Autonomous materials synthesis via hierarchical active learning of nonequilibrium phase diagrams
SCIENCE ADVANCES(2021)
摘要
Autonomous experimentation enabled by artificial intelligence offers a new paradigm for accelerating scientific discovery. Nonequilibrium materials synthesis is emblematic of complex, resource-intensive experimentation whose acceleration would be a watershed for materials discovery. We demonstrate accelerated exploration of metastable materials through hierarchical autonomous experimentation governed by the Scientific Autonomous Reasoning Agent (SARA). SARA integrates robotic materials synthesis using lateral gradient laser spike annealing and optical characterization along with a hierarchy of AI methods to map out processing phase diagrams. Efficient exploration of the multidimensional parameter space is achieved with nested active learning cycles built upon advanced machine learning models that incorporate the underlying physics of the experiments and end-to-end uncertainty quantification. We demonstrate SARA's performance by autonomously mapping synthesis phase boundaries for the Bi2O3 system, leading to orders-of-magnitude acceleration in the establishment of a synthesis phase diagram that includes conditions for stabilizing delta-Bi2O3 at room temperature, a critical development for electrochemical technologies.
更多查看译文
AI 理解论文
溯源树
样例
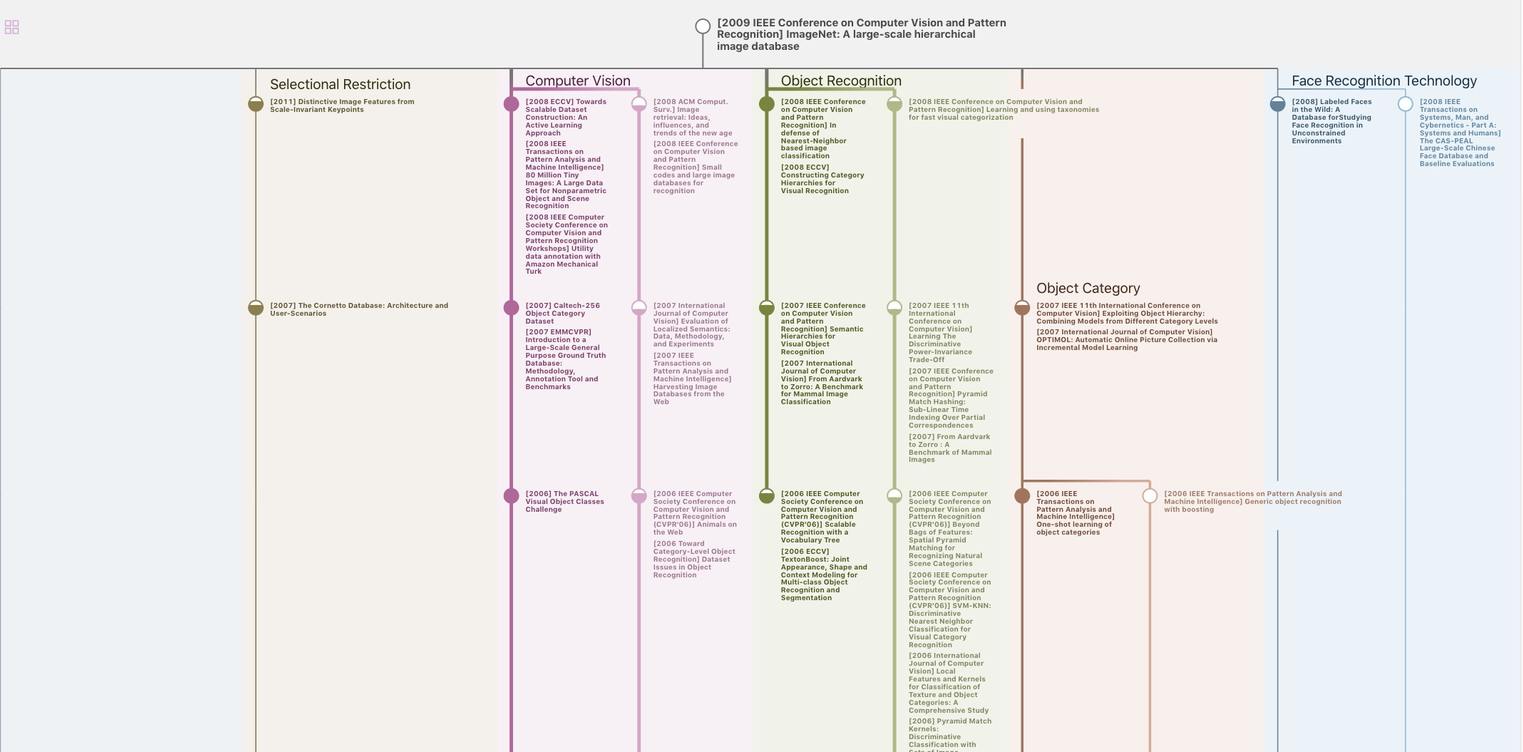
生成溯源树,研究论文发展脉络
Chat Paper
正在生成论文摘要