Development Of A Treatment-Decision Algorithm For Human Immunodeficiency Virus-Uninfected Children Evaluated For Pulmonary Tuberculosis
CLINICAL INFECTIOUS DISEASES(2021)
摘要
Background: Limitations in the sensitivity and accessibility of diagnostic tools for childhood tuberculosis contribute to the substantial gap between estimated cases and cases notified to national tuberculosis programs. Thus, tools to make accurate and rapid clinical diagnoses are necessary to initiate antituberculosis treatment in more children.Methods: We analyzed data from a prospective cohort of children <13 years old being routinely evaluated for pulmonary tuberculosis in Cape Town, South Africa, from March 2012 to November 2017. We developed a regression model to describe the contributions of baseline clinical evaluation to the diagnosis of tuberculosis using standardized, retrospective case definitions. We included baseline chest radiographic and Xpert MTB/RIF assay results to the model to develop an algorithm with >= 90% sensitivity in predicting tuberculosis.Results: Data from 478 children being evaluated for pulmonary tuberculosis were analyzed (median age, 16.2 months; interquartile range, 9.8-30.9 months); 242 (50.6%) were retrospectively classified with tuberculosis, bacteriologically confirmed in 104 (43.0%). The area under the receiver operating characteristic curve for the final model was 0.87. Clinical evidence identified 71.4% of all tuberculosis cases in this cohort, and inclusion of baseline chest radiographic results increased the proportion to 89.3%. The algorithm was 90.1% sensitive and 52.1% specific, and maintained a sensitivity of >90% among children <2 years old or with low weight for age.Conclusions: Clinical evidence alone was sufficient to make most clinical antituberculosis treatment decisions. The use of evidence-based algorithms may improve decentralized, rapid treatment initiation, reducing the global burden of childhood mortality.
更多查看译文
关键词
Diagnostic algorithm, diagnostic system, pediatric, clinical evidence, Diagnosis, clinical decision-making
AI 理解论文
溯源树
样例
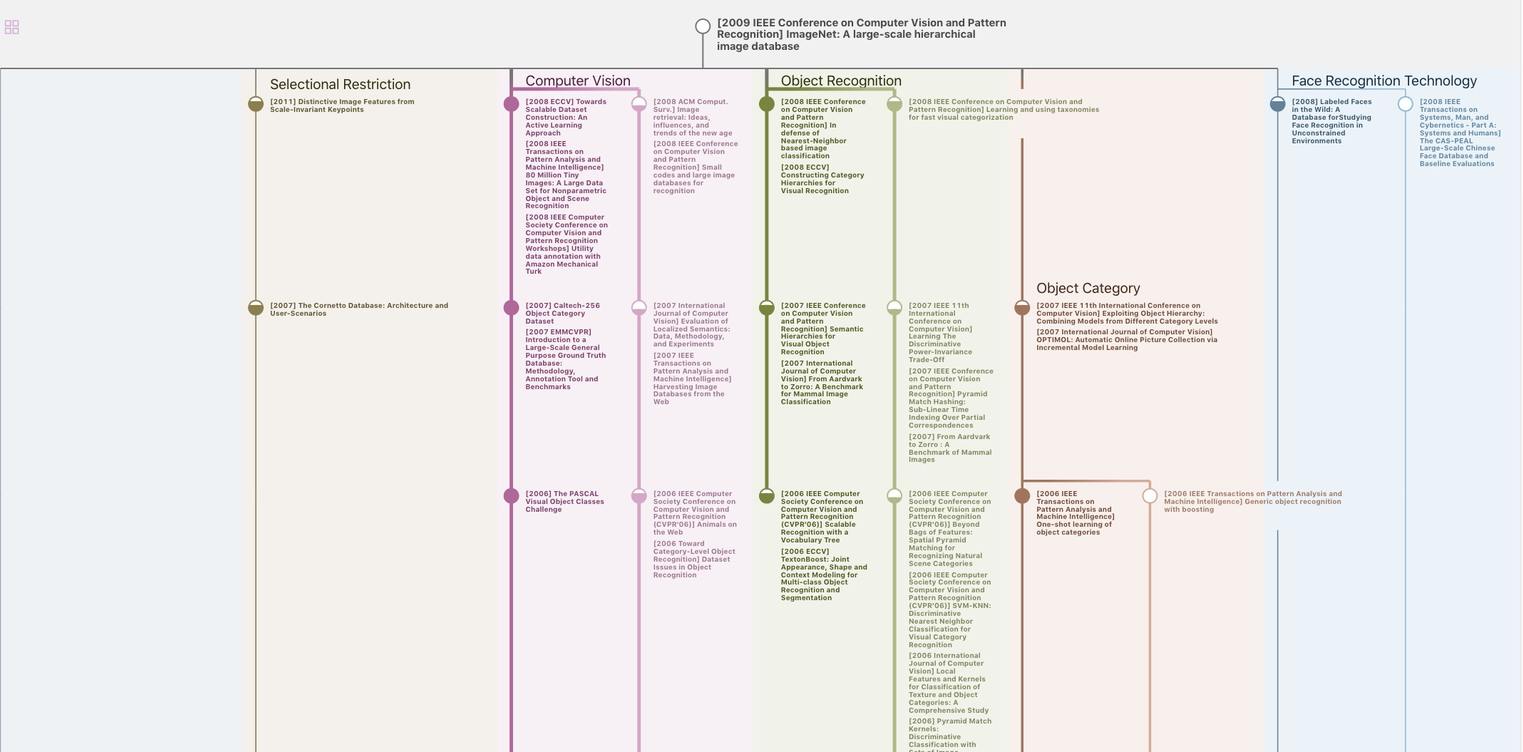
生成溯源树,研究论文发展脉络
Chat Paper
正在生成论文摘要