Through the Data Management Lens: Experimental Analysis and Evaluation of Fair Classification
PROCEEDINGS OF THE 2022 INTERNATIONAL CONFERENCE ON MANAGEMENT OF DATA (SIGMOD '22)(2022)
摘要
Classification, a heavily studied data-driven machine learning task, drives a large number of prediction systems involving critical decisions such as loan approval and criminal risk assessment. However, classifiers often demonstrate discriminatory behavior, especially when presented with biased data. Consequently, fairness in classification has emerged as a high-priority research area. Data management research is showing an increasing presence and interest in topics related to data and algorithmic fairness, including the topic of fair classification. The interdisciplinary efforts in fair classification, with machine learning research having the largest presence, have resulted in a large number of fairness notions and a wide range of approaches that have not been systematically evaluated and compared. In this paper, we contribute a broad analysis of 13 fair classification approaches and additional variants, over their correctness, fairness, efficiency, scalability, robustness to data errors, sensitivity to underlying ML model, data efficiency, and stability using a variety of metrics and real-world datasets. Our analysis highlights novel insights on the impact of different metrics and high-level approach characteristics on different aspects of performance. We also discuss general principles for choosing approaches suitable for different practical settings, and identify areas where data-management-centric solutions are likely to have the most impact.
更多查看译文
关键词
Empirical study, Algorithmic fairness, Classifiers
AI 理解论文
溯源树
样例
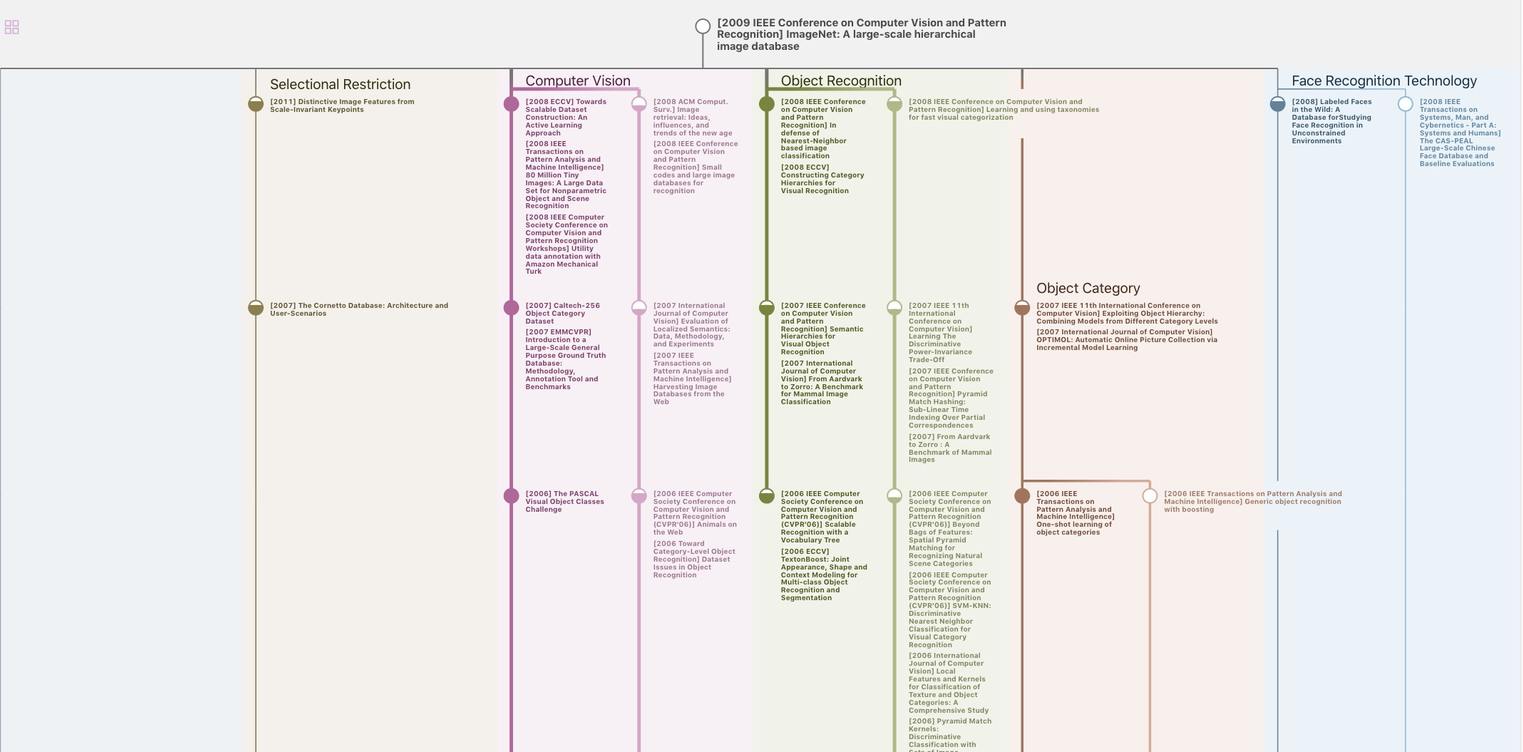
生成溯源树,研究论文发展脉络
Chat Paper
正在生成论文摘要