To "See" is to Stereotype: Image Tagging Algorithms, Gender Recognition, and the Accuracy-Fairness Trade-off
Proceedings of the ACM on Human-Computer Interaction(2020)
摘要
AbstractMachine-learned computer vision algorithms for tagging images are increasingly used by developers and researchers, having become popularized as easy-to-use "cognitive services." Yet these tools struggle with gender recognition, particularly when processing images of women, people of color and non-binary individuals. Socio-technical researchers have cited data bias as a key problem; training datasets often over-represent images of people and contexts that convey social stereotypes. The social psychology literature explains that people learn social stereotypes, in part, by observing others in particular roles and contexts, and can inadvertently learn to associate gender with scenes, occupations and activities. Thus, we study the extent to which image tagging algorithms mimic this phenomenon. We design a controlled experiment, to examine the interdependence between algorithmic recognition of context and the depicted person's gender. In the spirit of auditing to understand machine behaviors, we create a highly controlled dataset of people images, imposed on gender-stereotyped backgrounds. Our methodology is reproducible and our code publicly available. Evaluating five proprietary algorithms, we find that in three, gender inference is hindered when a background is introduced. Of the two that "see" both backgrounds and gender, it is the one whose output is most consistent with human stereotyping processes that is superior in recognizing gender. We discuss the accuracy--fairness trade-off, as well as the importance of auditing black boxes in better understanding this double-edged sword.
更多查看译文
关键词
bias detection,gender,image tagging algorithms,social stereotypes
AI 理解论文
溯源树
样例
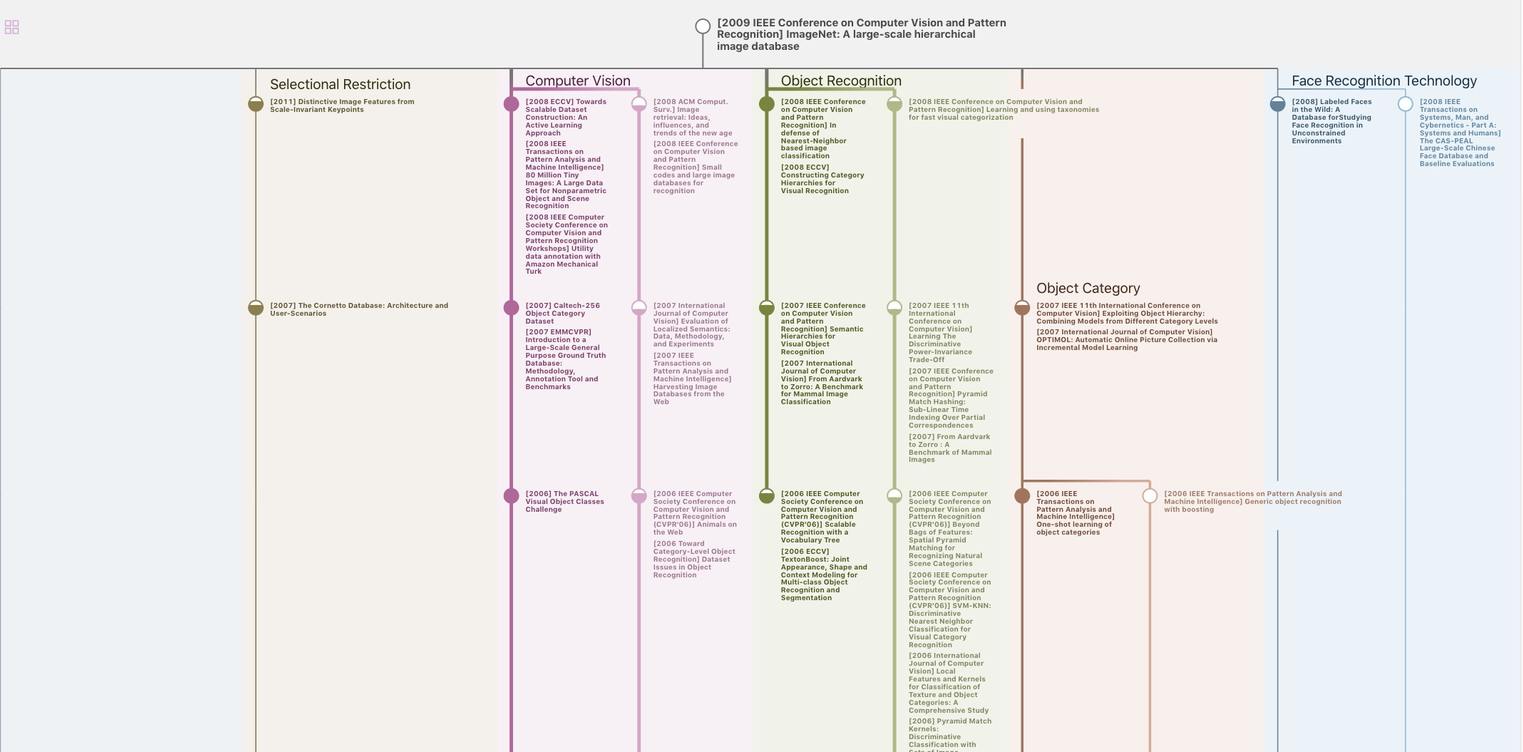
生成溯源树,研究论文发展脉络
Chat Paper
正在生成论文摘要