Crossbreeding in Random Forest
arxiv(2021)
摘要
Ensemble learning methods are designed to benefit from multiple learning algorithms for better predictive performance. The tradeoff of this improved performance is slower speed and larger size of ensemble learning systems compared to single learning systems. In this paper, we present a novel approach to deal with this problem in Random Forest (RF) as one of the most powerful ensemble methods. The method is based on crossbreeding of the best tree branches to increase the performance of RF in space and speed while keeping the performance in the classification measures. The proposed approach has been tested on a group of synthetic and real datasets and compared to the standard RF approach. Several evaluations have been conducted to determine the effects of the Crossbred RF (CRF) on the accuracy and the number of trees in a forest. The results show better performance of CRF compared to RF.
更多查看译文
关键词
random forest
AI 理解论文
溯源树
样例
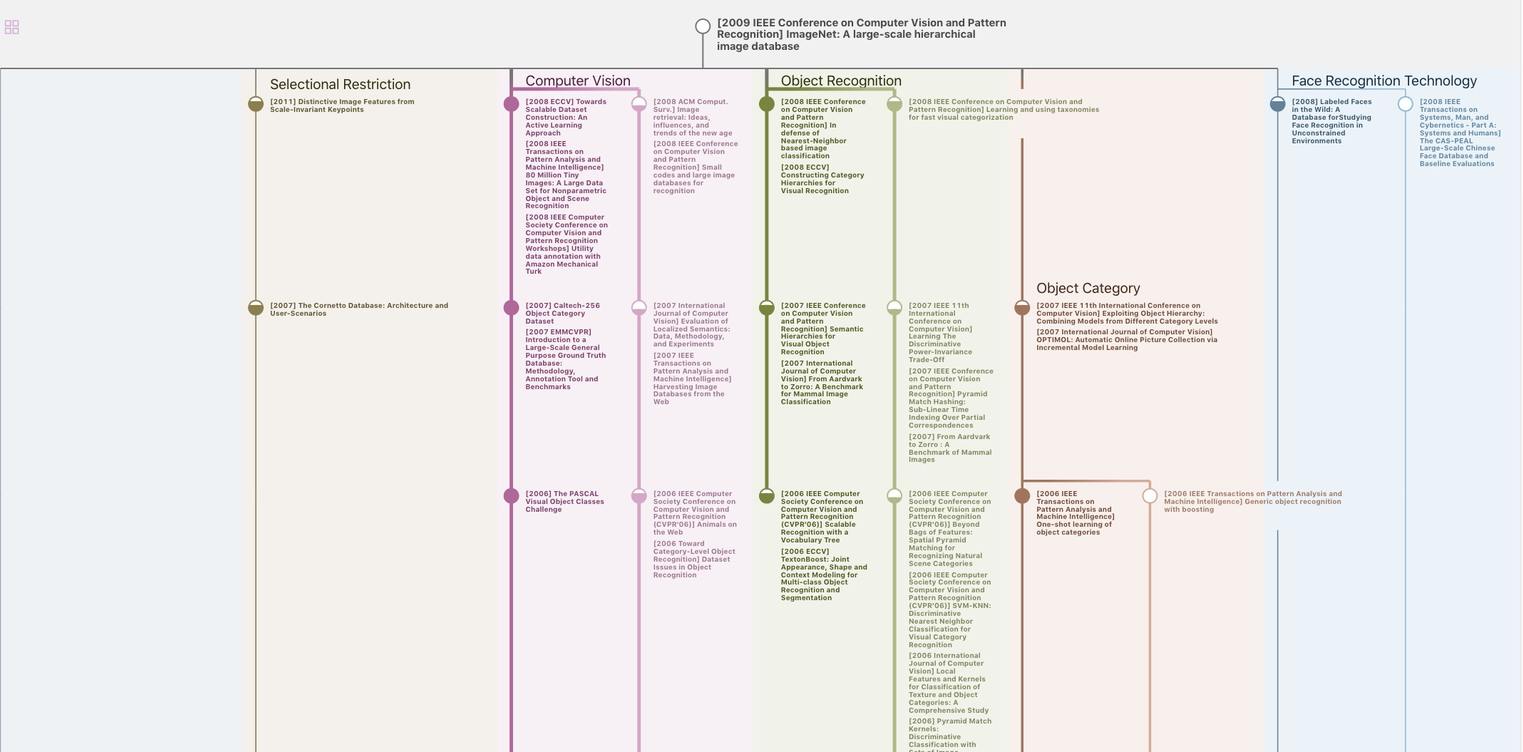
生成溯源树,研究论文发展脉络
Chat Paper
正在生成论文摘要