Fast Privacy-Preserving Text Classification Based on Secure Multiparty Computation
IEEE Transactions on Information Forensics and Security(2022)
摘要
We propose a privacy-preserving Naive Bayes classifier and apply it to the problem of private text classification. In this setting, a party (Alice) holds a text message, while another party (Bob) holds a classifier. At the end of the protocol, Alice will only learn the result of the classifier applied to her text input and Bob learns nothing. Our solution is based on Secure Multiparty Computation (SMC). Our Rust implementation provides a fast and secure solution for the classification of unstructured text. Applying our solution to the case of spam detection (the solution is generic, and can be used in any other scenario in which the Naive Bayes classifier can be employed), we can classify an SMS as spam or ham in less than 340ms in the case where the dictionary size of Bob’s model includes all words (
$n = 5200$
) and Alice’s SMS has at most
$m = 160$
unigrams. In the case with
$n = 369$
and
$m = 8$
(the average of a spam SMS in the database), our solution takes only 21ms.
更多查看译文
关键词
Privacy-preserving classification,secure multiparty computation,Naive Bayes,spam
AI 理解论文
溯源树
样例
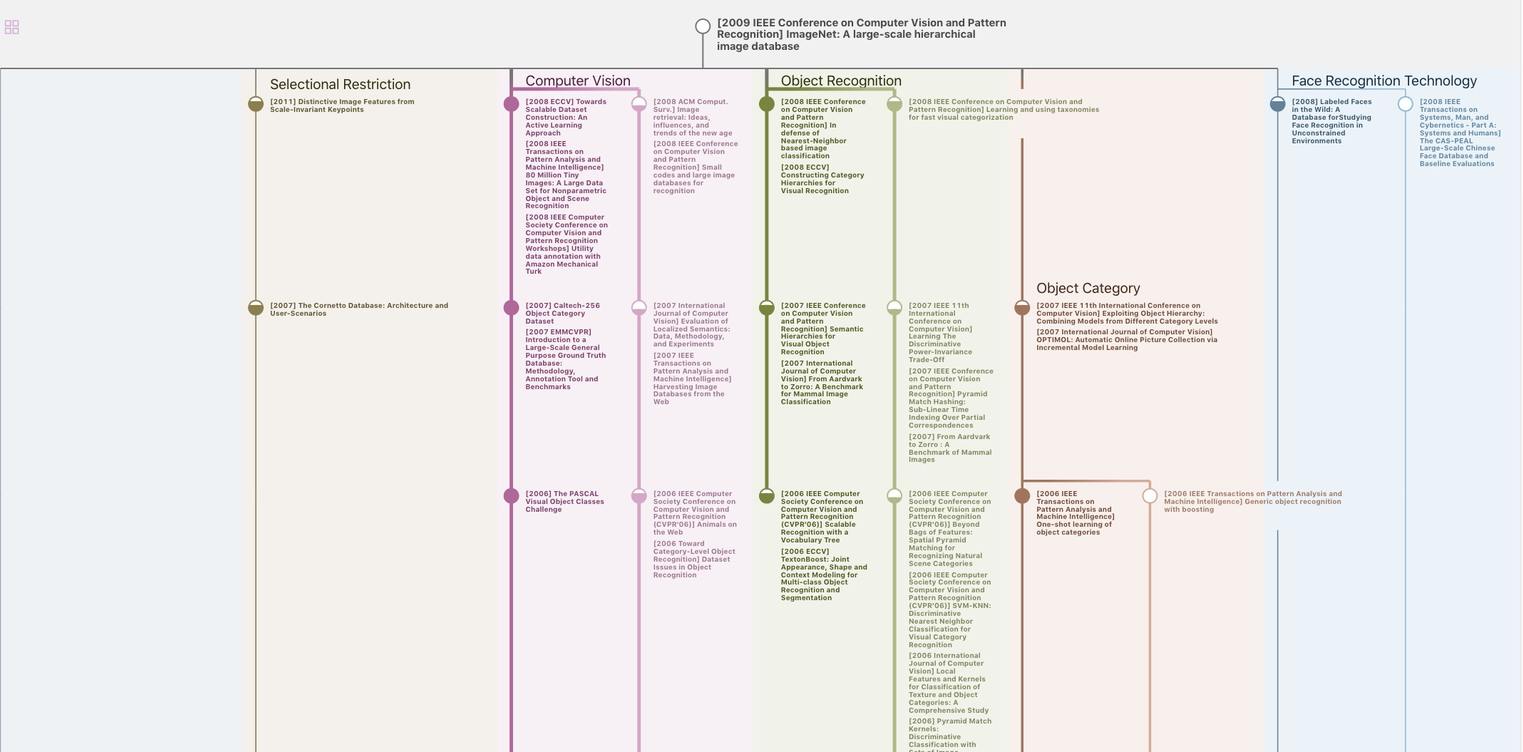
生成溯源树,研究论文发展脉络
Chat Paper
正在生成论文摘要