Revealing Common and Rare Patterns for Peritoneal Dialysis Eligibility Decisions with Association Discovery and Disentanglement
BIBM(2020)
摘要
Peritoneal dialysis (PD) removes waste products from blood when the kidney is malfunctioned. Since there is no clear criterion for PD recommendation for patients with kidney disease, existing machine learning models (ML), which rely on credible decision criterion, are ineffective in making PD eligibility decisions, especially when the correlated traits or indicators (patterns) inherent in the PD data are diverse and subtle. Furthermore, the lack of interpretable transparency in traditional ML also weakens the credibility of the decision they produce. Hence, an in-depth knowledge of the patients’ characteristics is needed to render a clearer picture of the decision-making process and model to detect the rare PD eligibility cases. In this paper, we extend our previous work (Attribute-Value-Association Discovery and Disentanglement (ADD)), to an extended ADD for PD data analysis (PD-ADD) to overcome these problems. We show that PD-ADD is able to discover association patterns of patient profiles and symptoms to reveal PD characteristics and detect eligible rare cases. Experimental results show that PDADD is much superior to existing unsupervised clustering (with accuracy of 89.87% vs 73.37% of K-Means). It also enables straightforward interpretation of the underlying relations of patient characteristics in an unsupervised setting.
更多查看译文
关键词
Clinical data analysis, Peritoneal Dialysis Eligibility, Unsupervised Clustering
AI 理解论文
溯源树
样例
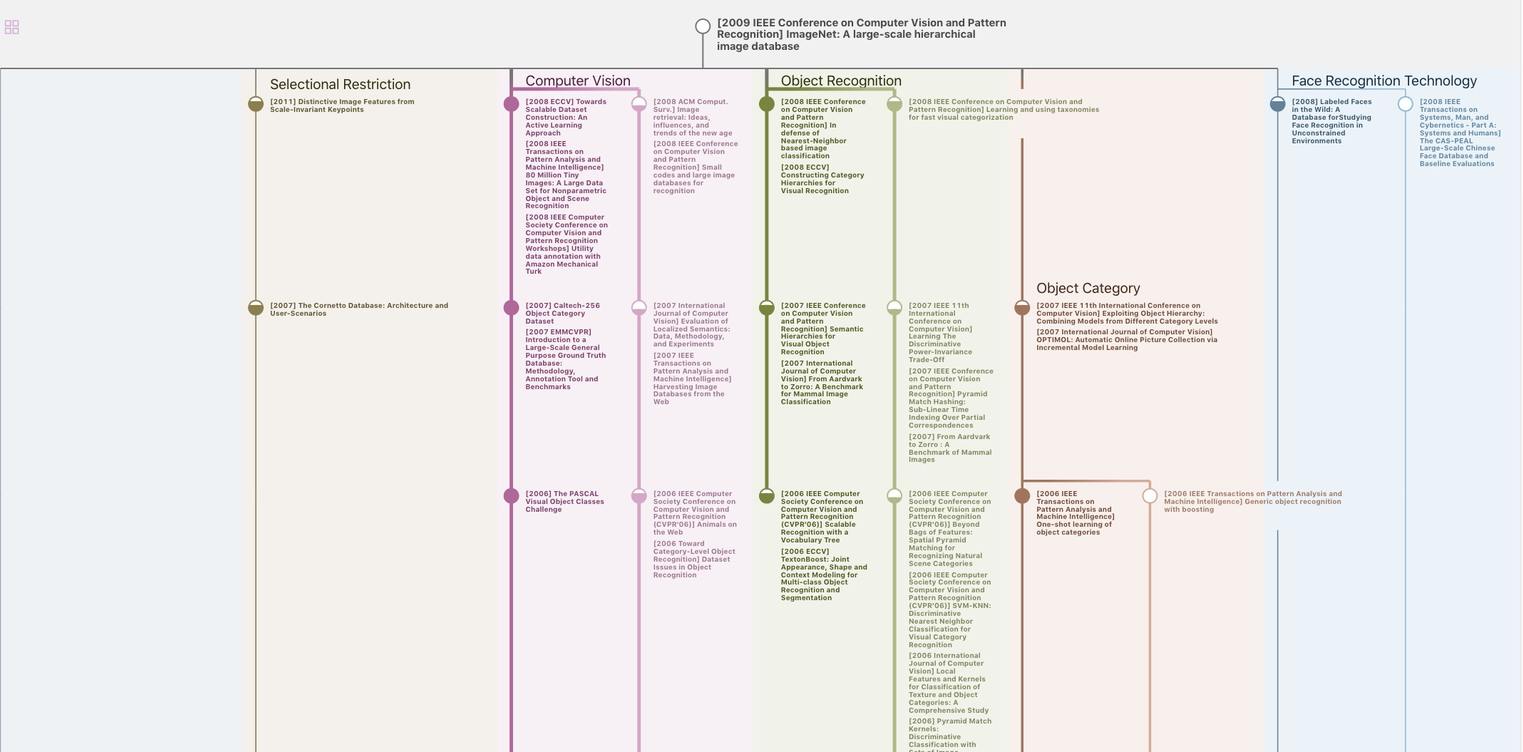
生成溯源树,研究论文发展脉络
Chat Paper
正在生成论文摘要