Clairvoyance: A Pipeline Toolkit for Medical Time Series
ICLR(2021)
摘要
Time-series learning is the bread and butter of data-driven *clinical decision support*, and the recent explosion in ML research has demonstrated great potential in various healthcare settings. At the same time, medical time-series problems in the wild are challenging due to their highly *composite* nature: They entail design choices and interactions among components that preprocess data, impute missing values, select features, issue predictions, estimate uncertainty, and interpret models. Despite exponential growth in electronic patient data, there is a remarkable gap between the potential and realized utilization of ML for clinical research and decision support. In particular, orchestrating a real-world project lifecycle poses challenges in engineering (i.e. hard to build), evaluation (i.e. hard to assess), and efficiency (i.e. hard to optimize). Designed to address these issues simultaneously, Clairvoyance proposes a unified, end-to-end, autoML-friendly pipeline that serves as a (i) software toolkit, (ii) empirical standard, and (iii) interface for optimization. Our ultimate goal lies in facilitating transparent and reproducible experimentation with complex inference workflows, providing integrated pathways for (1) personalized prediction, (2) treatment-effect estimation, and (3) information acquisition. Through illustrative examples on real-world data in outpatient, general wards, and intensive-care settings, we illustrate the applicability of the pipeline paradigm on core tasks in the healthcare journey. To the best of our knowledge, Clairvoyance is the first to demonstrate viability of a comprehensive and automatable pipeline for clinical time-series ML.
更多查看译文
关键词
clairvoyance,pipeline toolkit,series,time
AI 理解论文
溯源树
样例
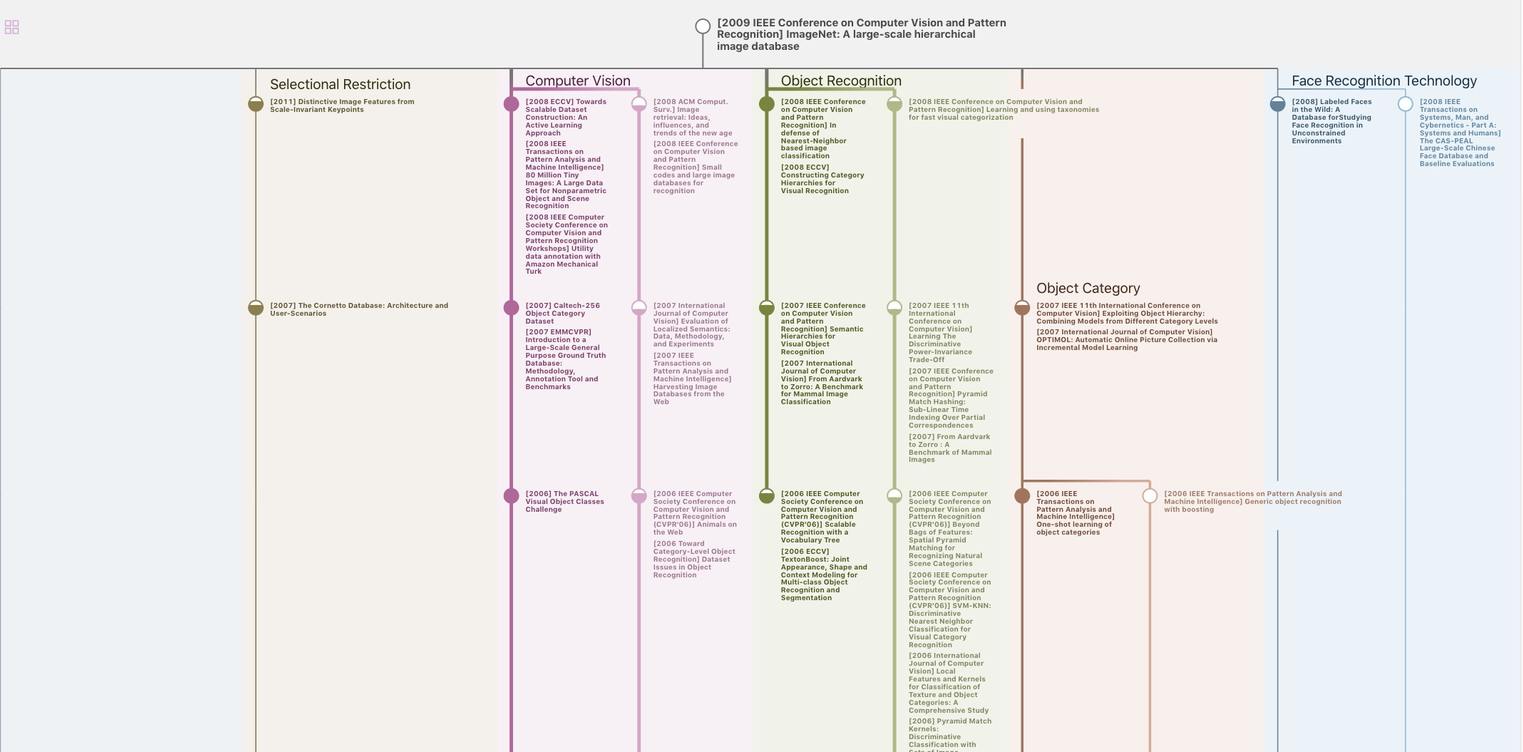
生成溯源树,研究论文发展脉络
Chat Paper
正在生成论文摘要