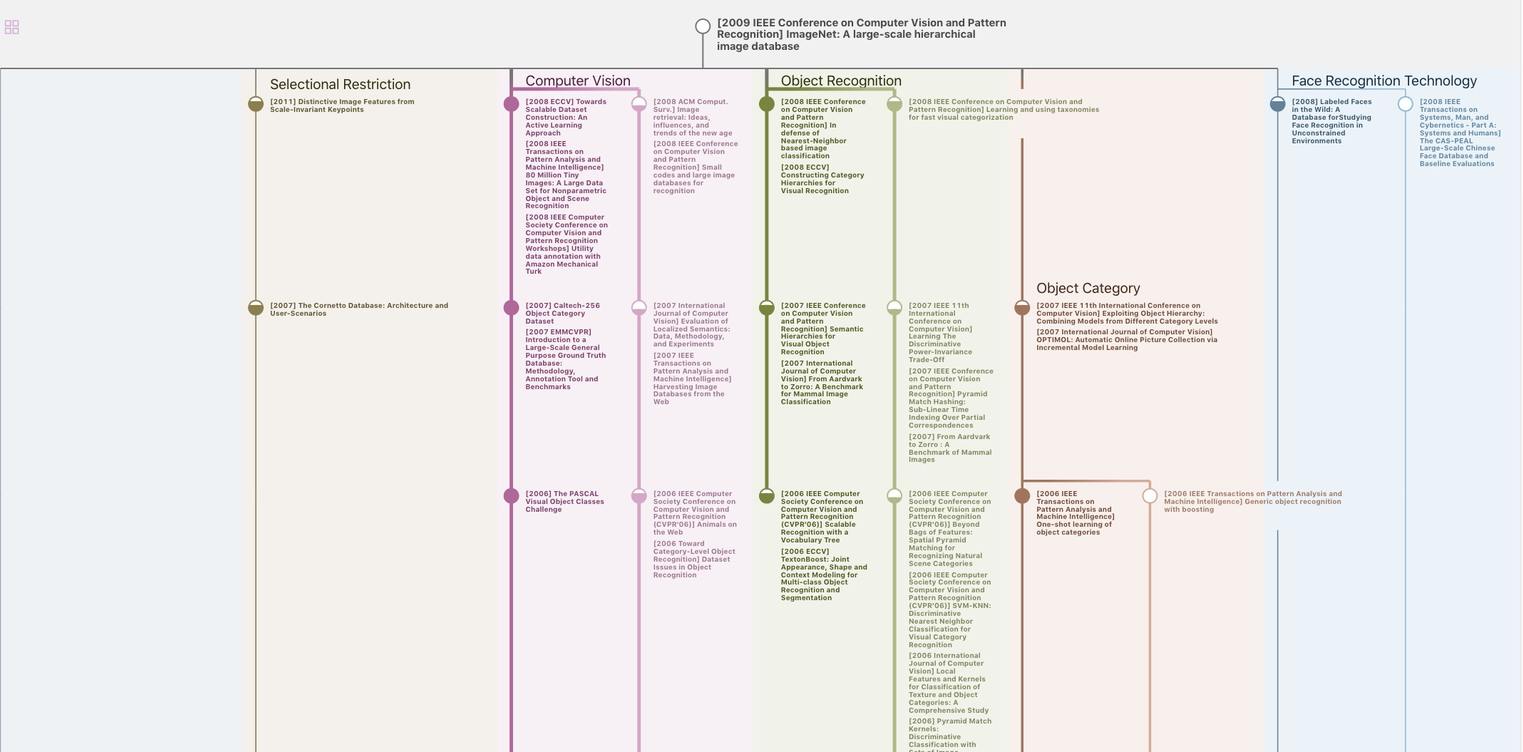
生成溯源树,研究论文发展脉络
Chat Paper
正在生成论文摘要