Unsupervised Evaluation of Lidar Domain Adaptation.
ITSC(2020)
摘要
In this work, we investigate the potential of latent representations generated by Variational Autoencoders (VAE) to analyze and distinguish between real and synthetic data. Although the details of the domain adaptation task are not the focus of this work, we use the example of simulated lidar data adapted by a generative model to match real lidar data. To assess the resulting adapted data, we evaluate the potential of latent representations learned by a VAE. During training, the VAE aims to reduce the input data to a fixed-dimensional feature vector, while also enforcing stochastic independence between the latent variables. These properties can be used to define pseudometrics to make statements about generative models that perform domain adaptation tasks. The variational autoencoder is trained on real target data only and is subsequently used to generate distributions of feature vectors for data coming from different data sources such as simulations or the output of Generative Adversarial Networks.
更多查看译文
关键词
unsupervised evaluation,lidar domain adaptation,latent representations,Variational Autoencoders,VAE,synthetic data,domain adaptation task,simulated lidar data,generative model,resulting adapted data,fixed-dimensional feature vector,latent variables,variational autoencoder,Generative Adversarial Networks
AI 理解论文
溯源树
样例
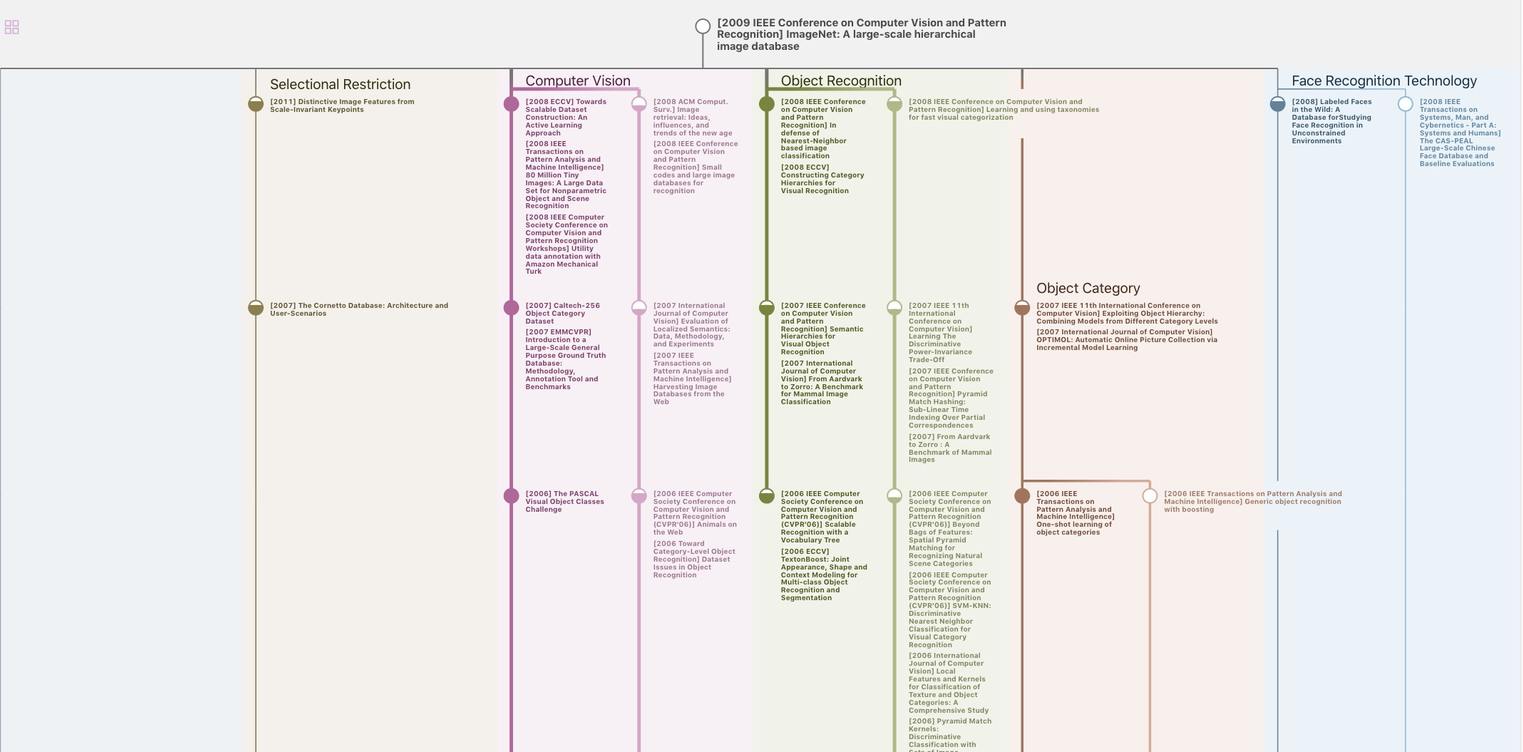
生成溯源树,研究论文发展脉络
Chat Paper
正在生成论文摘要