Marius: Learning Massive Graph Embeddings On A Single Machine
arxiv(2021)
摘要
We propose a new framework for computing the embeddings of large-scale graphs on a single machine. A graph embedding is a fixed length vector representation for each node (and/or edge-type) in a graph and has emerged as the de-facto approach to apply modern machine learning on graphs. We identify that current systems for learning the embeddings of large-scale graphs are bottlenecked by data movement, which results in poor resource utilization and inefficient training. These limitations require state-of-the-art systems to distribute training across multiple machines. We propose Marius, a system for efficient training of graph embeddings that leverages partition caching and buffer-aware data orderings to minimize disk access and interleaves data movement with computation to maximize utilization. We compare Marius against two state-of-the-art industrial systems on a diverse array of benchmarks. We demonstrate that Marius achieves the same level of accuracy but is up to one order of magnitude faster. We also show that Marius can scale training to datasets an order of magnitude beyond a single machine's GPU and CPU memory capacity, enabling training of configurations with more than a billion edges and 550 GB of total parameters on a single machine with 16 GB of GPU memory and 64 GB of CPU memory. Marius is opensourced at www.marius-project.org.
更多查看译文
关键词
Graph (abstract data type),Discrete mathematics,Computer science
AI 理解论文
溯源树
样例
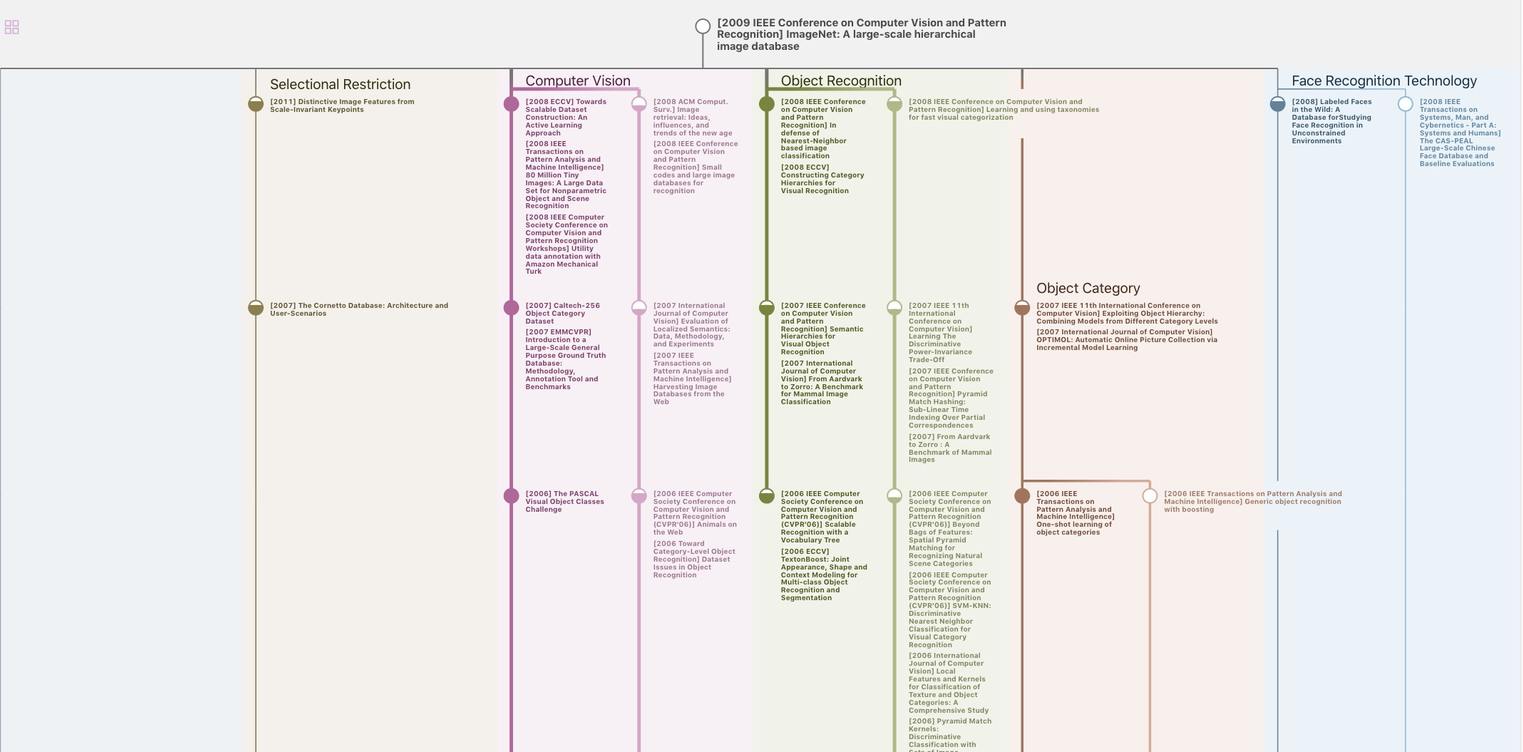
生成溯源树,研究论文发展脉络
Chat Paper
正在生成论文摘要