CNN-DDI - A novel deep learning method for predicting drug-drug interactions.
BIBM(2020)
摘要
Predicting drug-drug interactions (DDIs) is one of the major concerns in patients’ medication, which is crucial for patient safety and public health. Most of studies study whether drugs interact or not. In this study, we focus on 65 categories of drug-drug interaction-associated events and proposed a new method based on convolutional neural network (CNN), named CNN-DDI, for predicting DDIs. First, the categories, targets, pathways and enzymes of drugs were extracted as the features of drugs, which constructed the input of CNN-DDI. Then, these features were as input vectors of our CNN network, and the output is the prediction of drug-drug interaction-associated events’ categories. In the computational experiments, the CNN-DDI method achieves an accuracy rate up to 0.8914, an area under the precision-recall curve up to 0.9322. And the experiments also prove using feature combinations outperforms one feature. Compared with other state-of-the-art methods, the CNN-DDI method has better performance in the superiority and the effectiveness for predicting DDI’s events.
更多查看译文
关键词
Drug-drug interactions, CNN, drug categories, feature combination
AI 理解论文
溯源树
样例
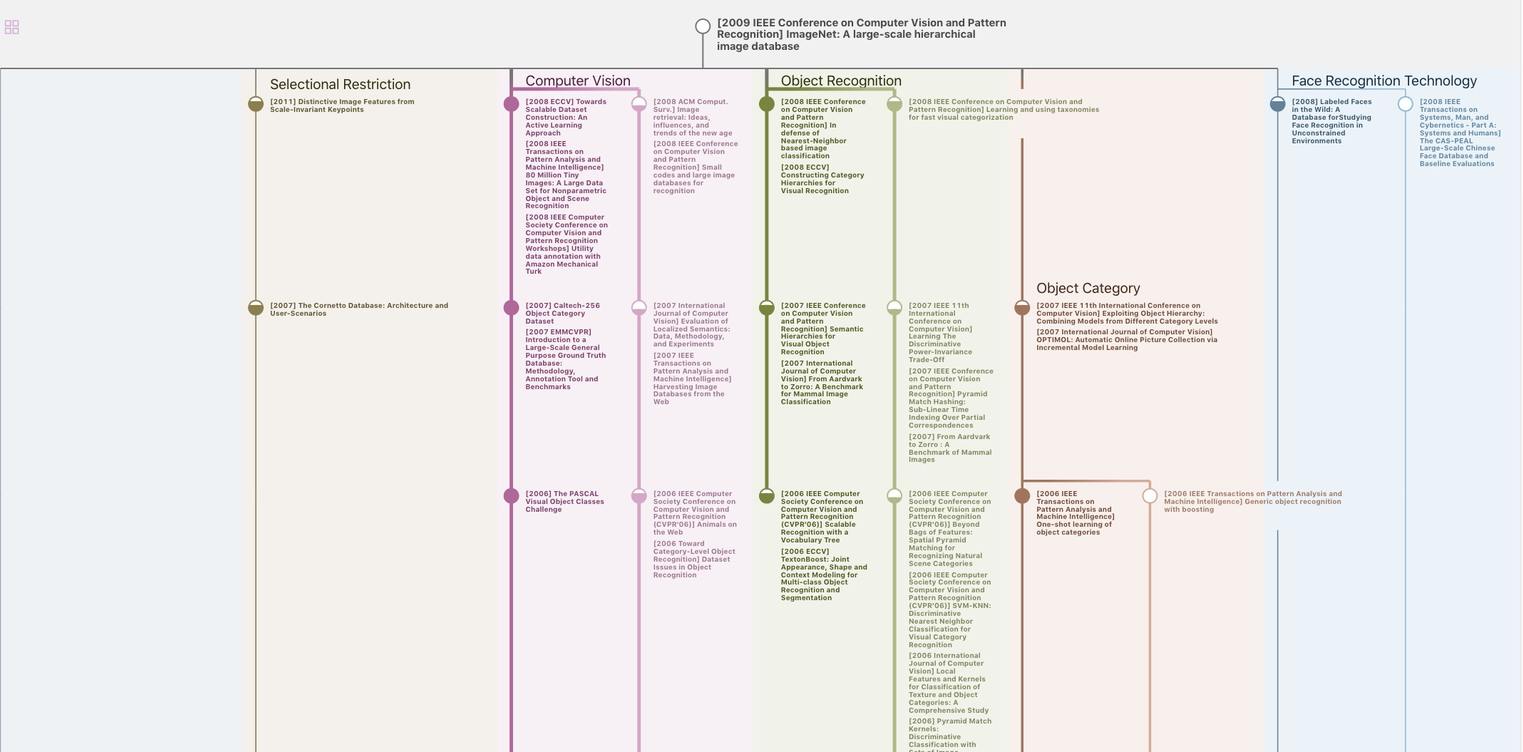
生成溯源树,研究论文发展脉络
Chat Paper
正在生成论文摘要