3d Object Representation Learning: A Set-To-Set Matching Perspective
IEEE TRANSACTIONS ON IMAGE PROCESSING(2021)
摘要
In this paper, we tackle the 3D object representation learning from the perspective of set-to-set matching. Given two 3D objects, calculating their similarity is formulated as the problem of set-to-set similarity measurement between two set of local patches. As local convolutional features from convolutional feature maps are natural representations of local patches, the set-to-set matching between sets of local patches is further converted into a local features pooling problem. To highlight good matchings and suppress the bad ones, we exploit two pooling methods: 1) bilinear pooling and 2) VLAD pooling. We analyze their effectiveness in enhancing the set-to-set matching and meanwhile establish their connection. Moreover, to balance different components inherent in a bilinear-pooled feature, we propose the harmonized bilinear pooling operation, which follows the spirits of intra-normalization used in VLAD pooling. To achieve an end-to-end trainable framework, we implement the proposed harmonized bilinear pooling and intra-normalized VLAD as two layers to construct two types of neural network, multi-view harmonized bilinear network (MHBN) and multi-view VLAD network (MVLADN). Systematic experiments conducted on two public benchmark datasets demonstrate the efficacy of the proposed MHBN and MVLADN in 3D object recognition.
更多查看译文
关键词
Three-dimensional displays, Kernel, Object recognition, Neural networks, Feature extraction, Two dimensional displays, Convolutional neural networks, 3D object recognition, convolutional neural network, bilinear pooling
AI 理解论文
溯源树
样例
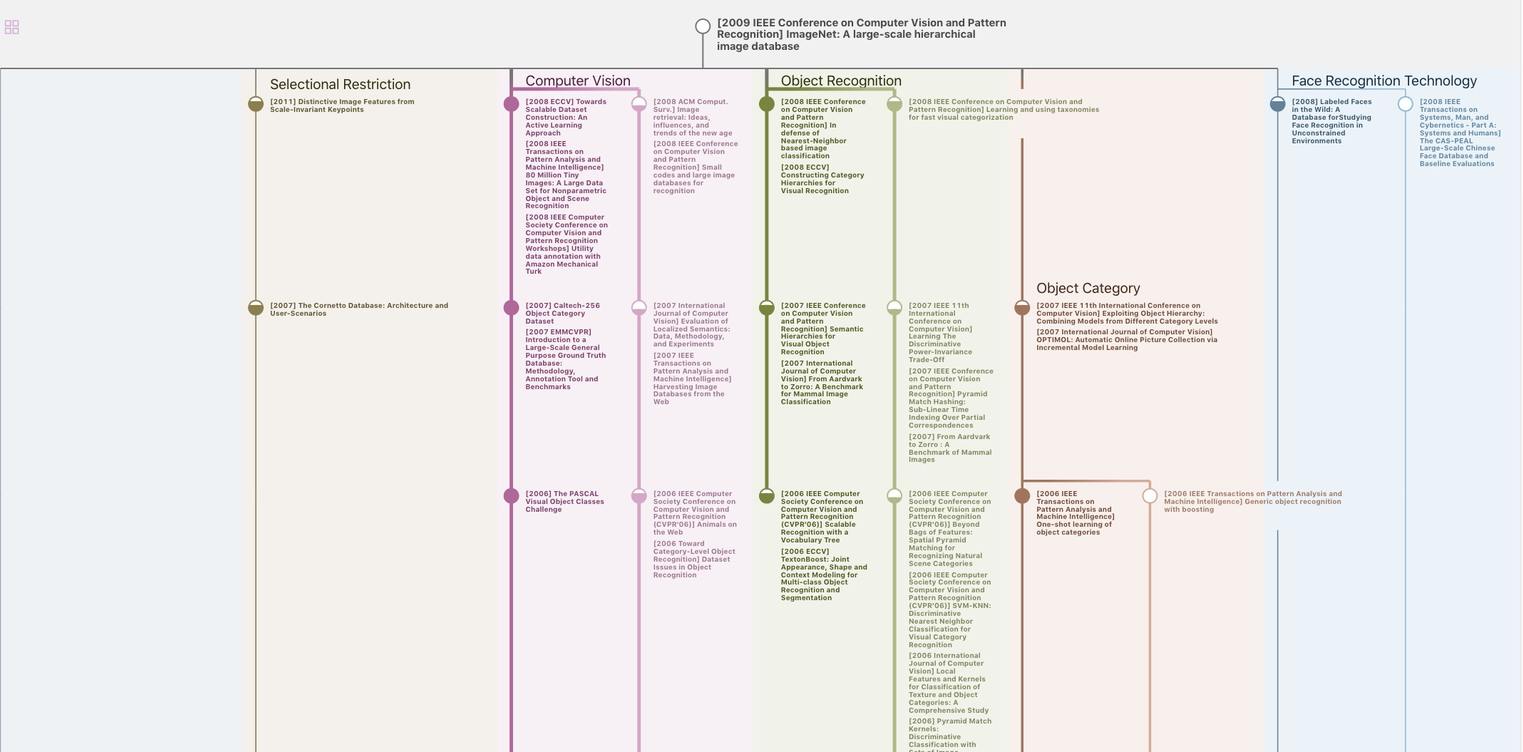
生成溯源树,研究论文发展脉络
Chat Paper
正在生成论文摘要