Improving type information inferred by decompilers with supervised machine learning
arxiv(2021)
摘要
In software reverse engineering, decompilation is the process of recovering source code from binary files. Decompilers are used when it is necessary to understand or analyze software for which the source code is not available. Although existing decompilers commonly obtain source code with the same behavior as the binaries, that source code is usually hard to interpret and certainly differs from the original code written by the programmer. Massive codebases could be used to build supervised machine learning models aimed at improving existing decompilers. In this article, we build different classification models capable of inferring the high-level type returned by functions, with significantly higher accuracy than existing decompilers. We automatically instrument C source code to allow the association of binary patterns with their corresponding high-level constructs. A dataset is created with a collection of real open-source applications plus a huge number of synthetic programs. Our system is able to predict function return types with a 79.1% F1-measure, whereas the best decompiler obtains a 30% F1-measure. Moreover, we document the binary patterns used by our classifier to allow their addition in the implementation of existing decompilers.
更多查看译文
关键词
type information,decompilers,machine learning
AI 理解论文
溯源树
样例
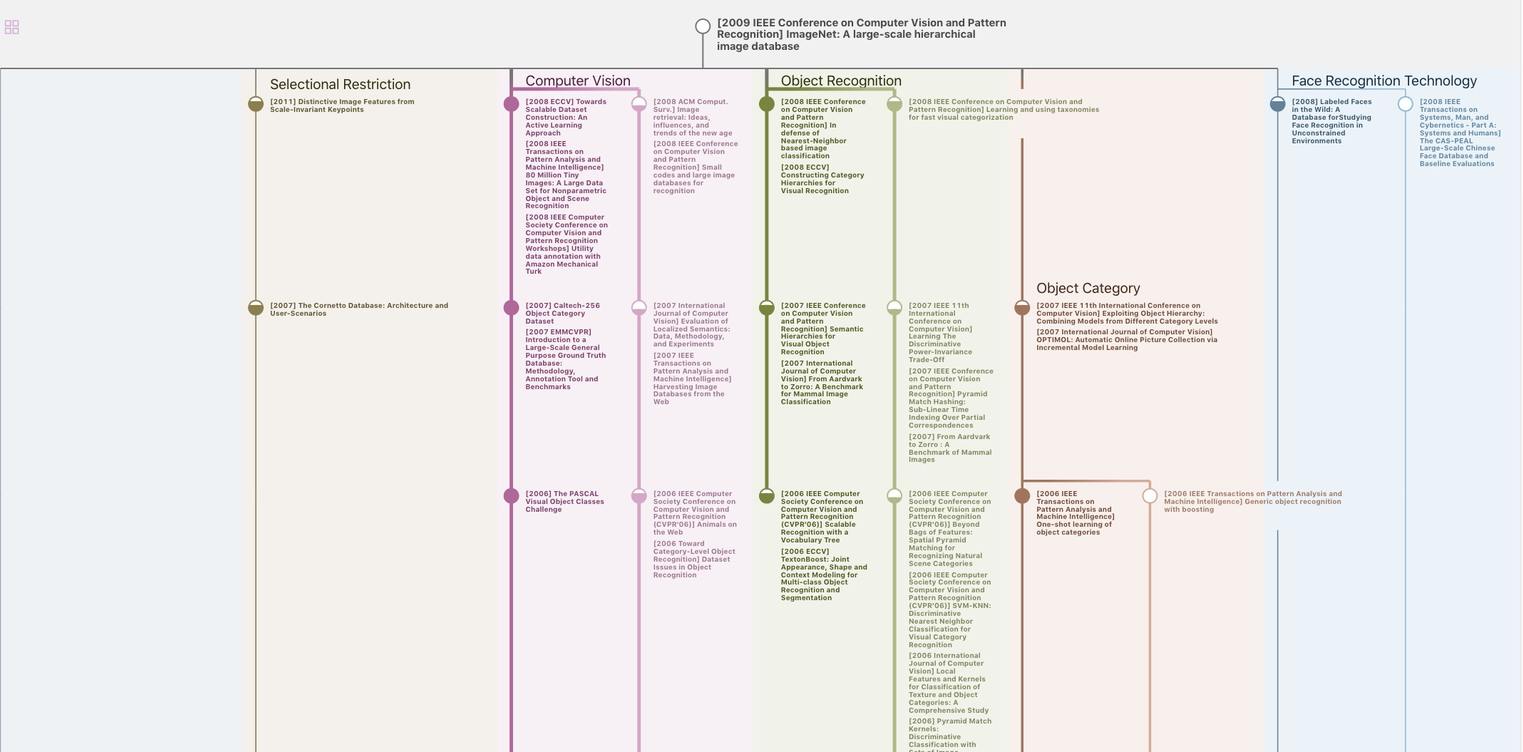
生成溯源树,研究论文发展脉络
Chat Paper
正在生成论文摘要