Gaussian Mixture Model With Feature Selection: An Embedded Approach
COMPUTERS & INDUSTRIAL ENGINEERING(2021)
摘要
Gaussian Mixture Model (GMM) is a popular clustering algorithm due to its neat statistical properties, which enable the "soft" clustering and the determination of the number of clusters. Expectation-Maximization (EM) is usually applied to estimate the GMM parameters. While promising, the inclusion of features that are not contributing to clustering may confuse the model and increase computational cost. Recognizing the issue, in this paper, we propose a new algorithm, termed Expectation Selection Maximization (ESM), by adding a feature selection step (5). Specifically, we introduce a relevancy index (RI), a metric indicating the probability of assigning a data point to a specific clustering group. The RI index reveals the contribution of the feature to the clustering process thus can assist the feature selection. We conduct theoretical analysis to justify the use of RI for feature selection. Also, to demonstrate the efficacy of the proposed ESM, two synthetic datasets, four benchmark datasets, and an Alzheimer's Disease dataset are studied.
更多查看译文
关键词
Gaussian Mixture Model (GMM), Expectation Maximization (EM), Feature selection
AI 理解论文
溯源树
样例
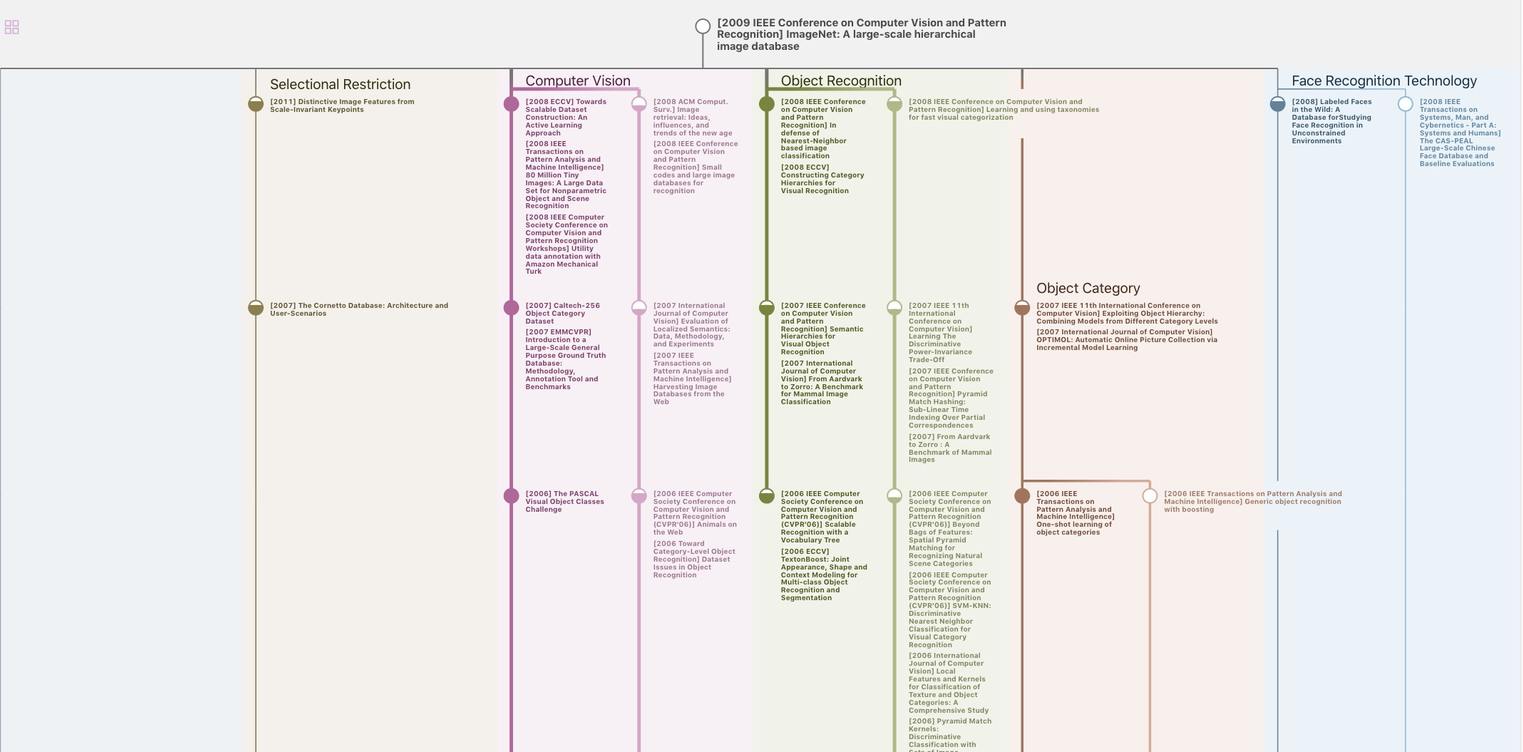
生成溯源树,研究论文发展脉络
Chat Paper
正在生成论文摘要