FWB-NET:FRONT WHITE BALANCE NETWORK FOR COLOR SHIFT CORRECTION IN SINGLE IMAGE DEHAZING VIA ATMOSPHERIC LIGHT ESTIMATION
2021 IEEE INTERNATIONAL CONFERENCE ON ACOUSTICS, SPEECH AND SIGNAL PROCESSING (ICASSP 2021)(2021)
摘要
In recent years, single image dehazing deep models based on Atmospheric Scattering Model (ASM) have achieved remarkable results. But the dehazing outputs of those models suffer from color shift. Analyzing the ASM model shows that the atmospheric light factor (ALF) is set as a scalar which indicates ALF is constant for whole image. However, for images taken in real-world, the illumination is not uniformly distributed over whole image which brings model mismatch and possibly results in color shift of the deep models using ASM. Bearing this in mind, in this study, first, a new non-homogeneous atmospheric scattering model (NH-ASM) is proposed for improving image modeling of hazy images taken under complex illumination conditions. Second, a new U-Net based front white balance module (FWB-Module) is dedicatedly designed to correct color shift before generating dehazing result via atmospheric light estimation. Third, a new FWB loss is innovatively developed for training FWB-Module, which imposes penalty on color shift. In the end, based on NH-ASM and front white balance technology, an end-to-end CNN-based color-shift-restraining dehazing network is developed, termed as FWB-Net. Experimental results demonstrate the effectiveness and superiority of our proposed FWB-Net for dehazing on both synthetic and real-world images.
更多查看译文
关键词
Single image dehazing, atmospheric scattering model, color shift correction, front white balance
AI 理解论文
溯源树
样例
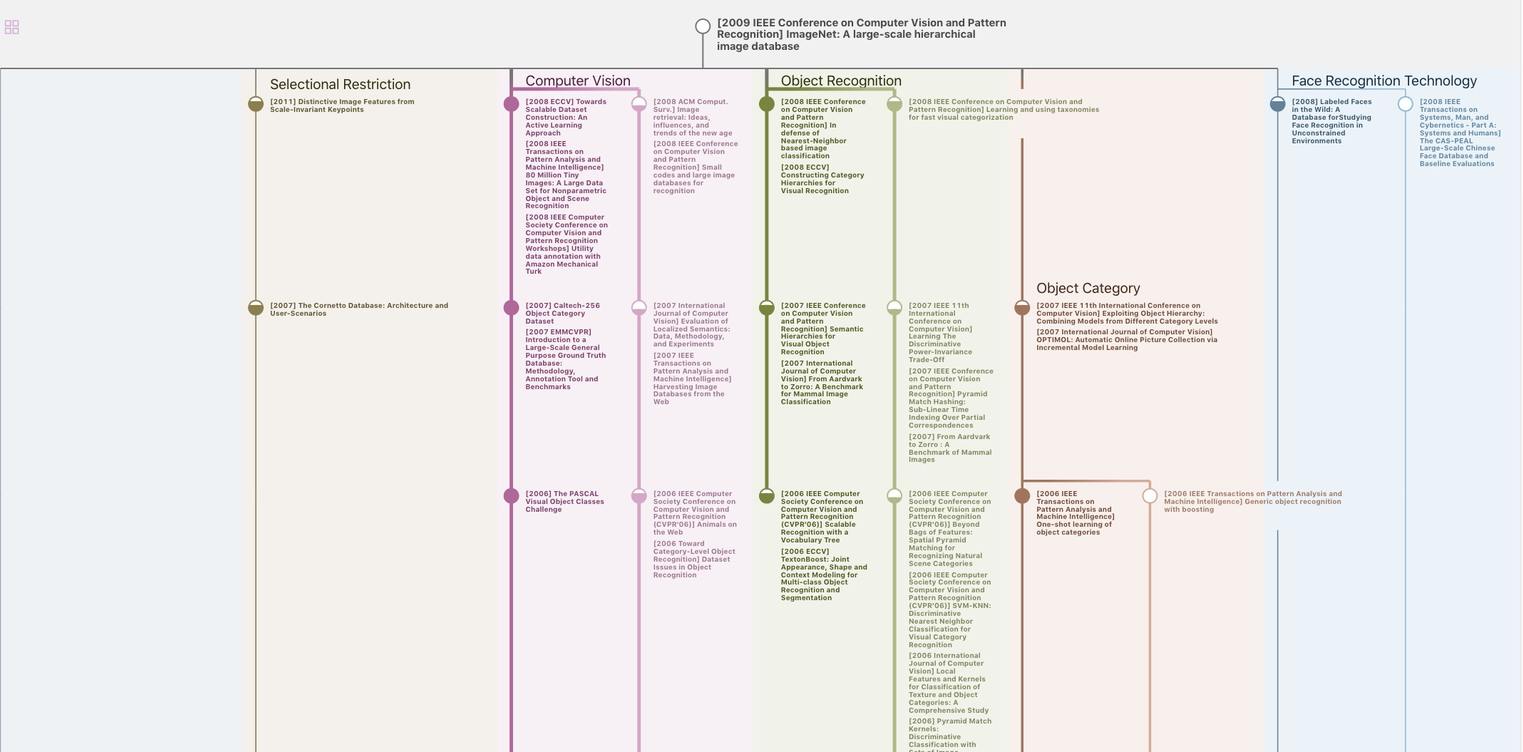
生成溯源树,研究论文发展脉络
Chat Paper
正在生成论文摘要