Tissue of origin classification from single cell mRNA expression by Artificial Neural Networks.
BIBM(2020)
摘要
Single cell transcriptomics (SCT) enables high-throughput measurement of mRNA expression concurrently from tens of thousands of single cells. Gene expression profiles in single cells cover only a small fraction of expressed genes and these data are inherently noisy. We developed a method that utilizes artificial neural networks (ANN) for classification of single cells by their tissue of origin. Data sets representing 10 different organs and tissues from C57BL/6 laboratory mice were standardized and used for training and testing ANN models. Each organ was represented by at least two datasets derived from different mice. We achieved 80% accuracy in 10-class classification. After combining data sets from spleen, bone marrow, and lung into one super-class and mammary tissue and muscle into another, we achieved overall cell classification accuracy of 98% across two tissue super-classes and five organs.
更多查看译文
关键词
classification of single cells,hierarchical classification,single cell gene expression,super-class,supervised machine learning
AI 理解论文
溯源树
样例
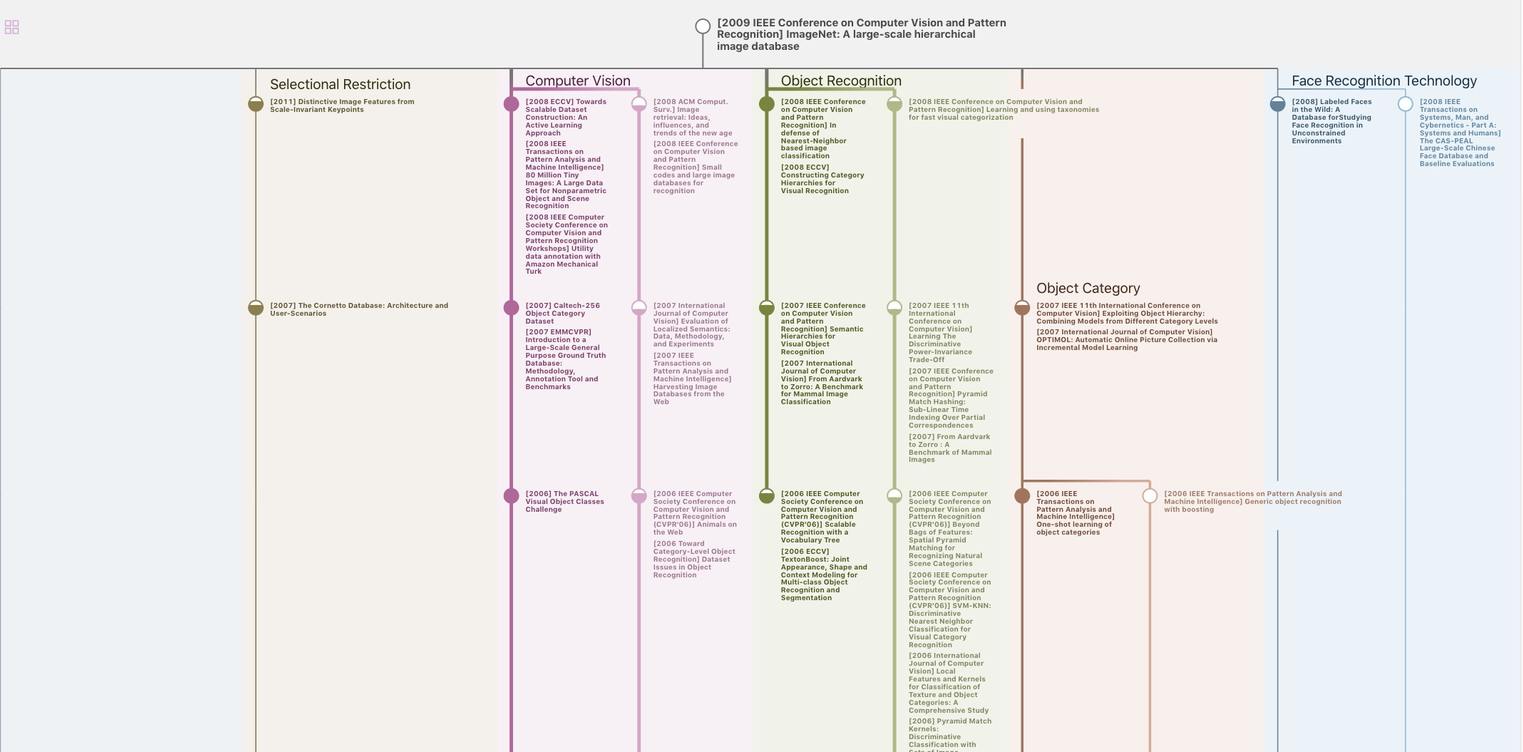
生成溯源树,研究论文发展脉络
Chat Paper
正在生成论文摘要