Prediction Error Reduction of Neural Networks for Car-Following Using Multi-Step Training.
ITSC(2020)
摘要
Predicting the surrounding vehicles' behavior is an important requirement for automated driving as it enables estimating others' reactions to the own behavior during planning as well as the identification of critical situations. This work proposes a recursive multi-step training scheme for neural networks that predict other vehicles' positions in a highway car-following scenario. We implement a neural network and compare the proposed approach to the commonly used single-step training as well as parametric models. For this, the Intelligent Driver Model (IDM) and its derivatives have been calibrated using the same approach. Evaluation is performed on 10 hours of real-world car-following situations, extracted from the extensive HighD dataset. Given equal inputs, we show that a minimal neural network with two layers composed of three neurons each surpasses the prediction performance of both the parametric prediction models and the network trained with the standard single-step approach.
更多查看译文
关键词
recursive multistep training,HighD dataset,neural networks,surrounding vehicle behavior prediction,standard single-step approach,parametric prediction models,intelligent driver model,single-step training,highway car-following scenario,automated driving,prediction error reduction
AI 理解论文
溯源树
样例
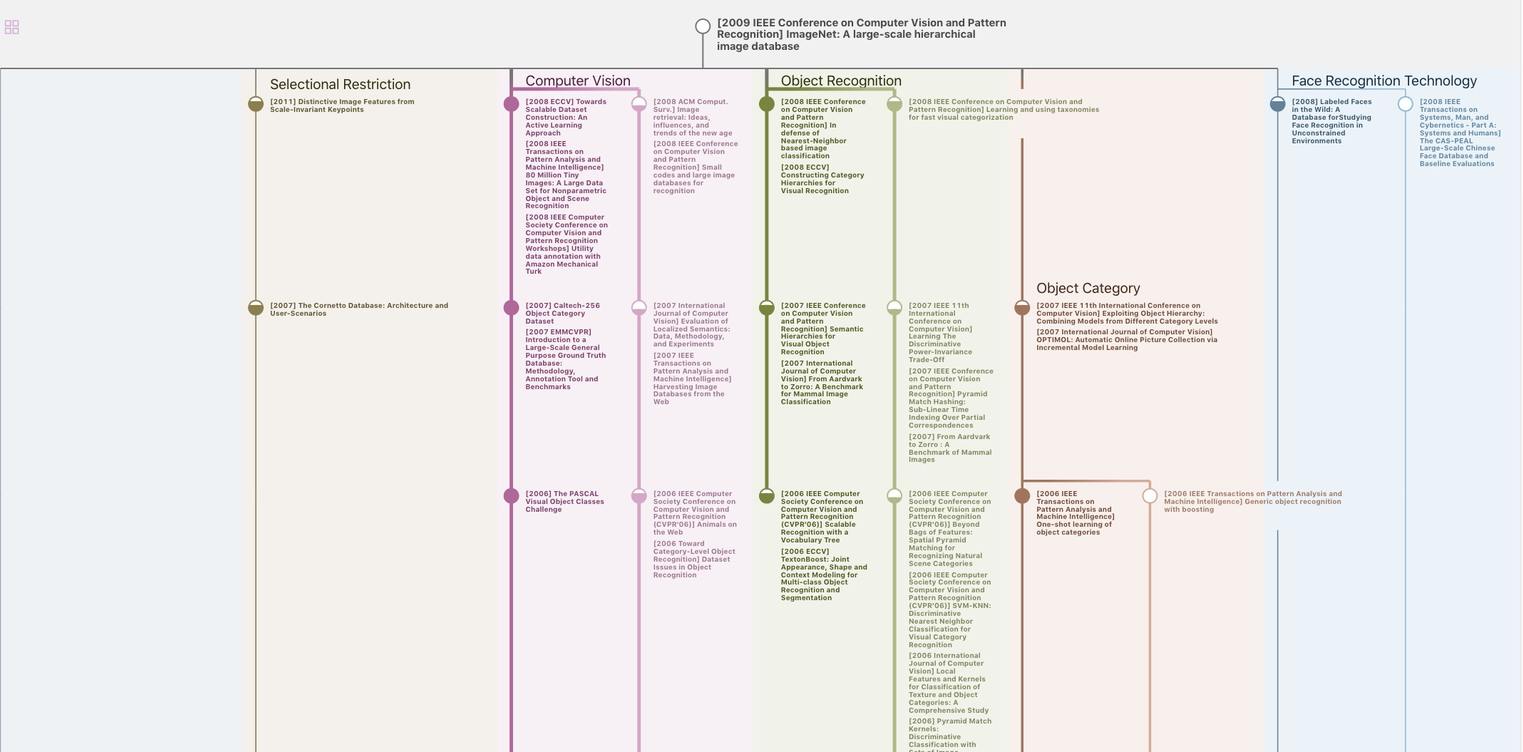
生成溯源树,研究论文发展脉络
Chat Paper
正在生成论文摘要