Inferring The Driver'S Lane Change Intention Through Lidar-Based Environment Analysis Using Convolutional Neural Networks
SENSORS(2021)
摘要
Most of the tactic manoeuvres during driving require a certain understanding of the surrounding environment from which to devise our future behaviour. In this paper, a Convolutional Neural Network (CNN) approach is used to model the lane change behaviour to identify when a driver is going to perform this manoeuvre. To that end, a slightly modified CNN architecture adapted to both spatial (i.e., surrounding environment) and non-spatial (i.e., rest of variables such as relative speed to the front vehicle) input variables. Anticipating a driver's lane change intention means it is possible to use this information as a new source of data in wide range of different scenarios. One example of such scenarios might be the decision making process support for human drivers through Advanced Driver Assistance Systems (ADAS) fed with the data of the surrounding cars in an inter-vehicular network. Another example might even be its use in autonomous vehicles by using the data of a specific driver profile to make automated driving more human-like. Several CNN architectures have been tested on a simulation environment to assess their performance. Results show that the selected architecture provides a higher degree of accuracy than random guessing (i.e., assigning a class randomly for each observation in the data set), and it can capture subtle differences in behaviour between different driving profiles.
更多查看译文
关键词
Convolutional Neural Networks, Intelligent Transportation Systems, lane change, ADAS, driver’, s behaviour, autonomous driving
AI 理解论文
溯源树
样例
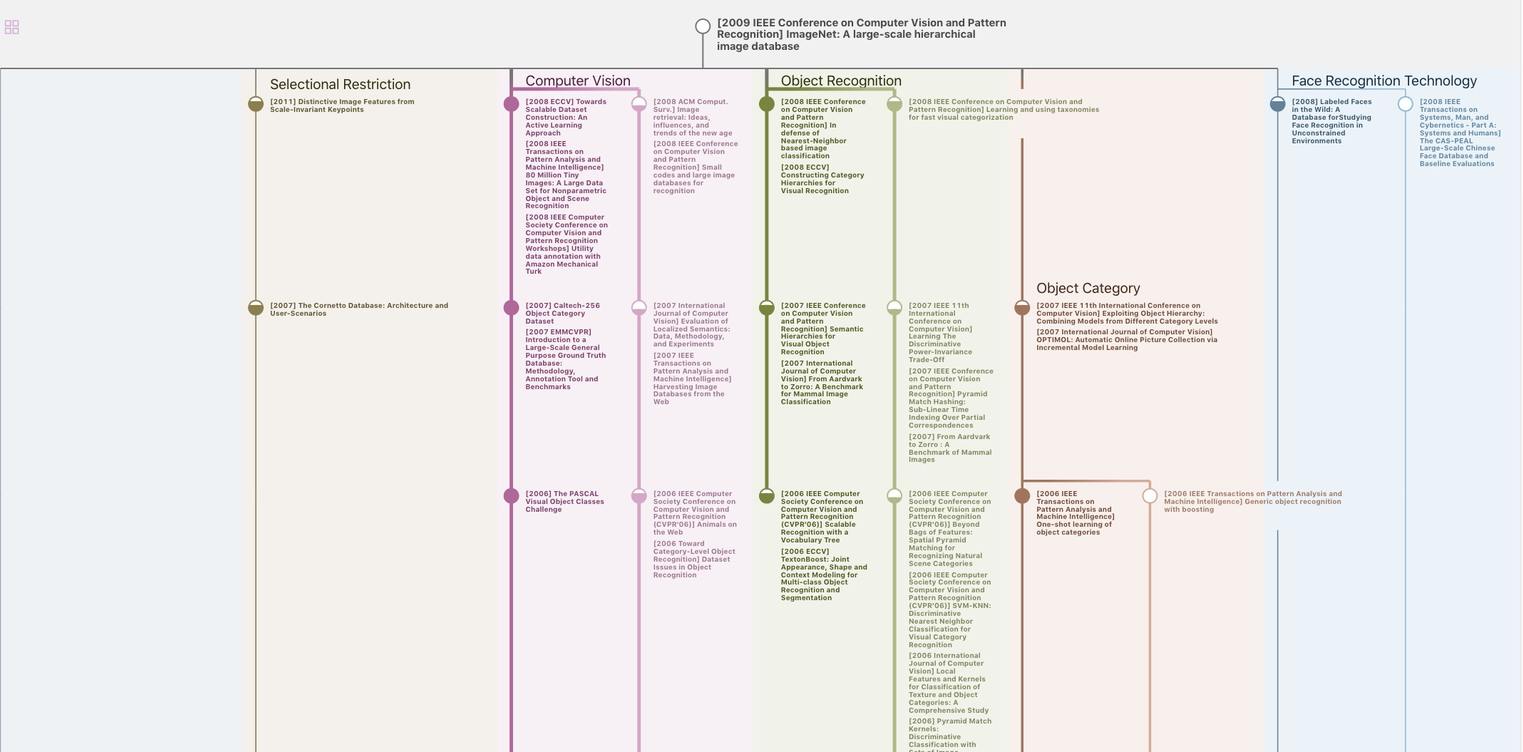
生成溯源树,研究论文发展脉络
Chat Paper
正在生成论文摘要