Continual Learning of Generative Models With Limited Data: From Wasserstein-1 Barycenter to Adaptive Coalescence
arxiv(2023)
摘要
Learning generative models is challenging for a network edge node with limited data and computing power. Since tasks in similar environments share a model similarity, it is plausible to leverage pretrained generative models from other edge nodes. Appealing to optimal transport theory tailored toward Wasserstein-1 generative adversarial networks (WGANs), this study aims to develop a framework that systematically optimizes continual learning of generative models using local data at the edge node while exploiting adaptive coalescence of pretrained generative models. Specifically, by treating the knowledge transfer from other nodes as Wasserstein balls centered around their pretrained models, continual learning of generative models is cast as a constrained optimization problem, which is further reduced to a Wasserstein-1 barycenter problem. A two-stage approach is devised accordingly: 1) the barycenters among the pretrained models are computed offline, where displacement interpolation is used as the theoretic foundation for finding adaptive barycenters via a "recursive" WGAN configuration and 2) the barycenter computed offline is used as metamodel initialization for continual learning, and then, fast adaptation is carried out to find the generative model using the local samples at the target edge node. Finally, a weight ternarization method, based on joint optimization of weights and threshold for quantization, is developed to compress the generative model further. Extensive experimental studies corroborate the effectiveness of the proposed framework.
更多查看译文
关键词
Adaptation models,Data models,Computational modeling,Optimization,Solid modeling,Task analysis,Servers,Continual learning,generative adversarial networks (GANs),optimal transport theory,Wasserstein barycenters
AI 理解论文
溯源树
样例
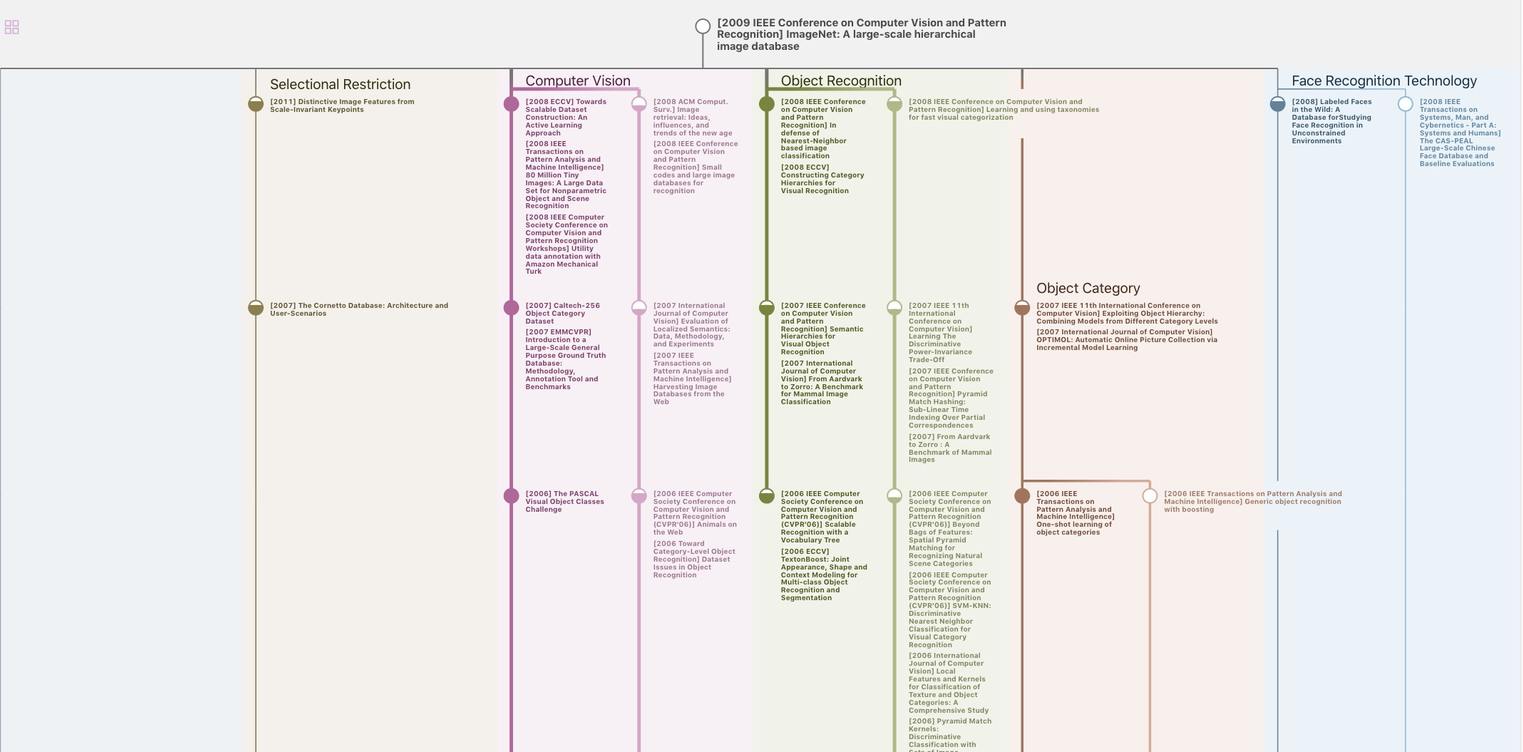
生成溯源树,研究论文发展脉络
Chat Paper
正在生成论文摘要