A regression-based model evaluation of the Curie temperature of transition-metal rare-earth compounds
Journal of Physics Conference Series(2019)
摘要
The Curie temperature (T-C) of RT binary compounds consisting of 3d transition-metal (T) and 4f rare-earth elements (R) is analyzed systematically by a developed machine learning technique called kernel regression-based model evaluation. Twenty-one descriptive variables were designed assuming completely obtained information of the T-C. Multiple kernel regression analyses with different kernel types: cosine, linear, Gaussian, polynomial, and Laplacian kernels were implemented and examined. All possible descriptive variable combinations were generated to construct the corresponding prediction models. As a result, by appropriate combinations between descriptive variable sets and kernel formulations, we demonstrate that a number of kernel regression models can accurately reproduce the T-C of the RT compounds. The relevance of descriptive variables for predicting T-C are systematically investigated. The results indicate that the rare-earth concentration is the most relevant variable in the T-C phenomenon. We demonstrate that the regression-based model selection technique can be applied to learn the relationship between the descriptive variables and the actuation mechanism of the corresponding physical phenomenon, i.e., T-C in the present case.
更多查看译文
AI 理解论文
溯源树
样例
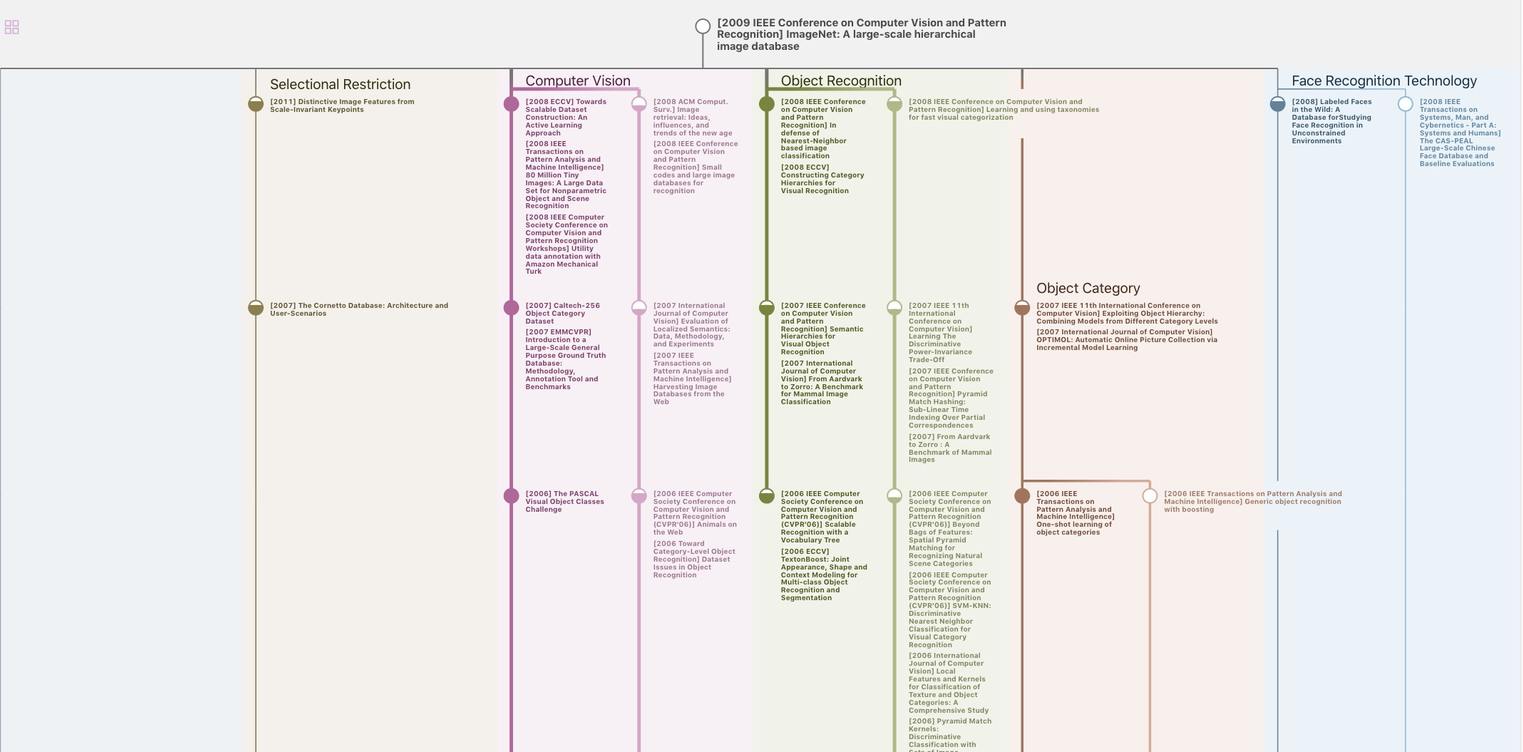
生成溯源树,研究论文发展脉络
Chat Paper
正在生成论文摘要