The difficulty of computing stable and accurate neural networks: On the barriers of deep learning and Smale's 18th problem
PROCEEDINGS OF THE NATIONAL ACADEMY OF SCIENCES OF THE UNITED STATES OF AMERICA(2022)
摘要
Deep learning (DL) has had unprecedented success and is now entering scientific computing with full force. However, current DL methods typically suffer from instability, even when universal approximation properties guarantee the existence of stable neural networks (NNs). We address this paradox by demonstrating basic well-conditioned problems in scientific computing where one can prove the existence of NNs with great approximation qualities; however, there does not exist any algorithm, even randomized, that can train (or compute) such a NN. For any positive integers K > 2 and L, there are cases where simultaneously 1) no randomized training algorithm can compute a NN correct to K digits with probability greater than 1/2; 2) there exists a deterministic training algorithm that computes a NN with K - 1 correct digits, but any such (even randomized) algorithm needs arbitrarily many training data; and 3) there exists a deterministic training algorithm that computes a NN with K - 2 correct digits using no more than L training samples. These results imply a classification theory describing conditions under which (stable) NNs with a given accuracy can be computed by an algorithm. We begin this theory by establishing sufficient conditions for the existence of algorithms that compute stable NNs in inverse problems. We introduce fast iterative restarted networks (FIRENETs), which we both prove and numerically verify are stable. Moreover, we prove that only O(vertical bar log(epsilon)vertical bar) layers are needed for an 6-accurate solution to the inverse problem.
更多查看译文
关键词
stability and accuracy, AI and deep learning, inverse problems, Smale's 18th problem, solvability complexity index hierarchy
AI 理解论文
溯源树
样例
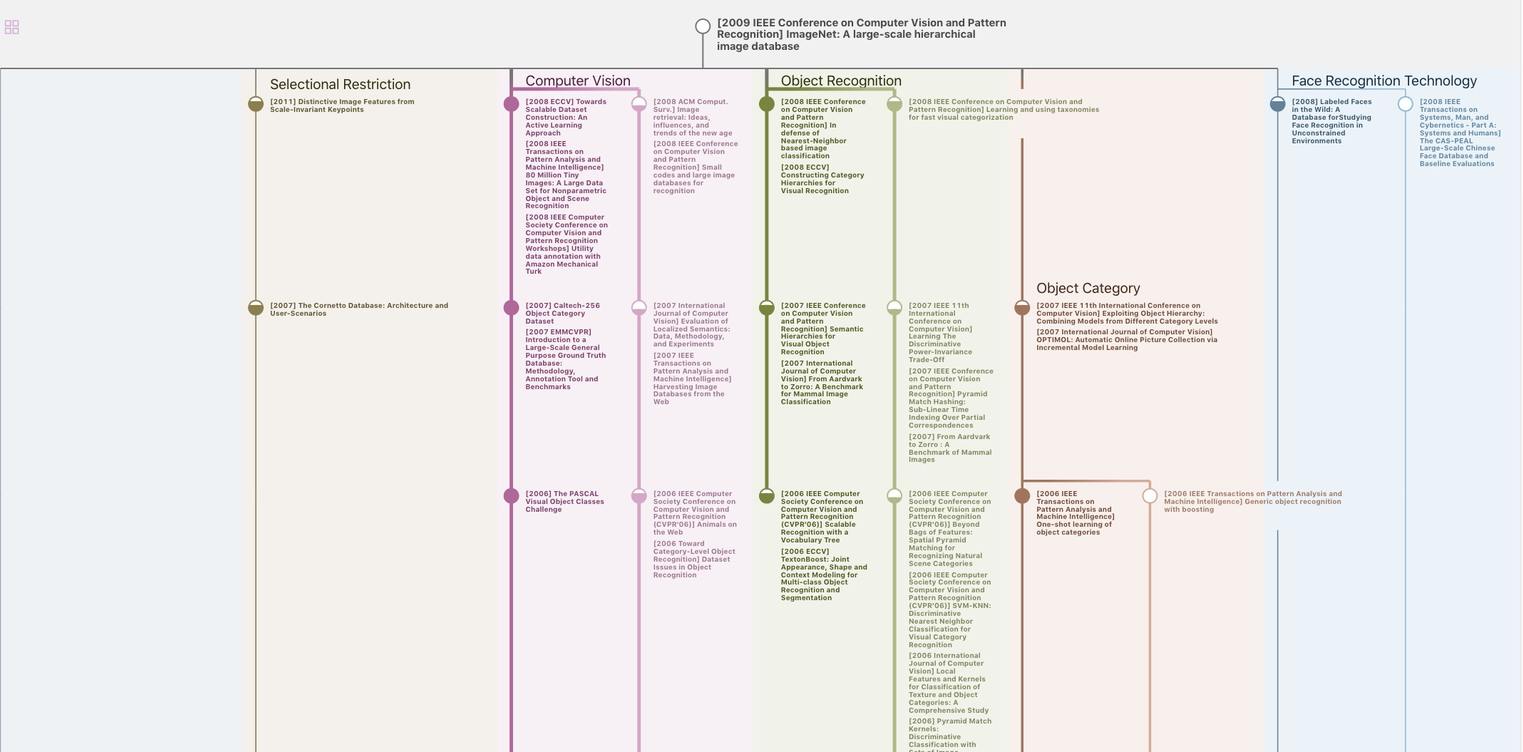
生成溯源树,研究论文发展脉络
Chat Paper
正在生成论文摘要