Learning Knowledge Graph for Target-driven Schema Matching
CODS-COMAD 2021: PROCEEDINGS OF THE 3RD ACM INDIA JOINT INTERNATIONAL CONFERENCE ON DATA SCIENCE & MANAGEMENT OF DATA (8TH ACM IKDD CODS & 26TH COMAD)(2021)
摘要
Though research on schema matching goes back many years, this remains a largely unsolved problem on the ground. The knowledge of concepts, terms and their correspondences useful for matching is implicit in historical matching information. Based on this insight, we propose a probabilistic framework for schema matching based on learning. It updates a knowledge graph of concepts, terms, their relationships and associated probabilities from historical `training' matches, and then uses this knowledge for predicting matches for a `test' source schema. We present a solution based on stochastic EM, where the latent concepts and relationships in historical matches are inferred by sampling during the expectation step, and parameters associated with the knowledge graph are estimated using these inferred variables. Using extensive experiments we show that by making effective use of historical matches, our approach significantly outperforms state-of-the-art matching techniques for a real-world Insurance dataset as well as a benchmark dataset.
更多查看译文
关键词
Schema Matching, Knowledge Graph, Machine Learning
AI 理解论文
溯源树
样例
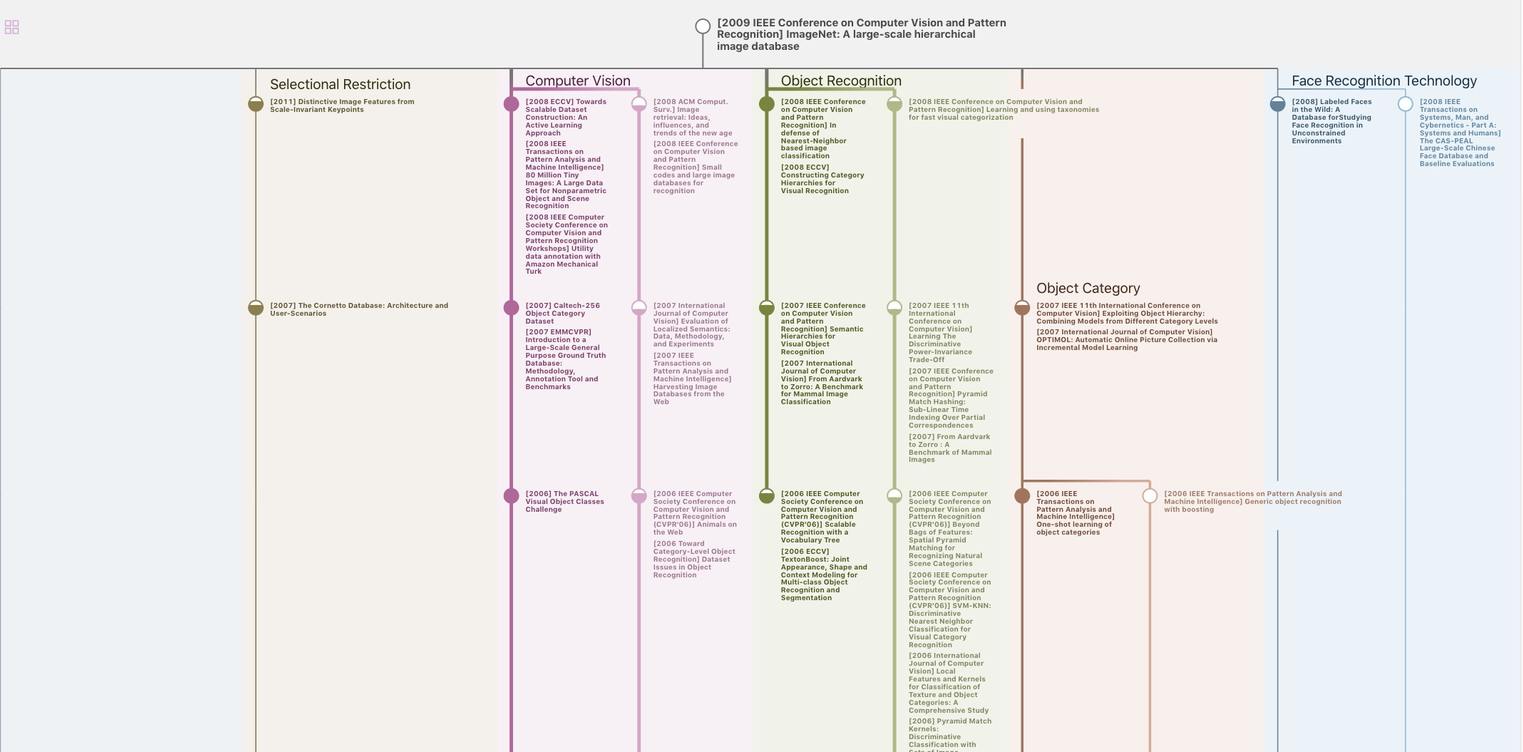
生成溯源树,研究论文发展脉络
Chat Paper
正在生成论文摘要