A Unified Algorithmic Framework for Distributed Composite Optimization.
CDC(2020)
摘要
We study distributed composite optimization over networks: agents minimize a sum of smooth (strongly) convex functions-the agents' sum-utility-plus a nonsmooth (extended-valued) convex one. We propose a general unified algorithmic framework for such a class of problems, whose unified convergence analysis leverages the theory of operator splitting. Distinguishing features of our scheme are: (i) When the agents' functions are strongly convex, the algorithm converges at a linear rate, whose dependence on the agents' functions and network topology is decoupled, matching the typical rates of centralized optimization; the rate expression improves on existing results; (ii) When the objective function is convex (but not strongly convex), similar separation as in (i) is established for the coefficient of the proved sublinear rate; and (iii) A byproduct of our analysis is a tuning recommendation for several existing (non-accelerated) distributed algorithms yielding the fastest provable (worst-case) convergence rate. This is the first time that a general distributed algorithmic framework applicable to composite optimization enjoys all such properties.
更多查看译文
关键词
smooth convex functions,general distributed algorithmic framework,centralized optimization,network topology,convergence analysis,general unified algorithmic framework,nonsmooth convex,sum-utility-plus,distributed composite optimization
AI 理解论文
溯源树
样例
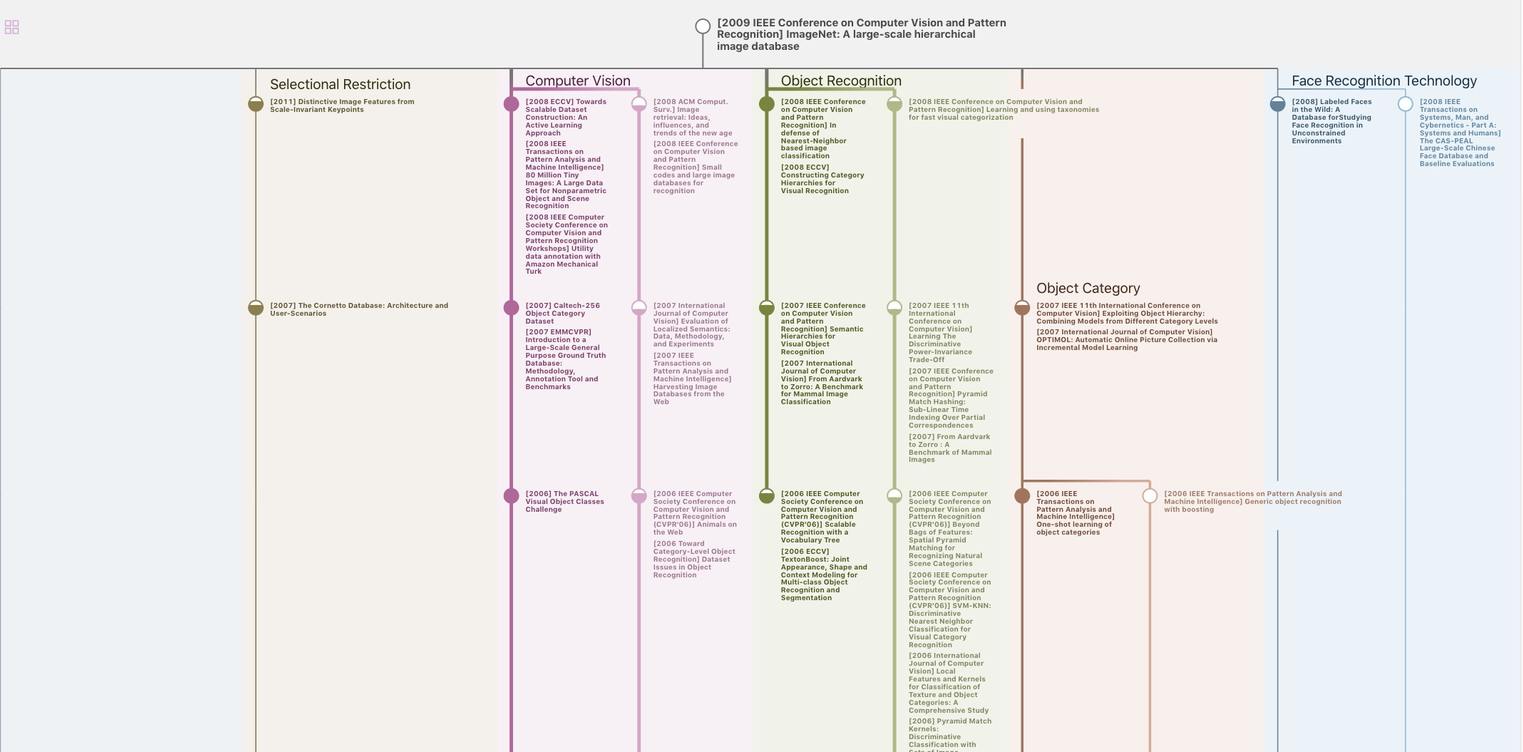
生成溯源树,研究论文发展脉络
Chat Paper
正在生成论文摘要