Pediatric Patient Traumatic Brain Injury Prediction
BIBM(2020)
摘要
Existing Traumatic Brain Injury (TBI) mortality prediction models have not historically accounted for the low mortality rate of pediatric patients, causing class-imbalance problems and undermining classifier prediction performance. We minimize class-imbalance effects by separately implementing case-weighting and subsampling for eight supervised machine learning (ML) techniques, trained and evaluated on pediatric patient National Trauma Data Bank (NTDB) data. The training set consists of pediatric TBI patient records between 2010 and 2014, where records from 2015 are used as the test set. Unweighted models, trained on data modified by Synthetic Minority Over-sampling Technique (SMOTE) and Random Over-sampling Examples (ROSE), included Stacked Autoencoder Deep Neural Network (SAEDNN), K-Nearest Neighbors (KNN), Naive Bayes Classifier (NB), and Support Vector Machine (SVM). Case-weighted models, trained on standardized data, included Artificial Neural Network (ANN), Extreme Gradient Boosting (XGB), C5.0 Decision Tree (C5.0), and Logistic Regression (LR). Preliminarily, XGB and C5.0 were best able to distinguish between classes. Models trained on SMOTE data maximized metrics accounting for class-imbalance and models trained on ROSE data tended to generally underperform.
更多查看译文
关键词
Pediatric,TBI,Imbalanced,Mortality Prediction,Machine Learning
AI 理解论文
溯源树
样例
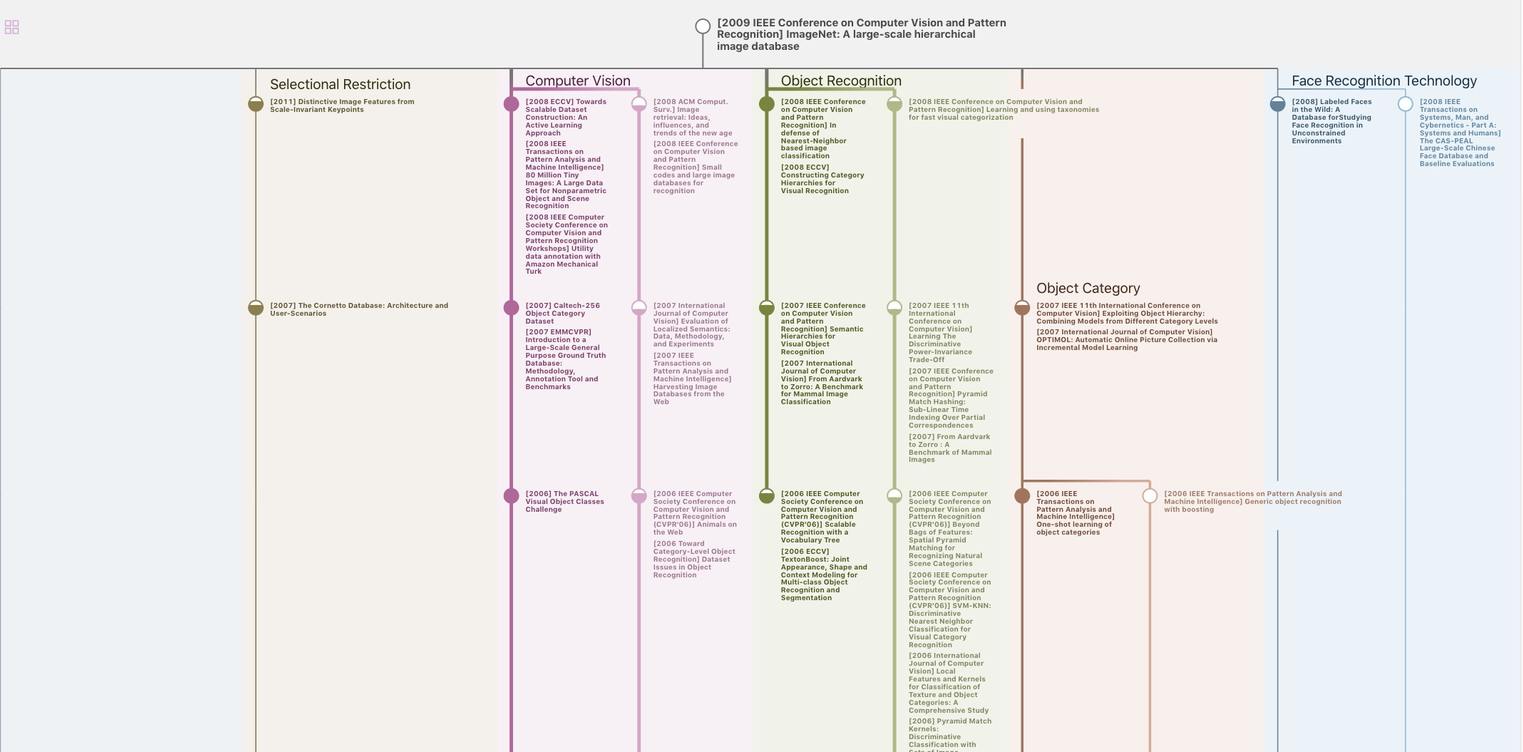
生成溯源树,研究论文发展脉络
Chat Paper
正在生成论文摘要