End-to-end online performance data capture and analysis for scientific workflows
Future Generation Computer Systems(2021)
摘要
With the increased prevalence of employing workflows for scientific computing and a push towards exascale computing, it has become paramount that we are able to analyze characteristics of scientific applications to better understand their impact on the underlying infrastructure and vice-versa. Such analysis can help drive the design, development, and optimization of these next generation systems and solutions. In this paper, we present the architecture, integrated with existing well-established and newly developed tools, to collect online performance statistics of workflow executions from various, heterogeneous sources and publish them in a distributed database (Elasticsearch). Using this architecture, we are able to correlate online workflow performance data, with data from the underlying infrastructure, and present them in a useful and intuitive way via an online dashboard. We have validated our approach by executing two classes of real-world workflows, both under normal and anomalous conditions. The first is an I/O-intensive genome analysis workflow; the second, a CPU- and memory-intensive material science workflow. Based on the data collected in Elasticsearch, we are able to demonstrate that we can correctly identify anomalies that we injected. The resulting end-to-end data collection of workflow performance data is an important resource of training data for automated machine learning analysis.
更多查看译文
关键词
Scientific workflows,Online performance monitoring,Extreme scale
AI 理解论文
溯源树
样例
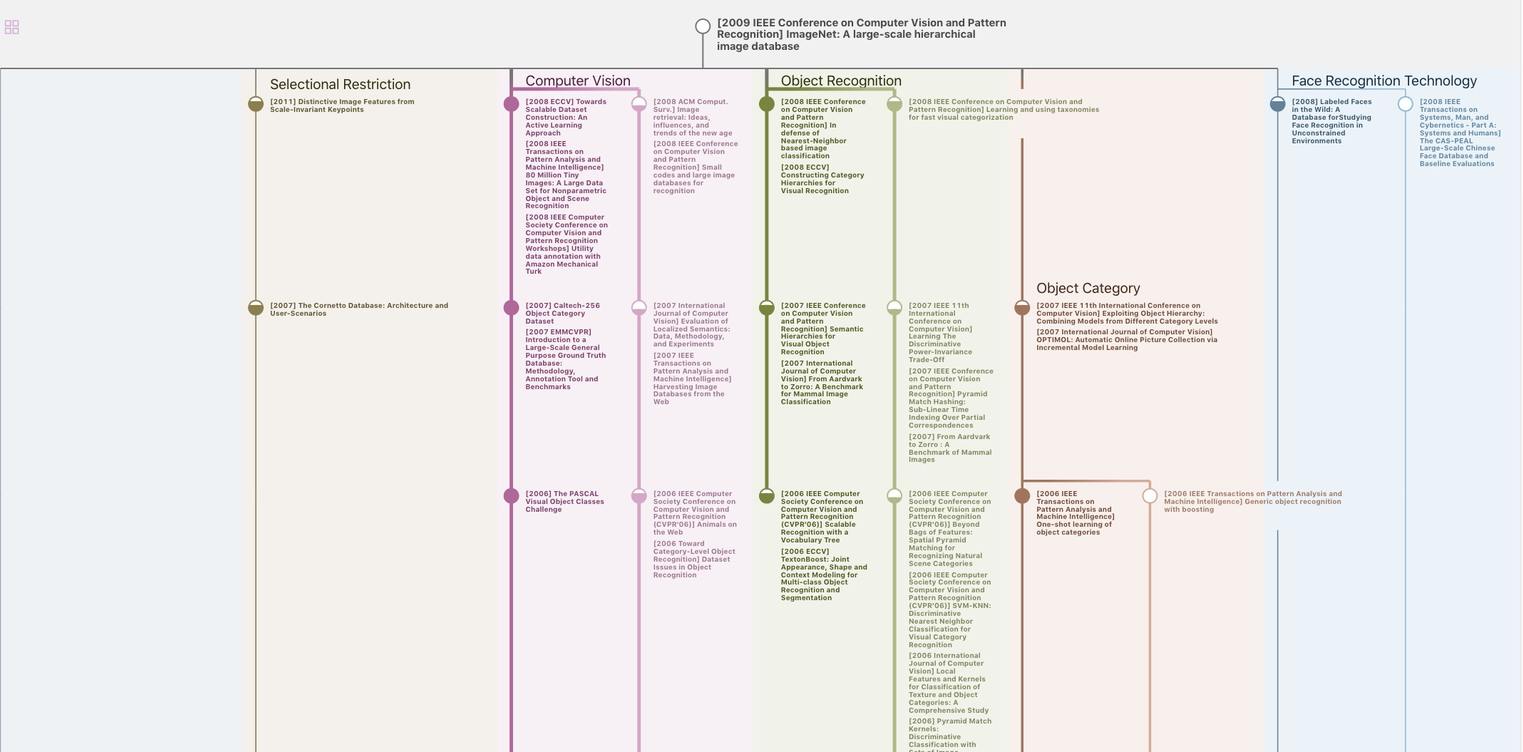
生成溯源树,研究论文发展脉络
Chat Paper
正在生成论文摘要