LoRaDRL: Deep Reinforcement Learning Based Adaptive PHY Layer Transmission Parameters Selection for LoRaWAN
2020 IEEE 45th Conference on Local Computer Networks (LCN)(2020)
摘要
The performance of densely-deployed low-power wide-area networks (LPWANs) can significantly deteriorate due to packets collisions, and one of the main reasons for that is the rule-based PHY layer transmission parameters assignment algorithms. LoRaWAN is a leading LPWAN technology where LoRa serves as the physical layer. Here, we propose and evaluate a deep reinforcement learning (DRL)-based PHY layer transmission parameter assignment algorithm for LoRaWAN. Our algorithm ensures fewer collisions and better network performance compared to the existing state-of-the-art PHY layer transmission parameter assignment algorithms for LoRaWAN. Our algorithm outperforms the state of the art learning-based technique achieving up to 500% improvement of PDR in some cases.
更多查看译文
关键词
state-of-the-art PHY layer transmission parameter assignment algorithms,learning-based technique,network performance,deep reinforcement learning-based PHY layer transmission parameter assignment algorithm,physical layer,leading LPWAN technology,rule-based PHY layer transmission parameters assignment algorithms,densely-deployed low-power wide-area networks,LoRaWAN,adaptive PHY layer transmission parameters selection
AI 理解论文
溯源树
样例
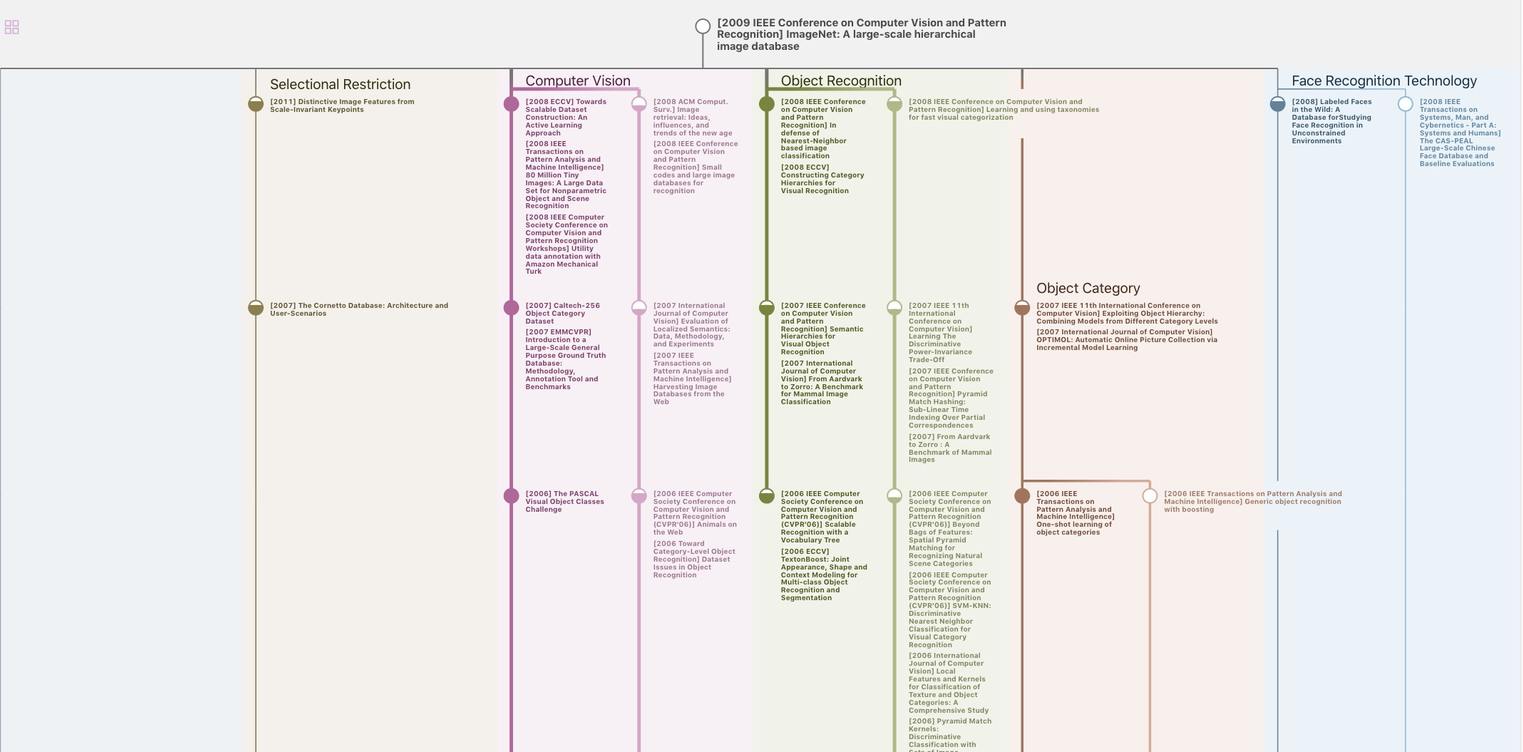
生成溯源树,研究论文发展脉络
Chat Paper
正在生成论文摘要