Subspace-Based Learning for Automatic Dysarthric Speech Detection
IEEE Signal Processing Letters(2021)
摘要
To assist the clinical diagnosis and treatment of speech dysarthria, automatic dysarthric speech detection techniques providing reliable and cost-effective assessment are indispensable. Based on clinical evidence on spectro-temporal distortions associated with dysarthric speech, we propose to automatically discriminate between healthy and dysarthric speakers exploiting spectro-temporal subspaces of speech. Spectro-temporal subspaces are extracted using singular value decomposition, and dysarthric speech detection is achieved by applying a subspace-based discriminant analysis. Experimental results on databases of healthy and dysarthric speakers for different languages and pathologies show that the proposed subspace-based approach using temporal subspaces is more advantageous than using spectral subspaces, also outperforming several state-of-the-art automatic dysarthric speech detection techniques.
更多查看译文
关键词
Spectral subspace,temporal subspace,grassmann discriminant analysis,dysarthria,SVD
AI 理解论文
溯源树
样例
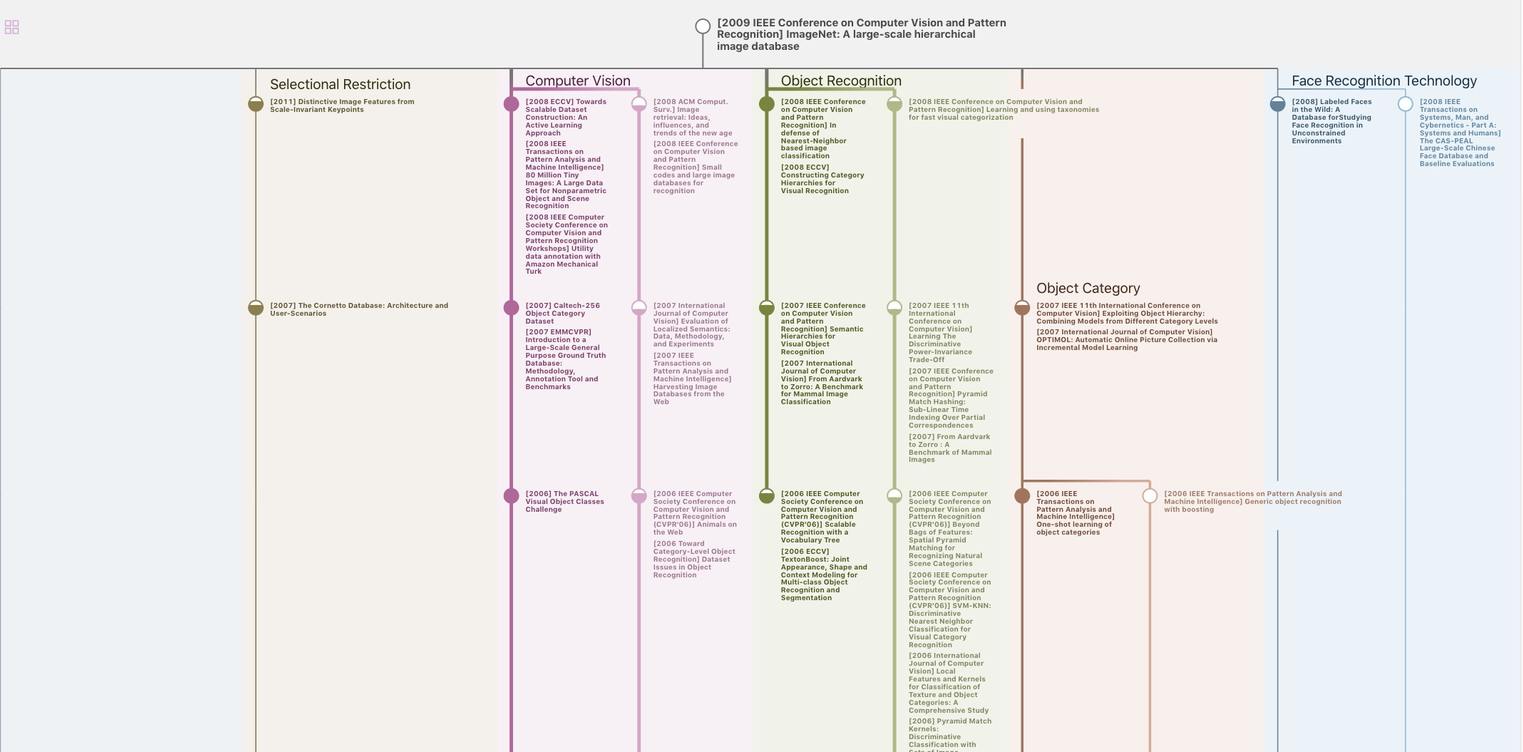
生成溯源树,研究论文发展脉络
Chat Paper
正在生成论文摘要