Model Agnostic Information Biasing for VQA
CODS-COMAD 2021: PROCEEDINGS OF THE 3RD ACM INDIA JOINT INTERNATIONAL CONFERENCE ON DATA SCIENCE & MANAGEMENT OF DATA (8TH ACM IKDD CODS & 26TH COMAD)(2021)
摘要
VQA involves generating information rich features from given images and questions based on them. Here we have explored the use of inducing biases and structuring of multi-modal latent spaces using fusion loss regularization. Our loss based strategy is aimed at making the multi-modal representation of the student branch (Image+Question) to be those like that of the teacher branch (Image+Answer), made with the same model. Our main contribution is that we explore a model agnostic approach based on creation of a homogeneous multimodal latent space for image with question and image with answer's representation. To our best knowledge this is the only work exploring the use of latent space fusion using regularization for VQA.
更多查看译文
关键词
Visual Question Answering, Space Fusion, Model Agnostic
AI 理解论文
溯源树
样例
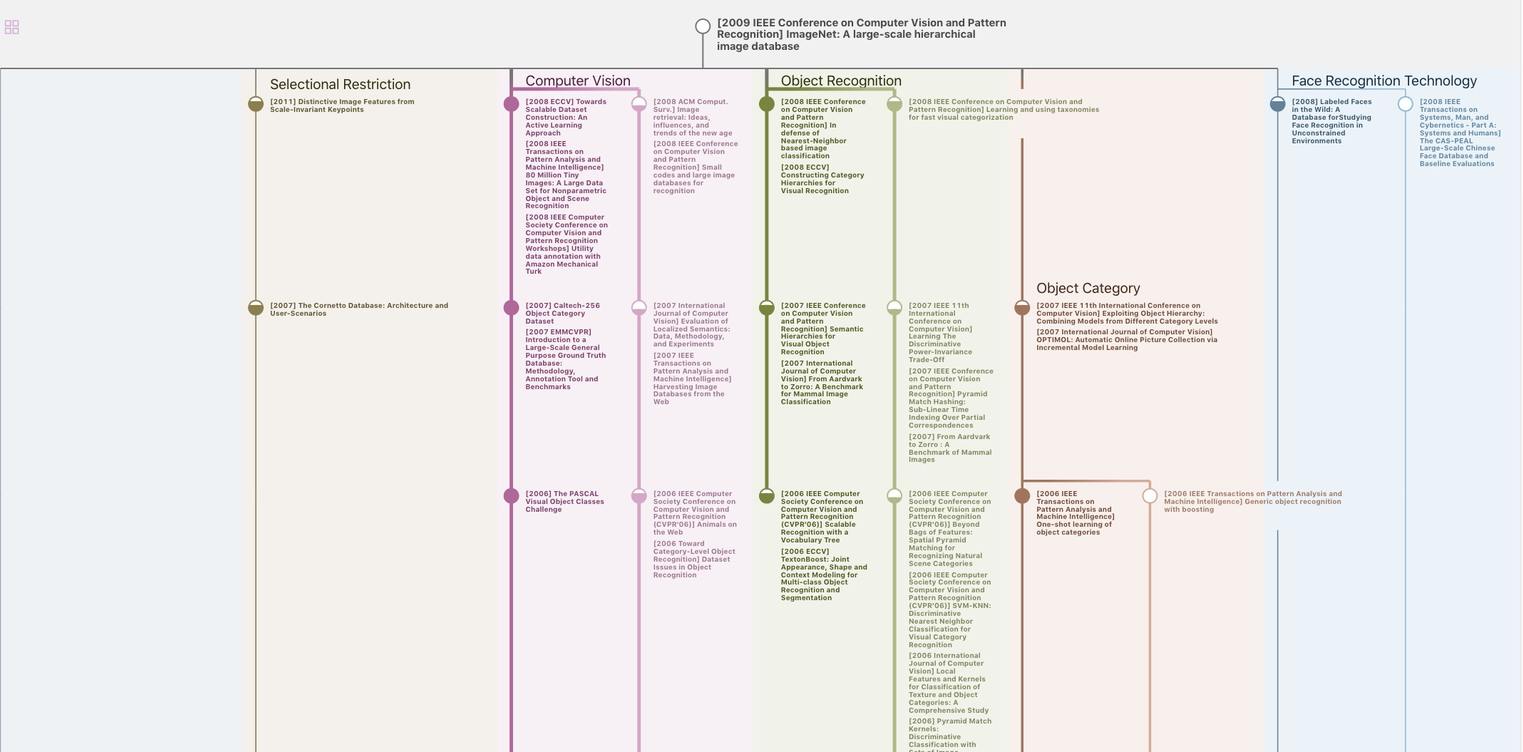
生成溯源树,研究论文发展脉络
Chat Paper
正在生成论文摘要